FedUB: Federated Learning Algorithm Based on Update Bias
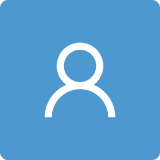
Round 1
Reviewer 1 Report
Comments and Suggestions for AuthorsThe authors nicely presented Federated Learning Algorithm Based on Update Bias which results in better performance and faster convergence of tasks. Still, suggestions are:
1. Equations must be cited.
2. References must be rewritten. Some of the references pp is missing.
Author Response
Please see the attachment.
Author Response File: Author Response.docx
Reviewer 2 Report
Comments and Suggestions for Authors· The benchmarked algorithm (FedProx, Scaffold, FedDyn, FedAvg, and FedDC) reference number should be indicated in your simulation diagram so as to validate your algorithm.
· The computational complexity of the proposed algorithm needs to be indicated.
· More detailed Related Work could be provided.
Comments on the Quality of English Language· The benchmarked algorithm (FedProx, Scaffold, FedDyn, FedAvg, and FedDC) reference number should be indicated in your simulation diagram so as to validate your algorithm.
· The computational complexity of the proposed algorithm needs to be indicated.
· More detailed Related Work could be provided.
Author Response
Please see the attachment.
Author Response File: Author Response.docx
Reviewer 3 Report
Comments and Suggestions for AuthorsThe paper presents a novel federated learning algorithm, FedUB, designed to address challenges arising from non-iid data distributions in federated learning settings. The authors conducted extensive experiments across various datasets to evaluate the performance of FedUB compared to existing federated learning algorithms. Overall, the paper presents interesting findings and contributes to the growing body of research in federated learning. However, there are several areas that need improvement before the paper can be considered for publication.
1- The paper lacks a detailed theoretical analysis of the FedUB algorithm. While the empirical results are promising, a deeper theoretical understanding of how FedUB addresses non-iid data distributions would strengthen the paper's contribution.
2- The paper compares FedUB against several federated learning algorithms, but the comparison lacks depth. More extensive comparisons with state-of-the-art methods, including analysis of convergence rates, computational efficiency, and robustness to hyperparameters, would provide a clearer understanding of FedUB's advantages.(Consider these papers as sample: ''FedBR: Improving Federated Learning on Heterogeneous Data via Local Learning Bias Reduction'' and ''Enhancing federated learning robustness through randomization and mixture''.
3- The paper does not adequately discuss the limitations of FedUB. Addressing potential drawbacks or scenarios where FedUB may underperform would provide a more balanced evaluation of the algorithm's efficacy.( For example what is the effect of aggregation function on FedHUB, Please check litrature in the Robust Aggregation Function Federated Learning)
4- English proofread is required.
Comments on the Quality of English LanguageN/A
Author Response
Please see the attachment.
Author Response File: Author Response.docx
Reviewer 4 Report
Comments and Suggestions for AuthorsIt would be beneficial to discuss a more comprehensive discussion on the specific challenges addressed by FedUB and how it improves upon existing FL algorithms.
More clarity required on the mathematical formulation of the update bias term and how it influences the aggregation process across distributed clients.
Provide insights into the selection of hyperparameters and any sensitivity analyses conducted to validate the robustness of FedUB across different datasets and network configurations.
A minimum of 10-15 recent publications should be taken and explored.
Figures are of poor resolution and clarity. They need to be revised with high resolution
The novelty of the proposed approach should be validated by the authors in the discussion.
More clarity on the data set is required
Conclusion should be revised by describing the features of proposed model along with its limitation, future scope and application.
Comments on the Quality of English Language
Minor editing of English language required
Author Response
Please see the attachment.
Author Response File: Author Response.docx
Round 2
Reviewer 3 Report
Comments and Suggestions for AuthorsThe authors have answered my questions.