Journal Description
Geomatics
Geomatics
is an international, peer-reviewed, open access journal on geomatic science published quarterly online by MDPI.
- Open Access— free for readers, with article processing charges (APC) paid by authors or their institutions.
- High Visibility: indexed within ESCI (Web of Science), Scopus, EBSCO, and other databases.
- Rapid Publication: manuscripts are peer-reviewed and a first decision is provided to authors approximately 22.1 days after submission; acceptance to publication is undertaken in 3.3 days (median values for papers published in this journal in the second half of 2024).
- Recognition of Reviewers: APC discount vouchers, optional signed peer review, and reviewer names published annually in the journal.
- Companion journal: Remote Sensing.
Latest Articles
Tropical Forest Carbon Accounting Through Deep Learning-Based Species Mapping and Tree Crown Delineation
Geomatics 2025, 5(1), 15; https://doi.org/10.3390/geomatics5010015 - 19 Mar 2025
Abstract
►
Show Figures
Tropical forests are essential ecosystems recognized for their carbon sequestration and biodiversity benefits. As the world undergoes a simultaneous data revolution and climate crisis, accurate data on the world’s forests are increasingly important. Completely novel in approach, this study proposes a methodology encompassing
[...] Read more.
Tropical forests are essential ecosystems recognized for their carbon sequestration and biodiversity benefits. As the world undergoes a simultaneous data revolution and climate crisis, accurate data on the world’s forests are increasingly important. Completely novel in approach, this study proposes a methodology encompassing two bespoke deep learning models: (1) a single encoder, double decoder (SEDD) model to generate a species segmentation map, regularized by a distance map in training, and (2) an XGBoost model that estimates the diameter at breast height (DBH) based on tree species and crown measurements. These models operate sequentially: RGB images from the ReforesTree dataset undergo preprocessing before species identification, followed by tree crown detection using a fine-tuned DeepForest model. Post-processing applies the XGBoost model and custom allometric equations alongside standard carbon accounting formulas to generate final sequestration estimates. Unlike previous approaches that treat individual tree identification as an isolated task, this study directly integrates species-level identification into carbon accounting. Moreover, unlike traditional carbon estimation methods that rely on regional estimations via satellite imagery, this study leverages high-resolution, drone-captured RGB imagery, offering improved accuracy without sacrificing accessibility for resource-constrained regions. The model correctly identifies 67% of trees in the dataset, with accuracy rising to 84% for the two most common species. In terms of carbon accounting, this study achieves a relative error of just 2% compared to ground-truth carbon sequestration potential across the test set.
Full article
Open AccessReview
The Application of Earth Observation Data to Desert Locust Risk Management: A Literature Review
by
Gachie Eliud Baraka, Guido D’Urso and Oscar Rosario Belfiore
Geomatics 2025, 5(1), 14; https://doi.org/10.3390/geomatics5010014 - 18 Mar 2025
Abstract
The desert locust is documented as one of the most destructive polyphagous plant pests that require preventive or proactive management practices due to its phase polyphenism, rapid breeding, transnational migration, and heavy feeding behaviour. Desert locust situation analysis, forecasting and early warning are
[...] Read more.
The desert locust is documented as one of the most destructive polyphagous plant pests that require preventive or proactive management practices due to its phase polyphenism, rapid breeding, transnational migration, and heavy feeding behaviour. Desert locust situation analysis, forecasting and early warning are complex due to the systemic interaction of biological, meteorological, and geographical factors that play different roles in facilitating the survival, breeding and migration of the pest. This article seeks to elucidate the factors that affect desert locust distribution and review the application of earth observation (EO) data in explaining the pest’s infestations and impact. The review presents details concerning the application of EO data to understand factors that affect desert locust breeding and migration, elaborates on impact assessment through vegetation change detection and discusses modelling techniques that can support the effective management of the pest. The review reveals that the application of EO technology is inclined in favour of desert locust habitat suitability assessment with a limited financial quantification of losses. The review also finds a progressive advancement in the use of multi-modelling approaches to address identified gaps and reduce computational errors. Moreover, the review recognises great potential in applications of EO tools, products and services for anticipatory action against desert locusts to ensure resource use efficiency and environmental conservation.
