Dynamic Graph Convolutional Crowd Flow Prediction Model Based on Residual Network Structure
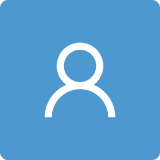
Round 1
Reviewer 1 Report
The paper adequately describes the architecture of the proposed model. However, providing more detailed information about the specific layers, their configurations, and the reasoning behind their choices would enhance the understanding of the model's design. Why has the author preferred the Huber loss 18function? The paper should provide a more comprehensive explanation of the dynamic graph construction process, including details on how the spatial and temporal relationships are captured and updated dynamically. It would be valuable to include a discussion on the computational complexity of the proposed model, particularly considering the integration of the residual network structure. This information would help assess the feasibility of deploying the model in real-world applications. Some editing for the English language is required throughout the manuscript. Provide source image citation in the figure 4. The paper should discuss any limitations of the proposed model and potential directions for future research. This would provide insights into areas that could be further explored and improved upon. Refer the latest and suitable references as follows: Jing-Yu, Chen, and Wang Ya-Jun. "Semi-Supervised fake reviews detection based on AspamGAN." Journal of Artificial Intelligence 4, no. 1 (2022): 17-36. Sushith, Mishmala. "Semantic Feature Extraction and Deep Convolutional Neural Network-based Face Sentimental Analysis." JIIP 4, no. 3 (2022): 157-164. The paper adequately describes the architecture of the proposed model. However, providing more detailed information about the specific layers, their configurations, and the reasoning behind their choices would enhance the understanding of the model's design. Why has the author preferred the Huber loss 18function? The paper should provide a more comprehensive explanation of the dynamic graph construction process, including details on how the spatial and temporal relationships are captured and updated dynamically. It would be valuable to include a discussion on the computational complexity of the proposed model, particularly considering the integration of the residual network structure. This information would help assess the feasibility of deploying the model in real-world applications. Some editing for the English language is required throughout the manuscript. Provide source image citation in the figure 4. The paper should discuss any limitations of the proposed model and potential directions for future research. This would provide insights into areas that could be further explored and improved upon. Refer the latest and suitable references as follows: Jing-Yu, Chen, and Wang Ya-Jun. "Semi-Supervised fake reviews detection based on AspamGAN." Journal of Artificial Intelligence 4, no. 1 (2022): 17-36. Sushith, Mishmala. "Semantic Feature Extraction and Deep Convolutional Neural Network-based Face Sentimental Analysis." JIIP 4, no. 3 (2022): 157-164.Author Response
Dear Editors and Reviewers:
Thanks for your letter and the reviewers' constructive comments concerning our manuscript entitled ' Dynamic Graph Convolutional Crowd Flow Prediction Model Based on Residual Network Structure ' (ID: applsci-2437777). Those comments are all valuable and very helpful for revising and improving our manuscript, and significantly guide our research. We have studied these comments carefully and made modifications which we hope meet with your approval. Revised portions are marked red in the manuscript. The main corrections in the manuscript and the responses to the reviewers' comments are listed below.
Point 1: Providing more detailed information about the specific layers, their configurations, and the reasoning behind their choices would enhance the understanding of the model's design.
Response 1: Thanks very much for your comments, which are very helpful to improve the quality of this article. We have made extensive changes in the second paragraph of 3.3, the entire section of 3.4, and the second paragraph of 3.5 to provide a more comprehensive explanation of the specific layers' configurations and choices, explaining the motivation as well as the purpose of the model improvements.
Point 2: Why has the author preferred the Huber loss 18function?
Response 2: As suggested by the valuable comment, We provide an explanation in the second paragraph of Section 3.5. The Huber function was used because a large number of outliers were found in the dataset, and the Huber function can handle this problem well. Regarding the detection of outliers, in Figure 5 and Figure 6 in Section 4.1.
Point 3: The paper should provide a more comprehensive explanation of the dynamic graph construction process, including details on how the spatial and temporal relationships are captured and updated dynamically.
Response 3: As suggested by the valuable comment, We have added an explanation of the dynamic graph construction process in Section 3.2. And about the capture of spatio-temporal correlation, which is implemented by dynamic graph convolution, spatio-temporal attention module and conditional convolution module, we have explained in more details in the second paragraph of Section 3.3 and the first paragraph of Section 3.4. Finally, about the implementation of dynamic updates, in the region segmentation training task in the third paragraph of Section 3.5.
Point 4: It would be valuable to include a discussion on the computational complexity of the proposed model, particularly considering the integration of the residual network structure. This information would help assess the feasibility of deploying the model in real-world applications.
