Data Processing Unit for Energy Saving in Computer Vision: Weapon Detection Use Case
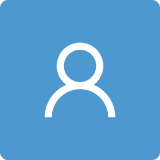
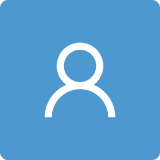
Round 1
Reviewer 1 Report
In the article, the authors presented their study called “Data Processing Unit for energy saving in computer vision: Weapon detection use caseThe authors present a study focused on measuring the reduction of workload on a server dedicated to weapon detection on a real CCTV video stream recording using a DPU. Also, They offer a framework that could be adapted to any computer-vision based detection system. My reviews and suggestions about their publications are listed;
· The mathematical background and technical knowledge of your article is very weak. Authors should provide technical details and mathematical background of their work in the article.
· The importance of the article and its contribution to the literature are not reflected in the abstract. The abstract should include the context or background information for your research; the general topic under study; the specific topic of your research; why is it important to address these questions; the significance or implications of your findings or arguments. It must also contain more numeric values. Please highlight your contribution.
· You should submit more experimental study results for your work. Experimental studies are insufficient. You should also provide comparisons with similar studies (In particular, studies in recent years). What solution you propose to make the system more robust. What is your difference from similar studies?
· Add more recent reference to enhance literature survey section. Discuss the state-of-art techniques with their merits and issues. The literature should be developed and, if possible, presented in more papers published in 2022. Discuss the research gaps and relate how the proposed work has improved them.
· Although some evaluation criteria are given in the article, It should be well supported by Precision, Recall (sensitivity), Accuracy, Specificity, Prevalence, Kappa, and F1-score. These results need to be analyzed, tabulated, presented graphically, and interpreted.
· Conclusion section too long. Rewrite the conclusion with following comment: (a) Highlight your analysis and reflect only the important points for the whole paper. (b) Mention the implication in the last of this section. Please, carefully review the manuscript to resolve these issues. (c) This section should be supported with numerical values.
· The quality of this article is not suitable for journals indexed SCI/SCI-E. Authors need to improve the quality of the article. Especially the mathematical infrastructure should be given in single details. More experimental studies should be submitted and values such as Accuracy, precision, F1 score should be presented.
Author Response
In the article, the authors presented their study called “Data Processing Unit for energy saving in computer vision: Weapon detection use case. The authors present a study focused on measuring the reduction of workload on a server dedicated to weapon detection on a real CCTV video stream recording using a DPU. Also, They offer a framework that could be adapted to any computer-vision based detection system. My reviews and suggestions about their publications are listed.
Thank you for your review and your helpful comments. Following the editor's invitation, we have prepared a new version of our manuscript to address your concerns. The writing has also been improved.
1. The mathematical background and technical knowledge of your article is very weak. Authors should provide technical details and mathematical background of their work in the article.
Thank you for your comment. We have included technical details of the motion detection algorithm and mathematically defined the threshold percentage of time with activity, beyond which our approach becomes less energy effective. Changes are marked in red and included in Section 4.2 and Section 5.
2. The importance of the article and its contribution to the literature are not reflected in the abstract. The abstract should include the context or background information for your research; the general topic under study; the specific topic of your research; why is it important to address these questions; the significance or implications of your findings or arguments. It must also contain more numeric values. Please highlight your contribution.
Thank you for your comment. We have improved the abstract by including your proposals.
“The growth of the Internet has led to the emergence of servers that perform increasingly heavy tasks. Some servers must remain active 24 hours a day, but the evolution of network cards has facilitated the use of Data Processing Units (DPUs) to reduce network traffic and alleviate server workloads. This capability makes DPUs good candidates for load alleviation in systems that perform continuous data processing when the data can be pre-filtered. Computer vision systems that use some form of artificial intelligence, such as facial recognition or weapon detection, tend to have high workloads and high power consumption, which is becoming increasingly costly. Reducing workload is therefore desirable and possible in some scenarios. The main contributions of this study are threefold: 1) to explore the potential benefits of using a DPU to alleviate the workload of a 24-hour active server; 2) to present a study that measures the workload reduction of a CCTV weapon detection system and evaluates its performance under different conditions. We observed a 43,123% reduction in workload over the 24 hours of video used in the experimentation, reaching more than 98% savings during night hours, which significantly reduces system stress and has a direct impact on electrical energy expenditure; and 3) to provide a framework that can be adapted to other computer vision-based detection systems.”