Full article
(This article belongs to the Topic Geographic Information and Remote Sensing Technology (GIRST))
Open AccessArticle
Identification of Vegetation Areas Affected by Wildfires Using RGB Images Obtained by UAV: A Case Study in the Brazilian Cerrado
by
Miguel Julio Machado Guimarães, Ian Dill dos Reis, Juliane Rafaele Alves Barros, Iug Lopes, Marlon Gomes da Costa, Denis Pereira Ribeiro, Gian Carlo Carvalho, Anderson Santos da Silva and Carlos Vitor Oliveira Alves
Geomatics 2025, 5(1), 13; https://doi.org/10.3390/geomatics5010013 - 16 Mar 2025
Abstract
►▼
Show Figures
The Cerrado is Brazil’s second largest biome, covering continuous areas in several states. Covering approximately 23% of Brazil’s territory, the Cerrado biome connects with all the main biomes in South America, thus forming a major biological corridor. This biome is one of those
[...] Read more.
The Cerrado is Brazil’s second largest biome, covering continuous areas in several states. Covering approximately 23% of Brazil’s territory, the Cerrado biome connects with all the main biomes in South America, thus forming a major biological corridor. This biome is one of those that has suffered the most from the incidence of wildfires, leading to a progressive depletion of the region’s natural resources. The aim of this study was to evaluate the use of an Unmanned Aerial Vehicle (UAV) embedded with an RGB sensor to obtain high-resolution digital products that can be used to identify areas of the Brazilian Cerrado affected by wildfires. The study was carried out in a savannah biome area selecting a vegetation corridor with native vegetation free from anthropogenic influence. The following UAV surveys were carried out before and after a burning event. Once the orthomosaics of the area were available, the GLI, VARI, ExG and NGRDI vegetation indices were used to analyze the vegetation. The data indicate that the B band and the GLI and ExG indices are more suitable for environmental impact analysis in Cerrado areas affected by fires, providing a solid basis for environmental monitoring and management in scenarios of fire disturbance.
Full article
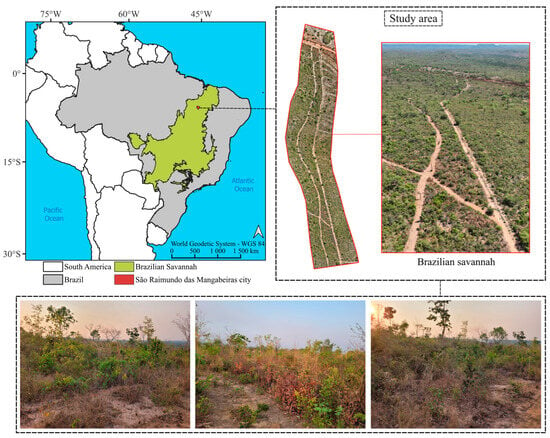
Figure 1
Open AccessArticle
Improving the Individual Tree Parameters Estimation of a Complex Mixed Conifer—Broadleaf Forest Using a Combination of Structural, Textural, and Spectral Metrics Derived from Unmanned Aerial Vehicle RGB and Multispectral Imagery
by
Jeyavanan Karthigesu, Toshiaki Owari, Satoshi Tsuyuki and Takuya Hiroshima
Geomatics 2025, 5(1), 12; https://doi.org/10.3390/geomatics5010012 - 10 Mar 2025
Abstract
Individual tree parameters are essential for forestry decision-making, supporting economic valuation, harvesting, and silvicultural operations. While extensive research exists on uniform and simply structured forests, studies addressing complex, dense, and mixed forests with highly overlapping, clustered, and multiple tree crowns remain limited. This
[...] Read more.
