A Traffic Crash Warning Model for BOT E-Tolling Operations Based on Predictions Using a Data Association Framework
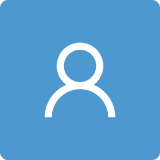
Round 1
Reviewer 1 Report
The paper is interesting. According to the title of the paper, an sociation method and framework combined with deep learning is proposed to construct a crash prediction and warning model for highways (based on data from in Taiwan national highway system). This is an important issue from the point of view of traffic safety.
Looking at the profile of the "Applied Sciences" journal, I can say that the proposed article fits with that profile.
The structure of the article is clear. A review of knowledge is presented, followed by formulation of the research problem. Then, the research method and models are presented. An important point is the presentation of the method testing and validation results. The article is summarized with conclusions in which the main achievements were noted. Directions for further work, resulting directly from the experience of testing the presented method, were also indicated.
I have one general comment regarding improving the quality and readability of the article. Please improve the quality of the drawings. They should be enlarged (including increasing the resolution) to make the labels on them legible. In the case of Fig. 1 - a comment is recommended that better describes its meaning, which will facilitate its interpretation. There are also symbols not explained in the text.
Author Response
Please see the attachment.
Author Response File: Author Response.docx
Reviewer 2 Report
1. In Introduction, the authors are recommended to provide more details on the research gaps to resolve.
2. The significance and contributions shall better be emphasized.
3. Since the authors mentioned applications of Bayesian Network in studying highway traffic. The relevant studies shall better be reviewed. e.g., Optimized structure learning of Bayesian Network for investigating causation of vehicles’ on-road crashes. Reliability Engineering & System Safety, 224, 108527.
4. Also, the authors are recommend to compare the proposed deep learning method with other state-of-the-art ML/DL or statistical methods and explain the pros/cons of the proposed method.
5. What are the possible applications of the proposed method in real world?
N/A
Author Response
Please see the attachment.
Author Response File: Author Response.docx
Reviewer 3 Report
Review
of the article “A Traffic Crash Warning Model for BOT e-Tolling Operations Based on Predictions Using a Data Association Framework”
This work is original, dedicated to the solution of the current issues of forecasting and prevention of traffic accidents, considering the dynamics of traffic parameters. Comparison of different approaches to modeling, consideration of several input data filtering criteria, use of modern training algorithms of the proposed model testify to the scientific importance of this study. The high accuracy of the simulated values emphasizes the practical significance of the work. However, there are some inaccuracies in the text of the article. The article can be accepted for publication after correction in accordance with the following comments and recommendations.
1) The authors state that the traffic volume results were converted into passenger car equivalents in accordance with the road capacity manual published by the Taiwanese government. But the reference to this source is not given. The applied gear ratios are of interest. It would be better to present them in the work.
2) The title of Figure 1 needs to be clarified.
3) It is not entirely clear how the average travel time was determined (Line 179). It is noted that the duration of travel through two neighboring gantries was summed up every 5 minutes. What values can the subscript t take in the designation of terms? Why is this index introduced?
4) Lines 192-193 indicate that changes in traffic can significantly reduce vehicle speed, potentially leading to traffic accidents. If changes in traffic volume are the primary cause, why is the relationship between speed difference (rather than intensity difference) and the occurrence of traffic accidents investigated?
5) Criterion 3 for data filtering (page 5) needs a more detailed explanation. It is necessary to specify the maximum allowed speed of movement on the investigated highway.
6) Some of the blocks in Figure 2 and Figure 5 are too small, so they should be enlarged.
7) For a better understanding, the axes on the graphs in Figure 3 should be signed.
8) It is not clear from the text how the process of early warning of road users regarding traffic accidents will be ensured.
9) Needs an explanation of the repetition of blocks regarding Current gantry area data at the bottom of Figure 5.
10) It would be better to supplement the article with tabular values of input data, actual and predicted values of resulting parameters.
11) The model outputs are binary solutions, but this does not explain the choice of sigmoidal activation function. Please provide a more comprehensive explanation.
Author Response
Please see the attachment.
Author Response File: Author Response.docx
Reviewer 4 Report
Although the nature of the analyzed highway becomes evident as the reader advances in the lecture, it is recommended to explicitly add a diagram (or table of characteristics) of the highway system. Namely, how many lanes does it contain? Is there a contention wall between the upstream and downstream lanes?
Since Figure 5 is central to this paper, improving its quality and aesthetics is suggested.
It needs to be clarified if the Accident Labeling Dataset described in Section 3.2 is the same one mentioned in Section 3. In Section 3.3, the authors state that traffic accident records come from insufficient headway and inattentional blindness (is that another dataset?). The authors should be more specific on the origin of the traffic accidents dataset. Besides, the quality of Figure 1 should be improved.
There are typos in lines 119, 120, 187, and 193.
The quality of Figure 2 should be improved.
Although the model's accuracy is around 94%, its precision is less than 10%. The impact of such a low precision value should be exposed. For instance, does this implies that too many unneeded alarms might be sent to the drivers? If too many alarms are sent, do drivers lose confidence in them?
Author Response
Please see the attachment.
Author Response File: Author Response.docx