Summary-Sentence Level Hierarchical Supervision for Re-Ranking Model of Two-Stage Abstractive Summarization Framework
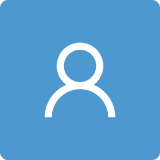
Round 1
Reviewer 1 Report
Comments and Suggestions for AuthorsThis paper proposes an abstractive summarization method by re-ranking the model that utilizes hierarchical supervision during training to address the two limitations of the existing re-ranking model in the two-stage abstract summary framework.
The author can narrate the story from their perspective effectively, enabling readers to easily follow key points such as the research gap and goals. However, a strong domain understanding is required to comprehend the narrative related to the method and results. For readers new to this issue, it can be challenging to understand what the author is conveying.
The authors have presented their results comprehensively and compared them with the baseline. If possible, the authors need to compare them with other relevant studies.
Author Response
Please see the attachment.
Author Response File: Author Response.pdf
Reviewer 2 Report
Comments and Suggestions for AuthorsThe paper addresses limitations of two-stage abstractive summarization framework, proposing a different method of training based on different losses defined over the sentences that compose each summary.
The work is well done and presented. It is scientifically sound and there are no evident errors or lacks.
There are some typos, but nothing critical.
Anyway Figure 3 is not clear, and neither is its explanation in the text. Its meaning should be explained better, and maybe the pie chart is not the best graphical tool in this case; maybe a distribution plot over the values could be more useful.
Author Response
Please see the attachment.
Author Response File: Author Response.pdf
Reviewer 3 Report
Comments and Suggestions for AuthorsThe paper presents a hierarchical supervision method for two-stage abstractive text summarisation. The paper is relevant for the journal and the special issue on Advances in Artificial Intelligence: Models, Optimization, and Machine Learning.
The paper is well structured and clearly organised. The problem is clearly stated and discussed in sufficient details in the view of the state-of-the art and recent advances in the field, justifying the choice of method. The figures and examples are illustrative and improve the readability of the paper.
The particulars of the method have been presented in details - summary scores, ranking loss formulae, etc.
In my opinion, the experiments, the implementation and the discussion need more focus. My main concern is the claim that the method outperforms current state-of-the art, while the improvement (if any) is not very convincing. While exploring a new method is relevant and it is not necessary to outdo existing methods, some claims in the paper need to be reformulated.
A discussion explaining the factors determining the performance of the method would be beneficial. The presented considerations of the dataset properties and noise are one way to go to address the performance and seek for ways to improve. Also, the limitations as outlined in the conclusion, are more of an opportunity to investigate the method's performance and tune for texts of particular types (domain specific, etc.).
Tables 2 and 3 captions are somewhat unclear.
intra sentence, intra-sentence - I recommend consistency
Author Response
Please see the attachment.
Author Response File: Author Response.pdf
Reviewer 4 Report
Comments and Suggestions for AuthorsThe authors highlight two existing issues in the two-stage abstractive summarization framework. The first is the framework has difficulty learning detailed and complex information. The second is that there is potential bias in encoder-decoder re-ranking models. A solution using a hierarchical supervision method that jointly performs summary and sentence-level supervision is proposed. It is evaluated against a number of alternatives and in some settings provides superior performance.
Major Comments:
(1) The paper would benefit from a more detailed explanation as to why the alternative approaches in the evaluation were chosen for comparison against the proposed approach. Most readers will expect this evaluation to include ChatGPT (especially with mention/citation of BART and BERT) it should be directly stated why it is either not capable of abstractive summarization or it is not fit for comparison in this evaluation. In addition, it is needs to be made more clear why CNN/DM and the XSum datasets where chosen. The authors highlight the need to evaluate effectiveness of hierarchical supervision in different summary structures is clear. However, it is not clear why other established industry standard datasets for this type of evaluation like the DUCCorpus (https://duc.nist.gov/duc2004/tasks.html) were not included for a more robust evaluation.
(2) Motivating the need for more detailed exploration into the biases of generated text summary/messages and their ability to reflect complexity and detail would increase scope and interest in the paper. Several recent publications have explored how generated text summaries and narratives can contain biases and misrepresent detailed and complex information. In their exploration they have identified similar issues to those suggested by the author with approaches to address them. These include:
An assessment of the extent to which generated text summarizing life events accurately reflects the sentiment used in human announcements of the same life events. This study looks to understand the role sentiment plays in generated text to create more realistic and correct text summaries of life events.
Lynch, Christopher J., et al. "A Structured Narrative Prompt for Prompting Narratives from Large Language Models: Sentiment Assessment of ChatGPT-Generated Narratives and Real Tweets." Future Internet 15.12 (2023): 375.
Others have created heuristics to control the amount of text that is copied exactly into the summary from source material to generate a more diverse, readable summary.
Song, Kaiqiang, et al. "Controlling the amount of verbatim copying in abstractive summarization." Proceedings of the AAAI conference on artificial intelligence. Vol. 34. No. 05. 2020.
(3) For the evaluation data presented for in tables 2-6 the best and at times the worst performing approach is identified. However, a number of issues with this analysis exist. First, it is unclear if the differences in the performance of the different tested approaches is statistically significantly different from one another. In other words in any evaluation one approach will perform the best and one will perform the worst. However, a rigorous evaluation needs to demonstrate the the difference in performance for the best approach is materially different from the other approaches by testing the data and showing that the increase in performance is statistically significant. Furthermore, table is unclear as there are multiple "best performing approaches" (i.e. SummaReranker - 40.00 and proposed+intra 39.99) with different results. It is not clear to readers why two approaches with different performance measures would both be labeled as highest.
(4) In the replication crisis era the source code used to generate the graphics and data used to for the analysis should be made available to reviewers and readers. It is not enough to say further inquiries can be directed to the corresponding author/s. In addition showing the source code used to conduct the analysis ensures transparent and replicable work and increases the likelihood that the paper will be useful (and cited) to/by readers.
Minor Comments:
(5) The superscripts and subscripts associated with the elements of Figure 2 are not clearly explained in a legend associated with the figure. As it is currently constructed the figure is not self-explanatory. this should be addressed by adding a legend or improving the explanation in the caption to clarify the meaning of the variables, superscripts and subscripts.
(6) The data is table 6 is not right justified. For the numeric data presented in the tables it makes it difficult for readers to compare results between rows because significant digits do not alight. Using right justification would enable comparison and improve the paper.
(7) The data in Table 6 does not feature numeric data with the same number of significant digits. This should be addressed so all numeric data has the same number of significant digits.
Author Response
Please see the attachment.
Author Response File: Author Response.pdf
Round 2
Reviewer 4 Report
Comments and Suggestions for AuthorsAll of my concerns related to the paper have been sufficiently addressed by the authors. It is now suitable for publication.