Individual tree parameters are essential for forestry decision-making, supporting economic valuation, harvesting, and silvicultural operations. While extensive research exists on uniform and simply structured forests, studies addressing complex, dense, and mixed forests with highly overlapping, clustered, and multiple tree crowns remain limited. This study bridges this gap by combining structural, textural, and spectral metrics derived from unmanned aerial vehicle (UAV) Red–Green–Blue (RGB) and multispectral (MS) imagery to estimate individual tree parameters using a random forest regression model in a complex mixed conifer–broadleaf forest. Data from 255 individual trees (115 conifers, 67 Japanese oak, and 73 other broadleaf species (OBL)) were analyzed. High-resolution UAV orthomosaic enabled effective tree crown delineation and canopy height models. Combining structural, textural, and spectral metrics improved the accuracy of tree height, diameter at breast height, stem volume, basal area, and carbon stock estimates. Conifers showed high accuracy (R2 = 0.70–0.89) for all individual parameters, with a high estimate of tree height (R2 = 0.89, RMSE = 0.85 m). The accuracy of oak (R2 = 0.11–0.49) and OBL (R2 = 0.38–0.57) was improved, with OBL species achieving relatively high accuracy for basal area (R2 = 0.57, RMSE = 0.08 m2 tree−1) and volume (R2 = 0.51, RMSE = 0.27 m3 tree−1). These findings highlight the potential of UAV metrics in accurately estimating individual tree parameters in a complex mixed conifer–broadleaf forest.
Full article
(This article belongs to the Topic Vegetation Characterization and Classification With Multi-Source Remote Sensing Data)
►▼
Show Figures
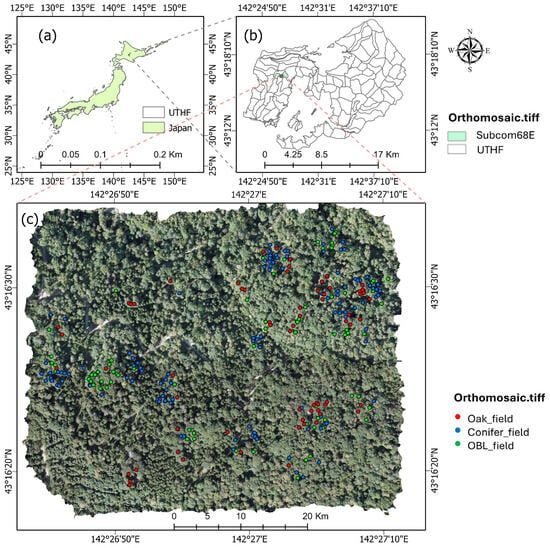
Figure 1
Open AccessArticle
Using Vegetation Indices Developed for Sentinel-2 Multispectral Data to Track Spatiotemporal Changes in the Leaf Area Index of Temperate Deciduous Forests
by
Xuanwen Wang, Yi Gan, Atsuhiro Iio and Quan Wang
Geomatics 2025, 5(1), 11; https://doi.org/10.3390/geomatics5010011 - 28 Feb 2025
Abstract
►▼
Show Figures
The leaf area index (LAI) in temperate forests is highly dynamic throughout the season, and lacking such dynamic information has limited our understanding of carbon and water flux patterns in these ecosystems. This study aims to explore the potential of using vegetation indices
[...] Read more.
The leaf area index (LAI) in temperate forests is highly dynamic throughout the season, and lacking such dynamic information has limited our understanding of carbon and water flux patterns in these ecosystems. This study aims to explore the potential of using vegetation indices based on Sentinel-2 data, which includes three additional spectral bands in the red-edge region of its multispectral imager (MSI) sensor compared to previous satellite-borne imagery, to effectively track seasonal variations in LAI within typical cold–temperate deciduous forests originating in rugged terrain in Japan. We evaluated reported vegetation indices and developed an index specific to Sentinel-2 data to effectively monitor the spatiotemporal changes of LAI in mountainous deciduous forests, providing more accurate data for ecological monitoring. Results showed that the developed index (SRB12,B7) was able to track LAI at both seasonal and spatial scales (R2 = 0.576). Further analyses revealed that the index nevertheless performed relatively poorly during the leaf-maturing season when LAI peaks, suggesting that it still suffers from a “saturation” problem. For high-resolution tracking of LAI in temperate deciduous forests at both temporal and spatial scales, future research is needed to incorporate additional information.
