Learning Deep Representations of Cardiac Structures for 4D Cine MRI Image Segmentation through Semi-Supervised Learning
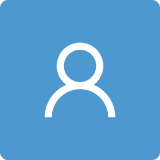
Round 1
Reviewer 1 Report
Review comments:
In this paper, the author proposes a semi supervised model of disentangled representation combined with various improved loss. On the Stacom 2017 ACDC video MRI dataset, only 1% of labeled data is used to achieve accurate segmentation and reconstruction of cardiac structure, which has certain reference significance for medical image segmentation that is difficult to label. The method of the article is innovative, and the results obtained have potential clinical research value. The article is written in standard English with clear logic, relatively sufficient experimental evidence and clear exposition. The theme of the article is in line with the subject coverage of the magazine and is attractive to some readers. It is recommended to accept the publication.
1. Please check whether Figure 2 (b) is correct. If it is correct, please add a description or make minor changes. At the same time, please carefully check other illustrations to avoid misleading the readers.
2. The article only compares its own experiments, and does not compare with existing research results and research results in related fields.
3. What networks are used for the segmentation generator and discriminator in this article? Please provide additional information.
4. How are the FiLM and SPADE modules spliced in this article? Is there a reconstruction network after splicing? Please provide additional information.
5. It is suggested that the author read the full text thoroughly, pay attention to the use of scientific research terminology, and express it strictly.
Author Response
A previous version of this manuscript was submitted for peer review – below is a summary of the major points raised by the reviewers, along with our response and how they were addressed in the revised manuscript:
Reviewer # 1: In this paper, the author proposes a semi supervised model of disentangled representation combined with various improved loss. On the Stacom 2017 ACDC video MRI dataset, only 1% of labeled data is used to achieve accurate segmentation and reconstruction of cardiac structure, which has certain reference significance for medical image segmentation that is difficult to label. The method of the article is innovative, and the results obtained have potential clinical research value. The article is written in standard English with clear logic, relatively sufficient experimental evidence and clear exposition. The theme of the article is in line with the subject coverage of the magazine and is attractive to some readers. It is recommended to accept the publication.
- Please check whether Figure 2 (b) is correct. If it is correct, please add a description or make minor changes. At the same time, please carefully check other illustrations to avoid misleading the readers.
- The article only compares its own experiments and does not compare with existing research results and research results in related fields.
- What networks are used for the segmentation generator and discriminator in this article? Please provide additional information.
- How are the FiLM and SPADE modules spliced in this article? Is there a reconstruction network after splicing? Please provide additional information.
- It is suggested that the author read the full text thoroughly, pay attention to the use of scientific research terminology, and express it strictly.
Authors’ Response:
We thank this reviewer for their constructive comments and suggestions, which have been addressed in the revision and have improved the overall presentation of the manuscript.
- We have corrected all the figures and clarified the presentation of the model.
- The main goal of this work is to demonstrate the ability to achieve a high segmentation accuracy, comparable to that of supervised techniques trained on large, annotated datasets, using only limited amounts of labeled data. Unfortunately, the availability of large amounts of well-annotated medical imaging datasets is limited, and, similarly, the availability of segmentation methods developed to perform optimally on limited annotated data is also quite scarce. As such, a fair and consistent comparison would entail the implementation of all methods on the same datasets; however, this is not feasible, as 1) similar methods are scarce, 2) any existing methods were evaluated on different datasets, and 3) are not available to implement on the current dataset. As such, to address this to the best of our availability under these circumstances, we resort to citing several previous works and their performance metrics (and state their conditions and test datasets used), while discussing our results in the contest of the results of these other methods. Please refer to page 25 in the revised manuscript for these edits.
- Our segmentation generator network consists of a few convolution layers followed by a batch normalization layer along with a Leaky-ReLU, except for the last layer. We use a structure similar to DCGAN for the discriminator network. We have provided additional detail on the architecture of the generator-discriminator network in Section 2.3.2 on page 12 of the revised manuscript.
- FiLM and SPADE are themselves reconstruction networks and work as decoders. These decoders generate an image by combining a spatial and a non-spatial representation. Two decoders are considered based on FiLM or SPADE conditioning. Finally, the output of the two decoders is re-entangled in order to reconstruct an image. We have added additional details about the architecture of the FiLM and SPADE decoders. These two decoders are added together to reconstruct the input image (Please see Section 2.1.4 on pages 9-10).
- We have thoroughly read and edited the manuscript to improve the overall presentation and facilitate the reader’s understanding of the disseminated work. These edits appear throughout the manuscript and unfortunately cannot be specifically identified via specific locations, but these edits are transparent throughout the revision.
Author Response File: Author Response.docx
Reviewer 2 Report
Journal: Applied Sciences (ISSN 2076-3417)
Manuscript ID: applsci-1984911
Type: Article
Title: Learning Deep Representations of Cardiac Structures for 4D Cine MRI Image Segmentation through Semi-supervised Learning.