3. You should submit more experimental study results for your work. Experimental studies are insufficient. You should also provide comparisons with similar studies (In particular, studies in recent years). What solution you propose to make the system more robust. What is your difference from similar studies?
Thank you for your comment. Another experimental study has been added, checking that, for the experiment conducted, the ratio of false positives detected can be reduced since, during periods of inactivity, it is implausible that there is an armed attacker. We also highlight, in Section 2, the difference with other studies in allowing us to save energy without sacrificing frames with weapon detections since it is impossible to assume skip-lengths based on previous data due to the appearance of a weapon being an anomalous and non-programmed event. For this reason, it was not possible to make a direct comparison with them.
4. Add more recent reference to enhance literature survey section. Discuss the state-of-art techniques with their merits and issues. The literature should be developed and, if possible, presented in more papers published in 2022. Discuss the research gaps and relate how the proposed work has improved them.
Thank you for your comment. The related works section has been improved, including more recent studies and related cost-saving techniques, analysing their application to our use case. Changes are marked in red and included in Section 2.
5. Although some evaluation criteria are given in the article, It should be well supported by Precision, Recall (sensitivity), Accuracy, Specificity, Prevalence, Kappa, and F1-score. These results need to be analyzed, tabulated, presented graphically, and interpreted.
Thank you for your comment. The metrics indicated are found in the article of Salazar et al. [18], as the model was taken from their study without modification, highlighted in Section 3.1. Our system focuses on energy savings, so it does not modify the detection model. Nonetheless, we have included a new false positive analysis in Section 5. On the other hand, the video used to test our energy-saving system comes from a public camera at the University of Michigan, captured over 24 hours, without weapons presence found; hence no other metrics can be applied.
[18] Salazar González, J.L.; Zaccaro, C.; Álvarez-García, J.A.; Soria Morillo, L.M.; Sancho Caparrini, F. Real-time gun detection in CCTV: an open problem. Neural Networks 2020, 132, 297–308.
6. Conclusion section too long. Rewrite the conclusion with following comment: (a) Highlight your analysis and reflect only the important points for the whole paper. (b) Mention the implication in the last of this section. Please, carefully review the manuscript to resolve these issues. (c) This section should be supported with numerical values.
Thank you for your comment. We have rewritten the conclusions section to reduce it and include your proposals.
“As far as we know, this is the first time a DPU has been used as a pre-filter in a computer vision detection task. The code used in this work is available on our GitHub account and can be adapted for use in other computer vision systems where the pre-filtering of input data is possible. As demonstrated in the previous sections, using a pre-filtering device can significantly reduce server workload and energy consumption. In our tests, energy savings ranged from 20.54% during periods of high-activity, to 68.7% during periods of medium-activity and up to 98.07% during periods of low-activity. This methodology is beneficial when there is no constant flow of people, but active surveillance is necessary at all times, such as in universities, public institutions, military buildings, and museums.
Additionally, the use of DPUs as load balancers allows for the distribution of workload in real-time video processing, as discussed in [23]. While we could not test this method due to only having one server capable of weapon detection, it is worth noting that the DPUs could distribute video frames among multiple servers rather than discarding them.”
[23] Watanabe, H.; Ghatp, A.; Nakazato, H. Distributed Computing for Real-time Video Processing, 2003.
7. The quality of this article is not suitable for journals indexed SCI/SCI-E. Authors need to improve the quality of the article. Especially the mathematical infrastructure should be given in single details. More experimental studies should be submitted and values such as Accuracy, precision, F1 score should be presented.
We have deeply reviewed the article and modified the study with your proposals. The study has improved considerably due to the proposals you made to us.
Author Response File: Author Response.pdf
Reviewer 2 Report
In your article, you used a Data Processing Unit (DPU) to reduce the workload and power consumption on a server whose task is to detect armed persons in a public building.
you followed the process timeline, you tested your model on real time data and you got very satisfactory results.
I would have liked you to talk more about the GPU to enhance its use in weapon detection.
