Assessing Maize Yield Spatiotemporal Variability Using Unmanned Aerial Vehicles and Machine Learning
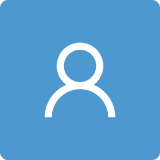
Round 1
Reviewer 1 Report
Comments and Suggestions for AuthorsThe manuscript describes a study on predicting Maize yields from spectral and textural indices obtained at different time points during crop growth. Machine learning algorithms have been used to predict crop yield and different algorithms have been evaluated. I found this manuscript well structured, contained all the details required, and was very easy to follow the content. Overall, I like the simplistic approach of presenting the results with good-quality figures. The main concern I have throughout this manuscript is that the authors have referred to the calendar dates when growth stages were described. This information is not generalizable. Therefore, I propose to use actual growth stages throughout the manuscript as this information is very important. Other than this I have some minor comments made within the manuscript.
Comments for author File: Comments.pdf
Author Response
Please see the attachment.
Author Response File: Author Response.pdf
Reviewer 2 Report
Comments and Suggestions for AuthorsDear all, the work needs some improvements in terms of methodology, some results and mainly discussion
Line 152-153: The soil moisture of 2.92% caught my attention. We are talking about extremely low humidity, at the point of permanent wilting. Question:
Is this value correct?
Was this moisture measured on a gravimetric or volumetric basis?
Was it related to irrigation?
Line 165: Provide information on the planting date and the crop cycle.
Line 194: Provide more details on how panel-less calibration works. Therefore, the radiometric data may not present good results in the absence of the panel. The panel is essential for correcting reflectance under natural lighting conditions at the time of monitoring, but it was not used, which may compromise the quality of the analysis. Explain in detail how panel-less radiometric calibration works.
Line 280: The metrics used are standard, commonly used metrics, which is correct. However, it is beneficial to add a relative metric so that the reader can evaluate the proportions of uncertainty. I recommend including both relative RMSE and/or MAPE.
3. Results
Line 298: Figure 3 shows many predictor variables and their correlation with corn productivity. However, there is no correlation matrix showing the correlations between variables. Many of the variables in Figure 3 are highly correlated with each other, such as NDVI and NDRE, among others. High correlations in machine learning models present variable redundancy and, in some cases, collinearity, where one variable interferes with another. Whether due to redundancy or collinearity, highly correlated variables must be reduced. Apply a correlation threshold above which strongly correlated variables are removed. I also suggest applying a feature selection method, such as Recursive Feature Elimination, to avoid including unnecessary variables in the model.
Line 337: The RMSE values represent what percentage of error? Absolute values do not faithfully show the uncertainty of the models. Therefore, it is appropriate to also use a relative metric.
Line 418: After line 418, add a table containing the test and cross-validation results so that it is possible to more clearly evaluate if overfitting occurs in the models.
Line 460: In Figure 8a, the values are represented by "." instead of ",". Points serve as decimal places. Based on the data in this figure, productivity is 3 kg and 49 grams for the smallest and 5 kg and 88 grams for the largest. To correct, either remove the period or replace it with a comma.
4. Discussion
The discussion is weak; it seems more like a synthesis of results than an actual discussion. Here, the results need to be explained. For example:
Why was PBI the most important variable in the Catboost model?
Why were the PBI, Green-entropy, and Green-homogeneity variables the three main ones in GradBoost?
Why was Green-dissimilarity the most important in RF?
Why was Green-homogeneity the most important for XGBoost?
Please explain the importance and relationship of these variables to corn cultivation and productivity, as they were the most significant.
Comments for author File: Comments.docx
Author Response
Please see the attachment.
Author Response File: Author Response.pdf
Reviewer 3 Report
Comments and Suggestions for AuthorsIn this paper, a maize yield detection based on machine learning approaches is presented. The paper is interesting. But I think the manuscript can be improved according to the following comment.
· Objectives of the study is presented but it is not clear what is new with respect to the previous studies.
· Figure1-b: please define curves in a legend. I am not sure this sub-figure is essential.