Authors: S. M. Kamrul Hasan*, Cristian A. Linte.
a) Introduction: add more recent refs from the literature survey? And write the objective of the present work carefully.
b) For references, choose recent refs. Please, refer to these refs. are very useful for the different measurement characterization
DOI: https://doi.org/10.1088/1742-6596/1879/2/022118
DOI: https://doi.org/10.1088/1742-6596/1999/1/012080
Best Regards
Author Response
A previous version of this manuscript was submitted for peer review – below is a summary of the major points raised by the reviewers, along with our response and how they were addressed in the revised manuscript:
Reviewer #2:
- a) Introduction: add more recent refs from the literature survey? And write the objective of the present work carefully.
- b) For references, choose recent refs. Please, refer to these refs. are very useful for the different measurement characterization
Authors’ edits:
We thank this reviewer for their constructive comments and suggestions, which have been addressed in the revision and have improved the overall presentation of the manuscript.
We have rewritten the introduction section to improve clarity and better state the premise of the proposed work, emphasize its motivation, and contributions to this research, and also included additional references. Please refer to the edited abstract and introduction section in the revised manuscript.
Author Response File: Author Response.docx
Reviewer 3 Report
The paper presents he application of semi-supervised learning in the context of cardiac structures. The following comments can help improve the paper:
1)The abstract is quite long and it is hard to follow the motivation, problem statement, and contributions. It would be good to trim the abstract to keep the focus on key elements of the work.
2) The structure of the introduction could be improved. The problem statement should be made clear. The gaps in research should also be made clear. The contributions should be stated in the context of prior work and how this paper improves upon the state of the art.
3) A roadmap of the paper is missing in the introduction.
4) A dedicated related work section is missing. It would be good to summarize the key papers on the topic. The summary could be presented in the form of a table and you could distinguish how your work is different from these prior works.
5) The related work should be comprehensive. Recent papers related to machine learning and object detection should be studied and included in the related work. Few examples are as follows: DOI: 10.1145/3544491 and 10.1109/JBHI.2021.3094311
6) The methodology section should have a table summarizing the key parameters and tools used.
7) Please include a table to summarize the ACDC dataset.
8) Please summarize the performance metrics and their definitions.
9) There are several results. It would be good to emphasize or highlight the takeaways from the results section.
10) The highlights of the paper should be emphasized in the introduction.
Author Response
A previous version of this manuscript was submitted for peer review – below is a summary of the major points raised by the reviewers, along with our response and how they were addressed in the revised manuscript:
Reviewer #3: The paper presents he application of semi-supervised learning in the context of cardiac structures. The following comments can help improve the paper:
1)The abstract is quite long and it is hard to follow the motivation, problem statement, and contributions. It would be good to trim the abstract to keep the focus on key elements of the work.
2) The structure of the introduction could be improved. The problem statement should be made clear. The gaps in research should also be made clear. The contributions should be stated in the context of prior work and how this paper improves upon the state of the art.
3) A roadmap of the paper is missing in the introduction.
4) A dedicated related work section is missing. It would be good to summarize the key papers on the topic. The summary could be presented in the form of a table, and you could distinguish how your work is different from these prior works.
5) The related work should be comprehensive. Recent papers related to machine learning and object detection should be studied and included in the related work. Few examples are as follows: DOI: 10.1145/3544491 and 10.1109/JBHI.2021.3094311
6) The methodology section should have a table summarizing the key parameters and tools used.
7) Please include a table to summarize the ACDC dataset.
8) Please summarize the performance metrics and their definitions.
9) There are several results. It would be good to emphasize or highlight the takeaways from the results section.
10) The highlights of the paper should be emphasized in the introduction.
Authors’ Response:
We thank this reviewer for their constructive comments and suggestions, which have been addressed in the revision and have improved the overall presentation of the manuscript.
- We have written the abstract and clarified our motivation, problem statement, as well as contributions. Please refer to the Abstract of the revised manuscript.
- We have rewritten the introduction section and clarified the problem statement, as well as the research gaps and contributions. Please refer to the Introduction section of the revised manuscript.
- We have added the roadmap of the paper at the end of the Introduction section in the revised manuscript.
- In the original version, the related work was integrated as part of the Introduction section, along with the problem statement, challenges, ongoing efforts, and their limitations, and proposed work. Nevertheless, as suggested by this reviewer, we have restructured the Introduction of the revised manuscript and labeled a sub-section of it as Related Works.
- These references suggested by this reviewer have been cited in the Related Works section in the revised manuscript.
- Key parameters have been summarized as part of the text in the methods section. Please refer to pages 5 - 7.
- For the sake of conciseness, the ACDC dataset was described briefly in a short paragraph (please refer to page 12), with additional citations pointing to publications specifically describing the dataset in greater detail for those interested.
