Weighted Similarity-Confidence Laplacian Synthesis for High-Resolution Art Painting Completion
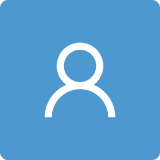
Round 1
Reviewer 1 Report
Comments and Suggestions for AuthorsThe paper provides the idea of deep learning for high-resolution digital image restoration with the Laplacian-based patch method. The underlying idea is to provide improvement over existing solutions in Digital Cultural Heritage.
The authors put stress on several image qualities of the reconstruction process and referred to a number of machine vision models with accompanying metrics useful in digitial media.
‘Patch’ is characterized by distinctive feature of obtaining good effects with few artifacts, however the crucial factor here is the quality of input media. It would be much more valuable, if authors specified the genre(s) of original (digitized) media.
What about the influence of the original object on the proposed algorithm? While the method seems to be very good for film or photography prints with dust and scratches, oil paintings with glaze suffering from varnish or hard oil with 3d structure ‘dropouts’ seem to be much harder to handle…
Does it handle every type of source media with the same efficiency? If so it should be annotated in the text…
Stated 1600×2136 is rather HD+ and good for web display and is far below current display technologies (4K is a standard and 8K is approved standard), what about gigapixel resolutions in professional actual (digital image) restoration of digitized paintings and other works of art? Will performance drop with drastic increase of image resolution?
Author Response
Please check the attached file.
Author Response File: Author Response.pdf
Reviewer 2 Report
Comments and Suggestions for AuthorsThis manuscript proposes a Weighted Similarity-Confidence Laplacian Synthesis, addressing challenges not only in high-resolution artistic image completion but also making a significant contribution to the broader field of patch-based synthesis. Experimental results confirm the effectiveness of this approach, offering promising outcomes for the preservation and restoration of art paintings. However, the manuscript raises several questions that the reviewer would like the authors to address:
1. The introduction section has covered too much related work; it should be further streamlined.
2. In formula one, the subscript in the upsampling formula is inconsistent with the explanation of the formula in the following text.
3. In the comparison of methods for seeking the optimal pixel value on page six, corresponding data should be provided to demonstrate that the proposed method is more optimal.
4. There are some repeated descriptions in this manuscript that increase redundancy.
- The first paragraph on page five and the first paragraph on page eight.
- The first paragraph of experimental results and the first paragraph of the discussion.
Author Response
Please check the attached file.
Author Response File: Author Response.pdf
Reviewer 3 Report
Comments and Suggestions for AuthorsThis paper introduces a groundbreaking approach, integrating Weighted Similarity-Confidence Laplacian Synthesis, to comprehensively address these challenges and advance the field of artistic image completion. The proposed method is of scientific sounds. My concerns are given below.
1. It's better to add the experimental results to Abstract, which will enhance the readability of the paper.
2. Although the authors have pointed out challenges of deep learning-based art painting completion methods in Introduction (Line 73-76). However, the motivation of the proposed method needs to be clearly presented.
3. The main contributions of the paper can be summarized in Introduction.
4. Currently, the Related Work section is a bit disorganized. The authors are suggested to rewrite this part through a timeline or method line.
5. The experimental settings should be detailed so that we can reproduce the experiments.
6. From the Figures 2 and 3. We can see that the missing parts of artistic images are line type. Can the authors use the missing image of the dot type for experiments? In addition, Can the authors do experiments on old faded images? This will be more valuable for real applications such as photo restoration.
7. Generally, the experimental comparison should be done on datasets rather than the current four images.
8. Conclusion: The suggestion of collaborations with art conservationists and experts is commendable. Offering more details on the potential benefits of such collaborations or specific aspects that could be improved through collaboration would add depth.
Author Response
Please check the attached file.
Author Response File: Author Response.pdf
Round 2
Reviewer 3 Report
Comments and Suggestions for AuthorsThe authors have addressed my concerns. It seems that it is ready for publication.
Author Response
Thank you for your review.