Emotional States versus Mental Heart Rate Component Monitored via Wearables
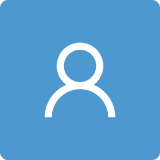
Round 1
Reviewer 1 Report
The paper experiments with emotional state classification using SVM machine learning model for classification. It is based on a dataset of features calculated from Fitbit collected data (accelerometer data and heart rate) and user collected labels for emotions from 18 participants. The features are data-based mechanistic transfer function model orders and parameters.
- The area of emotion recognition and classification using machine learning techniques is an active research area with many challenges and a wide variety of techniques. The manuscript moves from the introduction to the Materials and Methods without enough literature review for the emotions classification research .
- There are some errors in citing references e.g. at line 81 the cited reference [20] is not related to the sentence by any means. Some references are ill-formed e.g. [35][40][41]. All references and appropriate citing need to be rechecked.
- I find this sentence in the introduction section "The good news is that mental illnesses are preventable and treatable." a little bit misleading as not all mental illnesses are preventable and treatable.
- According to Figure 2, the transfer function model takes as input the emotion as input and the output is the HR mental component and based on this the TF model parameters are determined based on the step function of the emotion labeling and the HR mental component calculated from the Mindstretch application. These features are then input to the SVM model. It is not clear for me how these features will be extracted in real data setting for recognizing the emotional status based only on the HR mental component calculated in the Mindstretch application without the process of labeling the emotions.
Author Response
Please see our response to reviewer1 in submitted file.
Author Response File: Author Response.docx
Reviewer 2 Report
This study proposed a method for real-time emotion monitoring, using mental energy as a derived physiological signal. Mental energy is calculated by Mindstretch algorithm based on heart and acceleration data collected by Fitbit activity tracker. A transfer function model was applied to characterize the relationship between the input and the output. Finally, the estimated model orders and parameters were used as features by SVM classifier. However, there are some problems which must be solved before it’s considered for publication.
Firstly, in the abstract part, authors chose average classification accuracy, instead of ROC, as the indicator which represents the performance of the SVM classifier. Please explain the rationality of choosing average classification accuracy as indicator .
Secondly, in the part 2.2, please explain the validity of the model and are there other models that can complete this task.
Thirdly, in the part 3.2, authors chose the SVM as the classifier, I think you need to add some contrast experiments of other classifiers.
Fourthly, in the part 4, this paper is lack of sufficient explanation of the classification result, you need to explain the reason in detail and why you got such result.
Fifthly, in the part ‘limitations’, authors concludes that the best solution for future research is to collect more balanced data of a lower number of emotions. I don’t think so, training a model which can deal with the class imbalance and multiple emotions is a better way.
Finally, there are some additional issues: (1) Some abbreviations are not fully explained in the article, such as ‘mHealth’ and ‘HR’;(2)The title of ‘limitations’ may be labeled incorrectly, please confirm it;(3)You should check the grammar carefully,such as in line 10, ‘to advice users’ should be ‘to advise users’.
Author Response
Please see our response to reviewer2 in file submitted.
Author Response File: Author Response.docx
Reviewer 3 Report
This study considers static heart rate measurements to decide about human emotional state using a simple decision model of one input parameter and one output mental state among 13 defined. There are many limitations of the study – first the experiment is limited, including a limited number of subjects and uneven labelling of emotional states between subjects, apparently subjects did not follow common and well defined instructions on how to label their states. Secondly, it is not an option to define different emotional states together. Third, only one input feature (mean heart rate) has been used for the emotional state decision which is the heart rate. Mush complex models of the human emotions has been presented in literature which never relied on the mean heart rate as a single feature. The reported results are very low 51.1% (abstract) and 49.7% (Table 3). The statistical design of the clinical experiment was not properly defined. The prediction model is too simple for valuable classification. I don’t see any scientific novelty in this study. The paper cannot be published in its present form.
Major Revision Remarks:
1. Title: The title must emphasize the “mean heart rate” (HR) as the parameter used in this study for monitoring of the emotional states. Mean HR is the only parameter input to the classifier “single-input single-output transfer function” to identify emotional state and it is honest to setup this limitation of the study in the title.
2. Title: “Emotional States versus Mental Energy” -> Misleading title: I read the overall paper but didn’t find some parameter that estimates mental energy. The title should be fully rewritten to properly represent the contributions of this study.