- The definitions and necessary details pertaining to the evaluation metrics were included in the revised manuscript. Please refer to Section 2.4.
- A summary of the most relevant findings has been included in the Results/Discussion section. In addition, we also conducted an evaluation of the effect of different components of our overall model by removing different major components (e.g., disentanglement part, reconstruction part, etc.) and assessing their effect on the overall segmentation performance, as summarized in Table 1 – 4 in the Results section of the revised manuscript.
- As previously stated in our response to Comment #2, the contributions of this research have been better emphasized in both the Introduction (and Conclusion) of the revised manuscript.
Author Response File: Author Response.docx
Reviewer 4 Report
This study hypothesizes that your proposed model has the potential to become an efficient semantic segmentation tool that may be used for domain adaptation in data-limited medical imaging scenarios where annotations are expensive. However, there are many serious problems in this study.
1. Is this study a review? Or research? In a research study, what was the control group?
2. Is there a comparison between humans and AI or a comparative analysis of various AI technologies in this study?
3. Can this study make it clearer in the selection of regions of interest?
4. Is there a consistent analysis of the results? How to ensure the repeatability of the study?
Author Response
A previous version of this manuscript was submitted for peer review – below is a summary of the major points raised by the reviewers, along with our response and how they were addressed in the revised manuscript:
Reviewer #4: This study hypothesizes that your proposed model has the potential to become an efficient semantic segmentation tool that may be used for domain adaptation in data-limited medical imaging scenarios where annotations are expensive. However, there are many serious problems in this study.
- Is this study a review? Or research? In a research study, what was the control group?
- Is there a comparison between humans and AI or a comparative analysis of various AI technologies in this study?
- Can this study make it clearer in the selection of regions of interest?
- Is there a consistent analysis of the results? How to ensure the repeatability of the study?
Authors’ Response:
We thank this reviewer for their constructive comments and suggestions, which have been addressed in the revision and have improved the overall presentation of the manuscript.
- The manuscript focuses on the development, implementation and evaluation of a new deep learning-based technique for cardiac image segmentation. Moreover, the submitted work is best described as applied research, as the developed and validated methods are targeted toward improving the performance of cardiac image segmentation with minimal reliance on expert-annotated cardiac images for training. While the work makes several contributions to new methodologies/algorithms, the primary contribution is to the intended application field, specifically cardiac image segmentation. As such, we compared our proposed algorithm to traditional fully supervised methods trained on large, annotated datasets, but we also compared the performance of our method and explored its “breaking point” by increasingly reducing the extent of annotated data used for training while maintaining the application-expected performance. As such, the baseline methods consist of A) traditional fully supervised, fully trained methods using ample annotated data and B) a proposed algorithm “tuned” to use various reduced extents of annotated data.
- The performance of our algorithm is assessed against the ground truth segmentation data and the clinical parameters are quantified based on the ground truth data, which represents human performance, as the ground truth data was annotated by expert clinicians. Moreover, we also assessed the proposed method against other AI-based algorithms, as iterated in the response to comment # 1, as well as to several variations of the AI-based algorithm trained using increasingly lower amounts of annotated data.
- This manuscript presents our work on a semi-supervised learning framework for 4D cine cardiac MRI image segmentation that enables the learning of deep cardiac structures while relying on minimal expert annotated data for training. Our proposed method builds on several recent and key research findings in the fields of generative models, semi-supervised learning, and representation learning via disentanglement. As part of the data pre-processing, the region (or rather a volume) of interest is automatically identified from the thoracic/cardiac MRI images by cropping out a sub-volume of the image that contains the heart.
- Since this work proposes an automated, AI-based technique for cardiac image segmentation, the results are consistent each time the algorithm is run on the same datasets, so unlike human performance, which is highly non-repeatable and non-reproducible due to inherent intra- and inter-rater variability, automated methods yield consistent, repeatable results provided same, consistent input. Moreover, to further evaluate the robustness of the proposed method, we trained the proposed AI-based method on different datasets, and while the segmentation results are slightly different in response to the training data used, the variance associated with the automated AI-based segmentation results is much lower than the variance associated with human performance. Hence, we believe the proposed framework’s reliance on as little as 1% labeled data for training, in concert with the high segmentation accuracy achieved, comparable to the fully or semi-supervised models, renders the proposed work an attractive solution for medical image segmentation, where access to vast expert-annotated data is expensive and often difficult to gain access to.
Author Response File: Author Response.docx
Round 2
Reviewer 3 Report
Thanks for addressing my comments. Please note that 10.1145/3544491 has not been referenced as you mentioned in your response. Apart from proof-read I have no further comments.
Reviewer 4 Report
The author's answers to the four questions are more like sophistry than academic reply.
For example, the fourth question: the most common consistency analysis is the intraclass correlation coefficient.