Full article
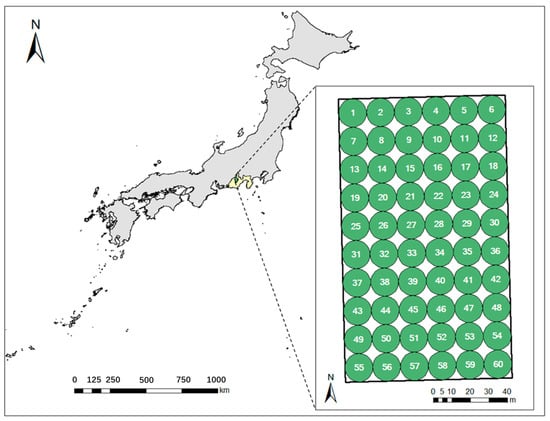
Figure 1
Open AccessArticle
Assessing Drought Severity in Greece Using Geospatial Data and Environmental Indices
by
Constantina Vasilakou, Dimitrios E. Tsesmelis, Kleomenis Kalogeropoulos, Pantelis E. Barouchas, Ilias Machairas, Elissavet G. Feloni, Andreas Tsatsaris and Christos A. Karavitis
Geomatics 2025, 5(1), 10; https://doi.org/10.3390/geomatics5010010 - 13 Feb 2025
Abstract
►▼
Show Figures
Drought represents a recurring natural event that holds notable socio-economic and environmental consequences. This research aims to analyze drought patterns in Greece by employing the standardized precipitation index (SPI) and several vegetation indices within a Geographic Information System (GIS) framework. GIS is a
[...] Read more.
Drought represents a recurring natural event that holds notable socio-economic and environmental consequences. This research aims to analyze drought patterns in Greece by employing the standardized precipitation index (SPI) and several vegetation indices within a Geographic Information System (GIS) framework. GIS is a potent tool for integrating geospatial data, encompassing climatic, topographic, and hydrological information, enabling a comprehensive assessment of drought conditions. By examining historical precipitation data, the SPI quantifies the severity and duration of drought relative to long-term average precipitation. In addition, the SPI is calculated from precipitation data from a total of 152 meteorological stations. Subsequently, geostatistical techniques are applied to generate drought maps (SPI 6- and 12-timescale) and to examine the secondary effects of drought on different land uses. Satellite data are utilized to calculate indices. This is completed using satellite data by calculating the corresponding indices such as the Enhanced Vegetation Index (EVI), Normalized Difference Vegetation Index (NDVI), and Normalized Difference Water Index (NDWI). Drought maps extracted using these methods and based on indicators and remote sensing data are useful tools for policymakers, stakeholders, and water experts. The resulting drought maps, based on the indicators and remote sensing data, serve as valuable tools for policymakers and stakeholders.