Response 4: Thanks very much for your comments, which are very helpful to improve the quality of this article. We conducted a comparative experiment on model training time and the number of parameters in Section 4.3, analyzing the feasibility of Res-DGCN in the project task. The results indicate that Res-DGCN is feasible for real-world project tasks.
Point 5: Some editing for the English language is required throughout the manuscript.
Response 5: We tried our best to improve the manuscript and made some changes to the manuscript. These changes will not influence the content and framework of the paper. And here we did not list the changes but marked in red in the revised paper. We appreciate for Reviewers' warm work earnestly and hope that the correction will meet with approval.
Point 6: Provide source image citation in the figure 4.
Response 6: We are very glad to receive your comments. But Figure 4 is our original, no source image citation.
Point 7: The paper should discuss any limitations of the proposed model and potential directions for future research. This would provide insights into areas that could be further explored and improved upon.
Response 7: As suggested by the valuable comment, I have added a description of the limitations of our model and future work to the conclusion of Chapter 5.
Author Response File: Author Response.docx
Reviewer 2 Report
The paper discusses a residual structure-based dynamic graph convolutional network (Res-DGCN) developed by the authors for predicting population inflows and outflows in irregular urban areas. Convolution in the time dimension to effectively capture spatial and temporal correlations in population flow data The experiment was conducted on two real datasets, and the results show that the proposed model performs better than the baseline models.
In the article, a comparative analysis of various models, but does not indicate a comparison of the timing of their implementation for the project task.
In connection with some changes in crowd behavior associated with post-epidemic changes in society, also it is desirable to test the effectiveness of the model on modern data sets.
The text of the article needs editing
Author Response
Response to Reviewer 2 Comments
Dear Editors and Reviewers:
Thanks for your letter and the reviewers' constructive comments concerning our manuscript entitled ' Dynamic Graph Convolutional Crowd Flow Prediction Model Based on Residual Network Structure ' (ID: applsci-2437777). Those comments are all valuable and very helpful for revising and improving our manuscript, and significantly guide our research. We have studied these comments carefully and made modifications which we hope meet with your approval. Revised portions are marked red in the manuscript. The main corrections in the manuscript and the responses to the reviewers' comments are listed below.
Point 1: In the article, a comparative analysis of various models, but does not indicate a comparison of the timing of their implementation for the project task.
Response 1: Thanks very much for your comments, which are very helpful to improve the quality of this article. We conducted a comparative experiment on model training time and parameter count in Section 4.3, analyzing the feasibility of Res-DGCN in the project task. The results indicate that Res-DGCN is feasible for real-world project tasks.
Point 2: In connection with some changes in crowd behavior associated with post-epidemic changes in society, also it is desirable to test the effectiveness of the model on modern data sets.
Response 2: We agree that more studies would help demonstrate the validity of the model. At this point, we do not have the necessary tools to collect data that match the changing patterns of post-epidemic crowd movements. Second, the BikeNYC and TaxiNYC datasets used in this paper are very consistent with modern population behavior, and they are relatively new datasets. This was verified in the Multi-View Graph Convolutional Networks proposed by Sun and Zhang et al. in a previous study. We hope that in future work, the effectiveness of Res-DGCN will be demonstrated in more datasets that satisfy post-epidemic crowd flow patterns.
Author Response File: Author Response.docx
Reviewer 3 Report
In the manuscript, in order to realize accurate crowd flow prediction is essential for traffic guidance and traffic control, based on the original model 3D-DGCN, the authors proposed a residual structure-based dynamic graph convolution model (Res-DGCN).
Compared with the original model, the main creations in model architecture are:
1. A new spatial attention module (SA) to obtain the dependencies between the current node and its indirect neighbors. Traditional GCN cannot capture the relationship between indirect nodes, while the proposed spatial attention module is able to capture the spatial dependence of second-order and higher neighboring nodes by learning the spatial correlations in the dynamic spatio-temporal graph.
2. An improved conditional convolution module (SCondConv), which can perform deep convolution to extract noise-uncorrelated features with high efficiency. The main difference is the replacement of ReLU activation function with the Swish activation function, resulting in a new conditional convolution module.
In terms of model training, the main creation is in the loss function. Huber loss function is utilized in this study to replace the Mean Squared Error (MSE) loss function employed in the original model for its strong robustness, being continuously differentiable, and parameter control.
The model is trained and tested on 2 real-world datasets (TaxiNYC and BikeNYC) and compared with several models as the baseline. The overall performance is promising, although the improvement over 3D-DGCN is not significant.