· Please provide a subsection regarding methodology in the material and method section.
· Workflow: ground parameter is entered into feature selection, but it seems that ground samples are used in machine learning approaches.
· Please present a subsection regarding descriptions of machine learning approaches.
· Descriptions such as below must be presented in the result section:
For optimization of the algorithm fine-tuning of hyperparameters was needed. This included the number of decision trees (n_estimators = 100, 200, 300), the maximum depth of each tree (max_depth = None, 5, 10) and the minimum samples required to split the node (min_sample_split = 2, 5, 10)
Author Response
Please see the attachment.
Author Response File: Author Response.pdf
Reviewer 4 Report
Comments and Suggestions for AuthorsModerate editing of English language required
Comments on the Quality of English LanguageModerate editing of English language required
Author Response
Please see the attachment.
Author Response File: Author Response.pdf
Reviewer 5 Report
Comments and Suggestions for AuthorsThe aim of this research is to investigate the capability of machine learning algorithms to predict maize yield using UAV (Unmanned Aerial Vehicles)-derived data and evaluate the spatiotemporal variability through the phenological cycle of the crop in Bronk horstspruit, South Africa. Five spectral bands (Red, Green, Blue, Near-Infrared, and Red-Edge) of the UAV data, vegetation indices and gray-level co-occurrence matrix (GLCM) textural features computed from the bands. Crop yield prediction was then conducted using our machine learning (ML) regression models, including Random Forest (RF), Gradient Boosting (GradBoost), Categorical Boosting (Catboost), and Extreme Gradient Boosting (XGBoost). The Catboost regression showed the best overall model accuracy with R2 ranging from 0.06 to 0.62 and RMSE from 2.00 to 2.88 kg/ha. The yield variability across the growing season indicated that overall higher yield values were predicted in the two later growth periods (2022/04/06 and 2022/05/18) for both maize fields. Analysis of variance (ANOVA) using the Welch’s test indicated statistically significant differences in maize yields from early through late-matured growing stages of the crop (p-value < 0.01). The findings of this work confirms the utility of UAV data andmachine learning modelling in detecting yield variations across space and time within smallholder farming environments. Assessing the spatiotemporal variability of maize yields in such environments accurately and timely improves decision-making essential for ensuring sustainable crop production.
Regarding the relevance to the field, the four machine learning algorithms used
(RF, GradBoost, XGBoost and Catboost) are “state of the art”. Besides this, feature selection, which is also an important metodology to improve the performce of algorithms, was done.
As predictor variables, the authors have used a wide range of textural properties and spectral vegetation indices
Regarding the statisticl análisis, the statistics and test used to compare the results were also appropriate.
I consider that no further improvements nor controls should be considered.
Conclusions are consistent with the evidence and arguments presented.
References are appropriate. Tables, figures, and quality of the data are OK.
I indicatea few corrections to the text.
I recommend accepting after minor revisión.
Comments on the Quality of English LanguageI understand that there is an error with the units, where it says kg/ha it should say ton/ha.
Figure 3. It is not a Heat map . I would put: Feature relevance plot based on Pearson`s correlation coefficients
Figure 8. Comparison of maize yield prediction maps wit...
A map with the observed values would help to compare the performance of the algorithm in the different stages
48 ... and plow penetration of soils[6,7]. add space
131 ... exercise by growth stage [28-30,35-37]. add space ( same line 133)
184 including Red (663-673 nm), Green ( 550-570 nm), Blue ( 465-485 nm), Red-Edge ( 712-722 nm) and Near infrared ( 820-860 nm) bands, delete space…
448 ...age on the 2022/02/23 (Figure8b), add space
Author Response
Please see the attachment.
Author Response File: Author Response.pdf
Round 2
Reviewer 2 Report
Comments and Suggestions for AuthorsThe text has undergone significant improvements, and I believe it is ready for publication.
Reviewer 3 Report
Comments and Suggestions for AuthorsAccept as it is.