Full article
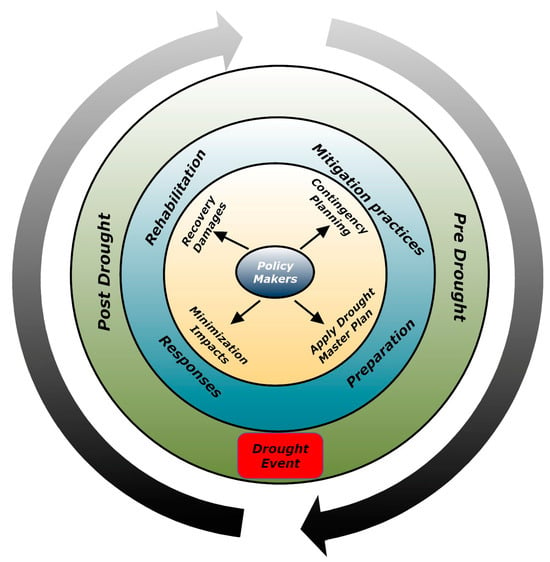
Figure 1
Open AccessReview
Advances in Remote Sensing and Deep Learning in Coastal Boundary Extraction for Erosion Monitoring
by
Marc-André Blais and Moulay A. Akhloufi
Geomatics 2025, 5(1), 9; https://doi.org/10.3390/geomatics5010009 - 6 Feb 2025
Abstract
►▼
Show Figures
Erosion is a critical geological process that degrades soil and poses significant risks to human settlements and natural habitats. As climate change intensifies, effective coastal erosion management and prevention have become essential for our society and the health of our planet. Given the
[...] Read more.
Erosion is a critical geological process that degrades soil and poses significant risks to human settlements and natural habitats. As climate change intensifies, effective coastal erosion management and prevention have become essential for our society and the health of our planet. Given the vast extent of coastal areas, erosion management efforts must prioritize the most vulnerable and critical regions. Identifying and prioritizing these areas is a complex task that requires the accurate monitoring and forecasting of erosion and its potential impacts. Various tools and techniques have been proposed to assess the risks, impacts and rates of coastal erosion. Specialized methods, such as the Coastal Vulnerability Index, have been specifically designed to evaluate the susceptibility of coastal areas to erosion. Coastal boundaries, a critical factor in coastal erosion monitoring, are typically extracted from remote sensing images. Due to the extensive scale of coastal areas and the complexity of the data, manually extracting coastal boundaries is challenging. Recently, artificial intelligence, particularly deep learning, has emerged as a promising and essential tool for this task. This review provides an in-depth analysis of remote sensing and deep learning for extracting coastal boundaries to assist in erosion monitoring. Various remote sensing imaging modalities (optical, thermal, radar), platforms (satellites, drones) and datasets are first presented to provide the context for this field. Artificial intelligence and its associated metrics are then discussed, followed by an exploration of deep learning algorithms for extracting coastal boundaries. The presented algorithms range from basic convolutional networks to encoder–decoder architectures and attention mechanisms. An overview of how these extracted boundaries and other deep learning algorithms can be utilized for monitoring coastal erosion is also provided. Finally, the current gaps, limitations and potential future directions in this field are identified. This review aims to offer critical insights into the future of erosion monitoring and management through deep learning-based boundary extraction.
Full article
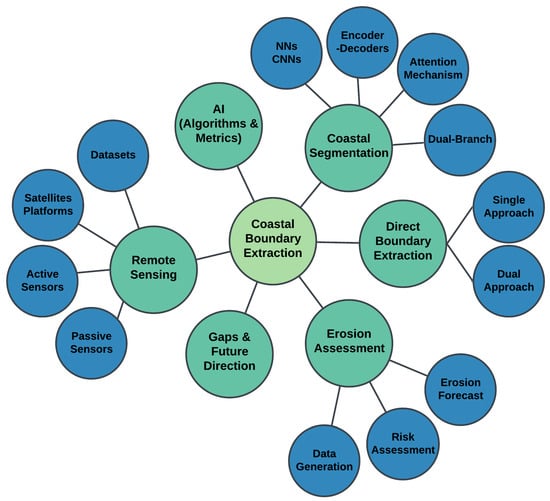
Figure 1
Open AccessArticle
Assessment of Minaret Inclination and Structural Capacity Using Terrestrial Laser Scanning and 3D Numerical Modeling: A Case Study of the Bjelave Mosque
by
Adis Hamzić, Nedim Kulo, Muamer Đidelija, Jusuf Topoljak, Admir Mulahusić, Nedim Tuno and Naida Ademović
Geomatics 2025, 5(1), 8; https://doi.org/10.3390/geomatics5010008 - 4 Feb 2025
Abstract
►▼
Show Figures
Terrestrial laser scanners (TLS) are widely employed in structural health monitoring (SHM) of large objects due to their superior capabilities compared to traditional geodetic methods. TLS provides rapid and detailed data on the geometric properties of objects, enabling various types of analyses. In
[...] Read more.
