Mixup Based Cross-Consistency Training for Named Entity Recognition
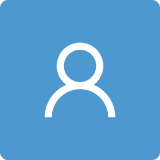
Round 1
Reviewer 1 Report
The article from a methodological point of view meets the expectations of a scientific article. The authors establish clear categories of analysis and comprehensively explain the procedure used deep learning models. The methodology is also very clear and well worked out from a practical point of view.
Minors
Lines 58-75 are for the contribution of this paper.
I had a little bit of curiosities as follows:
1. The method proposed in this paper proposes a method that can do NER better using weakly labeled data. There are existing studies on various deep learning approaches. As a result of searching for existing studies, I also found that, in practice, the most effective way to obtain better results by further training the existing model and improving the model itself. I think you have searched various studies. I am curious what made you focus on weakly labeled data other than improving the loss function of the existing model or improving the model itself.
2. In this paper, data from the biomedical domain and the tech domain were used in the experimental results (Lines 273-274). Another question I have is after checking the following funding information. (Lines 385-386). The English NER data set will be diverse, and it is thought that there will be many studies on the English data set. I wonder if there is an experimental plan for whether the framework proposed in this paper has potential even with the Korean NER dataset. Moreover, in general, the most commonly used data set for experiments is (1) CoNLL 2003 (Sang and Meulder, 2003) https://www.clips.uantwerpen.be/conll2003/ner/, (2)OntoNotes 5.0 (Weischedel) etal., 2013) https://catalog.ldc.upenn.edu/LDC2013T19, etc. However, this paper did not use such a general data set. I'm also curious as to what made you didn't use the generic data set.
Funding: This work was supported by the Technology development Program (S2957729) funded by 385
the Ministry of SMEs and Startups (MSS, Korea) 386
We show the effectiveness of the proposed method by the results of experiments on 273
the biomedical domain NER and tech domain NER. 274
Author Response
Response to Reviewer 1 Comments
We have revised the paper significantly based on the review comments and found that the paper is significantly enhanced so we thank the journal and reviews for their efforts in this regard. Below, we first repeat the reviewers’ comments and then note our response to that comment in red font.
Point 1: The method proposed in this paper proposes a method that can do NER better using weakly labeled data. There are existing studies on various deep learning approaches. As a result of searching for existing studies. I also found that, in practice, the most effective way to obtain better results by further training the existing model and improving the model itself. I think you have searched various studies. I am curious what made you focus on weakly labeled data other than improving the loss function of the existing model or improving the model itself.
Response 1: We thought that improving the loss function or the model itself is another direction of our study. This paper aims to alleviate human annotating efforts because the annotating NER dataset is expensive and time-consuming. That is, our study has focused on domain-specific NER tasks and therefore environments where there is insufficient data. With a full understanding of the purpose, we tried to utilize the generalized structure of the model and the loss function. At the same time, our proposed framework focuses on the utilization of additional resources. We explained the purpose of the paper in detail as below.
- (Page 6, line 199-201)
This paper aims to alleviate human annotating efforts. With a full understanding of the purpose, we tried to utilize the generalized structure of the model and the loss function.
Point 2: In this paper, data from the biomedical and tech domains were used in the experimental results (Lines 273-274). Another question I have is after checking the following funding information results (Lines 385-386). The English NER data set will be diverse, and it is thought that there will be many studies on the English data set. I wonder if there is an experimental plan for whether the framework proposed in this paper has potential even with the Korean NER dataset. Moreover, in general, the most commonly used data set for experiments is (1) CoNLL 2003 (Sang and Meulder, 2003), (2) OntoNotes 5.0 (Weischedel et al., 2013), etc. However, this paper did not use such a general data set. I’m also curious as to what made you didn’t use the generic data set.
Response 2: the purpose of the proposed framework in funding supported projects was to find specific domain entities within speech or documentation of specific domains rather than to find general entities such as a person, location, and organization. Further, as we mentioned in the paper, the proposed framework used the knowledge base to annotate weakly labeled data, especially domain-specific NER tasks. So, we focused on the domain-specific NER where there is an insufficient dataset. Also, as your comments, we wanted to conduct experiments on the Korean NER dataset, but there was no domain-specific Korean benchmark dataset such as the biomedical and tech domain.
Author Response File: Author Response.docx
Reviewer 2 Report
AttachedComments for author File: Comments.pdf
Author Response
Response to Reviewer 2 Comments
We have revised the paper significantly based on the review comments and found that the paper is significantly enhanced so we thank the journal and reviews for their efforts in this regard. Below, we first repeat the reviewers’ comments and then note our response to that comment in red font.
Point 1: My Recommendation is based on content and weighing of strengths verse weaknesses I therefore find that this article may be published without changes.
Response 1: We revised the paper according to your comments, and it further developed the paper. We added more details on the purpose of the paper as below.
- (Page 6, line 199-201)
This paper aims to alleviate human annotating efforts. With a full understanding of the purpose, we tried to utilize the generalized structure of the model and the loss function.
Author Response File: Author Response.docx