In figure 3, the diagram is not exhaustive. You did not treat the case where the CPU detects frames without motion
Author Response
In your article, you used a Data Processing Unit (DPU) to reduce the workload and power consumption on a server whose task is to detect armed persons in a public building. You followed the process timeline, you tested your model on real time data and you got very satisfactory results.
Thank you for your review and your helpful comments. Following the editor's invitation, we have prepared a new version of our manuscript to address your concerns. The writing has also been improved.
1. I would have liked you to talk more about the GPU to enhance its use in weapon detection.
Thank you for your comment. We have specified that the use of the GPU to enhance weapon detection is not the central goal of our system but is used in order to be able to assume real-time inference from the original model. To highlight this, changes are marked in red and included in Section 3.1 (Implementation details).
2. In figure 3, the diagram is not exhaustive. You did not treat the case where the CPU detects frames without motion.
Thank you for your comment. You were right. We have modified the diagram to include the case where the DPU does not alert the CPU (no motion detected).
Author Response File: Author Response.pdf
Reviewer 3 Report
The paper is well written and provides a useful approach for reducing the server power consumption in the proposed scenario. However it appears to me that in high-activity cases the proposed approach would determine an increase of the overall power consumption (as shown in Fig. 5) and not a reduction as stated in the text: it must be explained how the 20.54% saving was calculated. I suppose it depends on the definition of high-activity: by considering 75% as threshold for percentage of the time when there are people in the viewing range of the camera, there is a reduction as 25% of the time can be discarded by the prefiltering. Therefore, the usefulness of the approach strongly depends on the scenario: in a scenario where high-activity (almost all the time frames must be processed because there are people in them) is the dominant case, the proposed approach would be detrimental in my opinion, as there would be an increase in power consumption (the BF power consumption would add to the one of the GPU, as shown in Fig. 5). The limits of the proposed approach should also be studied and evaluated in order to fully understand the usefulness of the proposed approach in general and not limited to the proposed scenario. In particular it would be useful to know which is the threshold in percentage of the time when there are people in the viewing range of the camera that allows achieving with the proposed approach the same power consumption as with the standard one.
Author Response
The paper is well written and provides a useful approach for reducing the server power consumption in the proposed scenario. However it appears to me that in high-activity cases the proposed approach would determine an increase of the overall power consumption (as shown in Fig. 5) and not a reduction as stated in the text: it must be explained how the 20.54% saving was calculated. I suppose it depends on the definition of high-activity: by considering 75% as threshold for percentage of the time when there are people in the viewing range of the camera, there is a reduction as 25% of the time can be discarded by the prefiltering. Therefore, the usefulness of the approach strongly depends on the scenario: in a scenario where high-activity (almost all the time frames must be processed because there are people in them) is the dominant case, the proposed approach would be detrimental in my opinion, as there would be an increase in power consumption (the BF power consumption would add to the one of the GPU, as shown in Fig. 5). The limits of the proposed approach should also be studied and evaluated in order to fully understand the usefulness of the proposed approach in general and not limited to the proposed scenario. In particular it would be useful to know which is the threshold in percentage of the time when there are people in the viewing range of the camera that allows achieving with the proposed approach the same power consumption as with the standard one.
Thank you for your review and your helpful comments. Following the editor's invitation, we have prepared a new version of our manuscript to address your concerns. The writing has also been improved.
We consider your proposal very interesting and enriching. We have included a detailed study in the Results section, declaring the threshold of movement from which our system is not energetically optimal and calculating the percentage of time with activity at which GPU and DPU consumption is higher than a traditional GPU-only system, thus indicating when our system is beneficial. To highlight this, changes are marked in red and included in the Section 5.
Author Response File: Author Response.pdf
Round 2
Reviewer 1 Report
I have reviewed the revised manuscript title "Data Processing Unit for energy saving in computer vision: Weapon detection use case". After revising my initial comments and comparing the changes, done by the authors, with them. I found that the authors addressed and answered most of the comments efficiently. Overall, the revised manuscrip is well organized and carefully prepared. The response letter was elegant and satisfactory. I thank the authors for their kind responses. The authors have sufficiently address my all comments. So, I think it is appropriate to accept the revised article. The authors have addressed all the concerns and responded to the review comments. The manuscript can be published in this journal.
Reviewer 3 Report
The authors successfully addressed my previous concerns.