Terrestrial laser scanners (TLS) are widely employed in structural health monitoring (SHM) of large objects due to their superior capabilities compared to traditional geodetic methods. TLS provides rapid and detailed data on the geometric properties of objects, enabling various types of analyses. In this study, TLS was utilized to examine the minaret of the Bjelave Mosque, located in Sarajevo, Bosnia and Herzegovina. The inclination of the minaret was assessed using principal component analysis (PCA) and linear regression (LR) applied to sampled data from four edges of the minaret’s body. The geodetically determined inclination values were used as input data for subsequent static and pushover analyses conducted in DIANA FEA, where the minaret was modeled. The analyses indicate that the inclination increases stress and strain, leading to larger cracks and reduced structural capacity, as demonstrated by the pushover analysis curves. This study highlights the combined impact of structural inclination, water infiltration, and settlement on the minaret’s integrity and proposes these findings as a basis for future maintenance and strengthening measures.
Full article
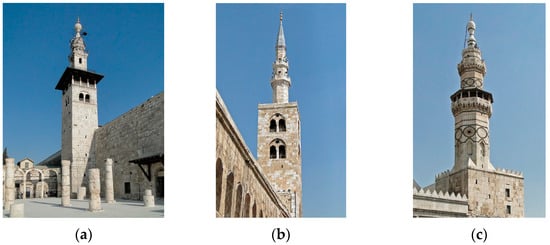
Figure 1
Open AccessArticle
Building Footprint Identification Using Remotely Sensed Images: A Compressed Sensing-Based Approach to Support Map Updating
by
Rizwan Ahmed Ansari, Rakesh Malhotra and Mohammed Zakariya Ansari
Geomatics 2025, 5(1), 7; https://doi.org/10.3390/geomatics5010007 - 31 Jan 2025
Abstract
►▼
Show Figures
Semantic segmentation of remotely sensed images for building footprint recognition has been extensively researched, and several supervised and unsupervised approaches have been presented and adopted. The capacity to do real-time mapping and precise segmentation on a significant scale while considering the intrinsic diversity
[...] Read more.
Semantic segmentation of remotely sensed images for building footprint recognition has been extensively researched, and several supervised and unsupervised approaches have been presented and adopted. The capacity to do real-time mapping and precise segmentation on a significant scale while considering the intrinsic diversity of the urban landscape in remotely sensed data has significant consequences. This study presents a novel approach for delineating building footprints by utilizing the compressed sensing and radial basis function technique. At the feature extraction stage, a small set of random features of the built-up areas is extracted from local image windows. The random features are used to train a radial basis neural network to perform building classification; thus, learning and classification are carried out in the compressed sensing domain. By virtue of its ability to represent characteristics in a reduced dimensional space, the scheme shows promise in being robust in the face of variability inherent in urban remotely sensed images. Through a comparison of the proposed method with numerous state-of-the-art approaches utilizing remotely sensed data of different spatial resolutions and building clutter, we establish its robustness and prove its viability. Accuracy assessment is performed for segmented footprints, and comparative analysis is carried out in terms of intersection over union, overall accuracy, precision, recall, and F1 score. The proposed method achieved scores of 93% in overall accuracy, 90.4% in intersection over union, and 91.1% in F1 score, even when dealing with drastically different image features. The results demonstrate that the proposed methodology yields substantial enhancements in classification accuracy and decreases in feature dimensionality.