Although the innovation shown in the manuscript is relatively limited to me, the study itself shows a high degree of completion, considering the technical details, experiments, and analysis included. I believe it deserves to be published in the journal after a minor revision.
Questions and suggestions:
1. Some of the content shares high similarities with the paper of 3D-DGCN. The authors should make it clear which part of the model (and the mathematical expression) is inherited from the original model without any change.
2. It seems there’s an unfinished line in Figure 2a.
Author Response
Response to Reviewer 3 Comments
Dear Reviewer:
Thanks for your letter and the reviewers' constructive comments concerning our manuscript entitled ' Dynamic Graph Convolutional Crowd Flow Prediction Model Based on Residual Network Structure ' (ID: applsci-2437777). Those comments are all valuable and very helpful for revising and improving our manuscript, and significantly guide our research. We have studied these comments carefully and made modifications which we hope meet with your approval. Revised portions are marked red in the manuscript. The main corrections in the manuscript and the responses to the reviewers' comments are listed below.
Point 1: Some of the content shares high similarities with the paper of 3D-DGCN. The authors should make it clear which part of the model (and the mathematical expression) is inherited from the original model without any change.
Response 1: Thanks very much for your comments, which are very helpful to improve the quality of this article. We add a description of the innovation points of this paper in the last paragraph in Section 3.2, as shown in the black dashed box in Figure 2. It can be divided into three main parts, the spatial attention module(SA), the improved conditional convolution module(SCondConv), and the loss function.
Point 2: It seems there’s an unfinished line in Figure 2a.
Response 2: We apologize for the oversight in Figure 2, and we have removed the excess lines.
Author Response File: Author Response.docx
Reviewer 4 Report
Dear Editor of Applied Science
The manuscript entitled "Dynamic graph convolutional crowd flow prediction model based on residual network structure” is an interesting study, which proposes a novel Dynamic Graph Convolutional Network (Res-DGCN) based on residual structures for predicting the inflow and outflow of populations in irregular urban areas. The research gap as well as the innovation of the research is properly represented. However, in my opinion, the manuscript has some shortcomings in the text. According to the mentioned items, I recommend a minor revision of the manuscript.
Best Regards
Comments for authors
1. More results should be provided in the abstract section.
2. Authors should use a third party to write the text. It is better not to use words like "we" (e.g., in the abstract section as well as in other sections).
3. What is “4.8%9.4%” in the abstract section?
4. The introduction section should be improved representing the literature review of the research background.
5. The caption of Figure 1 is too long. It should be concise. Just describe Figure 1 briefly in the caption, and present the explanations in the main text.
6. The heading of section 3 should be presented on the next page (page 5).
7. More conclusions should be presented in the conclusion section.
8. Abbreviations should be fully defined on the first use. For example, what are HA and ARIMA in section 2? It should be addressed.
9. Correct the heading of section 2 to “Methodology”.
10. The authors should present more future directions in the conclusion section. They can recommend other statistical analyses, modelling, and optimization algorithms to be incorporated into the proposed approaches: dx.doi.org/10.6007/IJARBSS/v4-i7/1002, doi.org/10.1109/TIA.2020.3010899, doi.org/10.1007/s10479-021-04003-5,
11. Keep the distance between the tables and figures and texts before or after it (e.g., before presenting Figures 1 and 4).
12. Language revision throughout the manuscript is needed to remove grammatical errors and make the language more technical.
13. Enrich the quality of the figures in this research (e.g., Figure 3).
14. What is the limitation of this research? It can be presented at the end of the conclusion section.
It is better not to use words like "we" (e.g., in the abstract section as well as in other sections).
Author Response
Response to Reviewer 4 Comments
Dear Reviewer:
Thanks for your letter and the reviewers' constructive comments concerning our manuscript entitled ' Dynamic Graph Convolutional Crowd Flow Prediction Model Based on Residual Network Structure ' (ID: applsci-2437777). Those comments are all valuable and very helpful for revising and improving our manuscript, and significantly guide our research. We have studied these comments carefully and made modifications which we hope meet with your approval. Revised portions are marked red in the manuscript. The main corrections in the manuscript and the responses to the reviewers' comments are listed below.
Point 1: More results should be provided in the abstract section.
Response 1: Thanks very much for your comments, which are very helpful to improve the quality of this article. We show more experimental results in the abstract section.
Point 2: Authors should use a third party to write the text. It is better not to use words like "we" (e.g., in the abstract section as well as in other sections).
Response 2: Thanks very much for your comments, which are very helpful to improve the quality of this article. We have made correction according to your comments.
Point 3: What is “4.8%9.4%” in the abstract section?
