Using AI Motion Capture Systems to Capture Race Walking Technology at a Race Scene: A Comparative Experiment
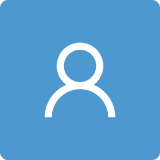
Round 1
Reviewer 1 Report
The research subject is essential, and it was clearly explained. The main advantage of this analysis system is its availability during competitions or exercises, but unfortunately, these issues are not well described.
1. Please explain in more detail the analysis and the equipment used for the study, including the mathematical analysis used.
2. Please explain how long the motion was captured? Did you take motion capture of the 20 km? Did you choose single moments or seconds? It is essential to explain all the methods that you used during the study.
3. I think the author must add a description of the athletes, age, height, etc.
4. There is only a Table of the correlation, but I think we need to know also the motion parameters, such as the angles of the joints, the velocity of movement, etc.
Author Response
1. Please explain in more detail the analysis and the equipment used for the study, including the mathematical analysis used.
We added more information about the operation of the software in the research method section of the manuscript.
2. Please explain how long the motion was captured? Did you take motion capture of the 20 km? Did you choose single moments or seconds? It is essential to explain all the methods that you used during the study.
Set the shooting frequency of the camera to 60 fps and the shutter speed to 1/1200 s. The camera is turned on when the athlete is about to enter the calibration space, and turned off when the athlete leaves the calibration space.
The competition field of adult women's 20km walking race is 1km/lap, and the athletes should walk 20 laps on the competition field. We filmed every lap of walking race of all athletes. By calculating the average speed of athletes in each lap, we found that the speed of almost all athletes in laps 6, 7 and 8 was very close to the average speed of the whole journey, and the athletes were very energetic in laps 6, 7 and 8, and were rarely interfered by other athletes. So we chose the competition video of athletes in laps 6, 7 and 8 for analysis.
3. I think the author must add a description of the athletes, age, height, etc.
In the subject part of the manuscript, we added the age, height and other information of the subject.
4. There is only a Table of the correlation, but I think we need to know also the motion parameters, such as the angles of the joints, the velocity of movement, etc.
In the result part of the manuscript, we added the comparison of kinematics parameters of athletes' walking race.
Reviewer 2 Report
The AI motion capture system can quickly and accurately obtain the 3D coordinate data of human joints in outdoor sports scenes, and provide the biomechanical parameters and effect indicators of athletes. It is verified that the 3D coordinates of human joints obtained by the AI motion capture system are highly similar to the average value of the 3D coordinates obtained by manual analysis, and the walking technique motion capture at the walking competition scene has high reliability. The system solves the problem that under the non laboratory conditions, the athletes can also obtain the kinematic data of the race walkers without pasting reflective points on them. In general, this paper is well written and easy to follow. I would like to accept this paper if my following concerns are carefully addressed.
(1) The authors need to emphasise their contributions/novelties in the revision. In the current version, the authors did not discuss their contributions in detail.(2) The proposed algorithm still can be improved if the ideas in the following papers are explored, i.e., "Making Sense of Spatio-Temporal Preserving Representations for EEG-Based Human Intention Recognition", "An Adaptive Semisupervised Feature Analysis for Video Semantic Recognition", and "A Semisupervised Recurrent Convolutional Attention Model for Human Activity Recognition". The authors are encouraged to discuss them in the revision.
(3) The authors should carefully proofread this paper and correct all the typos in the revision. In the current version, there are still some typos/grammar errors.
(4) Could the authors report the running time of the proposed algorithm? In this way, we can justify whether this algorithm can be applied to large-scale dataset.
Based on the above comments, I would like to accept this paper with minor revision.
Author Response
(1) The authors need to emphasise their contributions/novelties in the revision. In the current version, the authors did not discuss their contributions in detail.
We added and emphasized the author's contribution and innovation in the manuscript.
(2) The proposed algorithm still can be improved if the ideas in the following papers are explored, i.e., "Making Sense of Spatio-Temporal Preserving Representations for EEG-Based Human Intention Recognition", "An Adaptive Semisupervised Feature Analysis for Video Semantic Recognition", and "A Semisupervised Recurrent Convolutional Attention Model for Human Activity Recognition". The authors are encouraged to discuss them in the revision.
In the discussion part of the manuscript, we supplement and discuss the algorithm of the artificial intelligence system.
(3) The authors should carefully proofread this paper and correct all the typos in the revision. In the current version, there are still some typos/grammar errors.
we edited the revised manuscript in English in the company designated by MDPI. https://www.mdpi.com/authors/english
(4) Could the authors report the running time of the proposed algorithm? In this way, we can justify whether this algorithm can be applied to large-scale dataset.
We are very sorry that China Dalian Ruidong Technology Co., Ltd. only disclosed to the public which algorithms are used in the AI motion capture system. The specific details of the algorithms were not disclosed to the public.