3. The heart rate is a parameter that deviates between subjects, deviates over time and depends on the physical loading and cardiac status of the patients (not only affected by emotional state). How do the authors exclude the factor of physical loading and inter-patient physiological differences in the task for mental state identification by a single model? Exclusion of physiological factors and loading cannot be done in applications of wearables, therefore the title, objectives, and discussion in the context of application in wearables is misleading.
4. Abstract: “Mindstretch application”, “Fitbit bracelet” the model orders and parameters were used” -> Indefinite materials (experimental setup), methods and input parameters. The abstract should be an enough informative representation of the methods in the main text.
5. Abstract: Missing definition of the database size and input feature space.
6. Abstract: “average classification accuracy of 51.1%” -> What do you exactly classify? The annotation of the classification groups and the interpretation of the term “classification accuracy” should be identified for a comprehensive understanding of this low performance. Accuracies around 50% are likely to be unusable for reliable interpretation and real-life use of wearables. The authors need to justify the usefulness of such system with obviously very low accuracy. Note that the result 51.1 differs from 49.7% (Table 3) – inconsistency.
7. Abstract: “We concluded that the dynamics of emotions are not only highly variable between individuals as expected, but that they are also time varying on an individual basis.” -> Conclusion not supported by the methods and results. Methods do not specify the intra- and inter-patient measurements, as well as the method for measurement of “dynamics”.
8. Ln 77-8: It is irrelevant to give methodological details for the current implementation in the mid of Introduction. The intention to use of some novel methods can be presented in the last paragraph of Introduction, dedicated to the aims of the study.
9. Ln 79: “measured with Mindstretch smartphone application” -> Give a reference as well as commercial company, city, country. Explain the measurement classification index of mental energy and whether it covers some standards or not. If yes, provide a reference to the standards.
10. Ln 79,80: Unidentified terms: HR, “Fitbit activity tracker”.
11. Ln 95-96: “In this research, mental HR (bpm) is linked to the mental energy, which is the component of the total HR which is used for mental performances.” -> This statement needs more attention and explanation. The definition that part of HR is used for mental performances is uncommon and should be clarified in detail. Do the authors define HR as heart rate variability (with its time-domain or spectral components) or do the authors consider HR as the average heart rate measured in the analysis interval? These are completely different studies on the HR behavior that need to be comprehensively explained.
12. Ln 97-102: AIMs of the study are not clearly defined. Three references are cited [26, 27, 28] which indicates that the study methods and results are already published. What is the novelty of this study? What is the input and output of the application? As soon as commercial algorithms are used, then what are the novel developments? This study should not duplicate former studies in any sense. The objective of the study should be fully rewritten.
13. Ln 108-109: “All participants were in good physical and mental health” -> The lack of patients with mental disease in a study that is targeted to patients with mental disease is a an obvious limitation that need to be indicated in section “Limitations of the study” – a section normally placed after section “Discussion”.
14. Ln 117: 3-axis accelerometers has been defined but the data have never been used. This is confusing.
15. Ln 120: “The Mindstretch algorithm used minute averages of these data” -> The HR is a dymanic variable that is important to be studied in a beat-by-beat basis. The minute averages reduces its informative value of the dynamics, and makes use of its rough average value.
16. Ln 134-135: “The researcher advised to label the emotion right after the experience to achieve an optimal accuracy in the time domain, but labels could also be added later, as well” -> Clarify the statement. What kind of accuracy? Furthermore, induction of delays between current experience and manual annotation of the emotion will surely introduce a bias of the performance evaluation. Explain how you deal with this problem.
17. Ln 143, 146: “mental HR data”, “mental HR signal” -> The term mental HR is not appropriate because HR is the average heart rate. I didn’t understand: Do you evaluate a mental HR component? If yes, please explain comprehensively in the text. What do you mean by “mental HR signal”? This should be explained and a figure illustration need to be included in the text.
18. Ln 151: “(step) input” -> The time-course of the input should be better explained and the input feature map should be shown in an example figure. The analyzed duration of the HR signal should be identified before and after the emotional state.
19. Ln 152-154: “The model parameters were considered being time-invariant, because the emotion processing of an individual in a single instance was not supposed to change over the course of the experience of that emotion.” -> This statement is in conflict with the conclusion in Ln 22-23: “We concluded that the dynamics of emotions are not only highly variable between individuals as expected, but that they are also time varying on an individual basis.” The methods are not designed for analysis of variations over time, therefore conclusions about variations of emotions over time are irrelevant. Correct all such misleading interpretation of the results.
20. Figure 2: Is there a mechanism to regulate against rapid (erroneous) output switching between different emotional states, considering the simple decision scheme when the emotional state output is given for a single input sample?