Response 3: We are very sorry for our incorrect writing. The model in this paper evaluates the performance by two metrics, mean absolute error MAE and mean square error RMSE, which correspond to two metrics respectively. We have revised the abstract section and thank you for your corrections.
Point 4: The introduction section should be improved representing the literature review of the research background.
Response 4: Thanks very much for your comments, which are very helpful to improve the quality of this article. We have moved the original section 2.1 to the introduction, which will make the article more structured.
Point 5: The caption of Figure 1 is too long. It should be concise. Just describe Figure 1 briefly in the caption, and present the explanations in the main text.
Response 5: Thanks very much for your comments, which are very helpful to improve the quality of this article. We have made correction according to your comments.
Point 6: The heading of section 3 should be presented on the next page (page 5).
Response 6: Thanks very much for your comments, which are very helpful to improve the quality of this article. We have made correction according to your comments.
Point 7: More conclusions should be presented in the conclusion section.
Response 7: As suggested by the valuable comment, I have added a description of the limitations of our model and future work to the conclusion of Chapter 5.
Point 8: Abbreviations should be fully defined on the first use. For example, what are HA and ARIMA in section 2? It should be addressed.
Response 8: We are very sorry for our incorrect writing, and we have revised the entire manuscript. HA is a historical average model and ARIMA is an autoregressive integrated moving average model.
Point 9: Correct the heading of section 2 to “Methodology”.
Response 9: Thanks very much for your comments, which are very helpful to improve the quality of this article. We have made correction according to your comments.
Point 10: The authors should present more future directions in the conclusion section. They can recommend other statistical analyses, modelling, and optimization algorithms to be incorporated into the proposed approaches: dx.doi.org/10.6007/IJARBSS/v4-i7/1002, doi.org/10.1109/TIA.2020.3010899, doi.org/10.1007/s10479-021-04003-5,
Response 10: As suggested by the valuable comment, I have added a description of the limitations of our model and future work to the conclusion of Chapter 5.
Point 11: Keep the distance between the tables and figures and texts before or after it (e.g., before presenting Figures 1 and 4).
Response 11: Thanks very much for your comments, which are very helpful to improve the quality of this article. We have made correction according to your comments.
Point 12: Language revision throughout the manuscript is needed to remove grammatical errors and make the language more technical.
Response 12: We tried our best to improve the manuscript and made some changes to the manuscript. These changes will not influence the content and framework of the paper. And here we did not list the changes but marked in red in the revised paper. We appreciate for Reviewers’ warm work earnestly and hope that the correction will meet with approval.
Point 13: Enrich the quality of the figures in this research (e.g., Figure 3)
Response 13: Thanks very much for your comments, which are very helpful to improve the quality of this article. We have made correction according to your comments.
Point 14: What is the limitation of this research? It can be presented at the end of the conclusion section.
Response 14: As suggested by the valuable comment, I have added a description of the limitations of our model and future work to the conclusion of Chapter 5.
Author Response File: Author Response.docx
Reviewer 5 Report
Title: A Review of "Dynamic graph convolutional crowd flow prediction model based on residual network structure"
Introduction:
The article "Dynamic graph convolutional crowd flow prediction model based on residual network structure" clearly states the purpose of the study and provides sufficient background information and context for the research question. The research question and hypothesis are also clearly stated.
Methods:
The methods used in the study are appropriate for the research question, and they are described in sufficient detail for the study to be replicated. There are no potential sources of bias or limitations in the study design.
Results:
The results are presented clearly and logically, and the tables and figures are easy to understand and interpret. The results support the conclusions drawn by the authors.
Discussion:
The conclusions are supported by the results of the study, and the limitations of the study are acknowledged and addressed. The implications of the study are clearly stated.
Writing Style:
The article is well-written and easy to understand. The grammar, spelling, and punctuation are correct, and the article is appropriately structured and organized.
Contribution to the Field:
The article makes a contribution to the field and adds to the existing body of knowledge. It is likely to be of interest to readers in the field.
Overall Recommendation:
The study design, methods, results, and conclusions are all sound, and the article makes a valuable contribution to the field. The article should be accepted without revision, as it meets all of the criteria for a good academic publication.
Author Response
Response to Reviewer 5 Comments
Dear Reviewer:
Thanks for your letter and the reviewers' constructive comments concerning our manuscript entitled ' Dynamic Graph Convolutional Crowd Flow Prediction Model Based on Residual Network Structure ' (ID: applsci-2437777). Those comments are all valuable and very helpful for revising and improving our manuscript, and significantly guide our research.
Author Response File: Author Response.docx
Round 2
Reviewer 1 Report
Recommended for acceptance
Recommended for acceptance