21. Ln 63: “HR ([bpm]) response” -> improper reference of ([bpm]). The measurement units are not interpretable in the context of methods. Anyway, the measurement units can be specified once in the definition of the HR parameter, as well as should be reported after numerical data.
22. Ln 178: “TF model structure [?a ?b ?]” -> such notation of arguments is NOT defined in methods. The authors need to clarify the meaning of [?a ?b ?].
23. Tables 1,2, Figures 2,3 and related text should be included in sub-section 3.1. Data collection or 3.1. Experimental results or another name (if relevant). The collection of the raw data should be separated from the classification results.
24. Table 1: The distribution of labeled events is very different between subjects, i.e. one subject has marked 0 or 2 labels, while another subject has 583 labels. Obviously, different subjects applied different criteria and frequencies (time intervals) to label their own state, applying its own feeling and obviously on a subjective basis. It is questionable if it is proper to combine such inhomogeneous data together and to process by a single classifier. Justify in the text and setup as a limitation of the study.
25. Table 2: Summary for all emotions should be given in a new column (most right), as well as summary for all responses per person should be included in a new row (bottom).
26. Table 1,2: The training and validation data should be identified. The authors need to identify if the data from the same patient are used in both training and validation. This would give a bias in the results.
27. Figure 3,4: Legend with interpretation of different colors should be included.
28. Ln 266-275: The overall paragraph presents a kind of methodological definitions, which are NOT relevant for section Results. The methodological definitions should be moved where appropriate in section 2.
29. Ln 277-279: “When the optimal model orders and parameters for each labelled event were determined by the mathematical approach, their explanatory value for emotion classification must be searched for. Therefore, the model orders and parameters for each labelled event were used as features (in vector format) in a SVM classifier” -> Incomprehensive statement. Should be fully rewritten.
30. Ln 279-281: “In total, there were four features for each labelled event: number of a-parameters, number of b-parameters, value of the a-parameter(s) and value of the b-parameter(s).” -> This is a methodological detail that should be defined in Methods, where appropriate. It should be linked with specific formula. The values and numbers of a, b should be specified, otherwise incomprehensive. The only definition in methods is about one parameter, named HR, no?
31. Table 3: The formula for computation of the “DBM classification” should be presented in section 2. Methods, where appropriate. Reading the value in results is indefinite about how to interpret.
32. Instead of the case specific confusion matrix in Figure 4 and 5, the authors should present a summary confusion matrix for all participants and emotional states, and this confusion matrix to be used for performance estimation instead of Table 3. The confusion matrix with only one observation per class (Figure 5) indicates biased statistical study where one error vs. 11 observations is equivalent to 9% and within the ‘calm’ class of 2 observations to 50% error. There is a minimal sample size which should be respected to obtain statistically correct results. Obviously, in the patient-by-patient basis, such classification is not relevant to be reported due to the small number of annotations per subject. .
33. The paper does not consider the case when a person can experience several emotional states at the same time.
34. The training of the SVM classifier is not shown. What exactly was optimized in the classifier with one input feature?
35. Discussion: missed to compare to the performances of other such studies in the field.
Author Response
Please see our response to reviewer3 in file submitted.
Author Response File: Author Response.docx
Round 2
Reviewer 2 Report
After reading the revised article, most of the problems have been explained, but there are still some additional issues:
(1) In line 23, ‘support vector machines’ should be ‘support vector machine’.
(2) In part 2.2, there is a format problem which is to start the text on a new line.
(3) In part 3.2 and part 4, there is a format problem which is to indent the first line of the text.
You should check the details carefully of the manuscript before accepted.
Author Response
Dear Reviewer 2,
Many thanks for your suggestions!
- In line 23, ‘support vector machines’ should be ‘support vector machine’.
We have changed this in line 23.
- In part 2.2, there is a format problem which is to start the text on a new line.
This has been adapted.
- In part 3.2 and part 4, there is a format problem which is to indent the first line of the text.
This has been adapted.
We wish you a happy 2023 with in the first place a good health!
Kind regards,
Daniel Berckmans
Author Response File: Author Response.pdf
Reviewer 3 Report
The authors have provided complete answers to my revision comments. I have no further comments. Wish success and Happy New Year 2023!
Author Response
Dear Reviewer 3,
Many thanks for confirming that we have answered all your questions.
We wish you also a happy 2023 with in the first place a good health!
With kind regards,
Daniel Berckmans