Full article
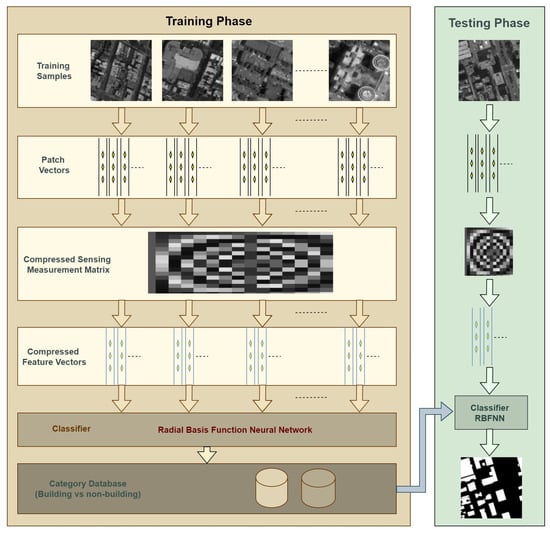
Figure 1
Open AccessArticle
Analyzing Decadal Trends of Vegetation Cover in Djibouti Using Landsat and Open Data Cube
by
Julee Wardle and Zachary Phillips
Geomatics 2025, 5(1), 6; https://doi.org/10.3390/geomatics5010006 - 30 Jan 2025
Abstract
This study investigates decadal trends in vegetation cover in Djibouti from 1990 to 2020, addressing challenges related to its arid climate and limited resources. Using Digital Earth Africa’s Open Data Cube and thirty years of Landsat imagery, change detection algorithms, and statistical analysis,
[...] Read more.
This study investigates decadal trends in vegetation cover in Djibouti from 1990 to 2020, addressing challenges related to its arid climate and limited resources. Using Digital Earth Africa’s Open Data Cube and thirty years of Landsat imagery, change detection algorithms, and statistical analysis, this research explores vegetation dynamics at various spatial and temporal scales. Studies on change detection have advanced the field through exploring Landsat time series and diverse algorithms, but face limitations in handling data inconsistencies, integrating methods, and addressing practical and socio-environmental challenges. The results, obtained through change detection using NDVI differencing and Welch’s t-test, reveal significant trends in vegetation across Djibouti’s administrative and countrywide levels. Results show significant countrywide vegetative loss from 1990 to 2010, but recovery from 2010 to 2020, as evidenced by Welch’s t-test results. This disproved the Null Hypothesis of no trend and confirmed significant trends across all regions and resolutions analyzed. The findings provide important information for policymakers, land managers, and conservationists, providing awareness into patterns of Djibouti’s vegetation trends in the face of future climate change. The use of Open Data Cube and cloud computing enhances research capacity, allowing for the rapid and repeated analysis of larger time periods and geographical regions.
Full article
(This article belongs to the Topic Remote Sensing and Geoinformatics in Agriculture and Environment, 2nd Edition)
►▼
Show Figures
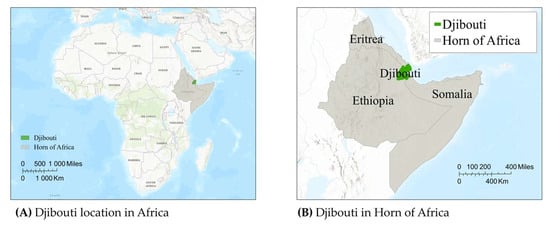
Figure 1
Open AccessArticle
Improving Bimonthly Landscape Monitoring in Morocco, North Africa, by Integrating Machine Learning with GRASS GIS
by
Polina Lemenkova
Geomatics 2025, 5(1), 5; https://doi.org/10.3390/geomatics5010005 - 20 Jan 2025
Abstract
►▼
Show Figures
This article presents the application of novel cartographic methods of vegetation mapping with a case study of the Rif Mountains, northern Morocco. The study area is notable for varied geomorphology and diverse landscapes. The methodology includes ML modules of GRASS GIS ‘r.learn.train’, ‘r.learn.predict’,
[...] Read more.
This article presents the application of novel cartographic methods of vegetation mapping with a case study of the Rif Mountains, northern Morocco. The study area is notable for varied geomorphology and diverse landscapes. The methodology includes ML modules of GRASS GIS ‘r.learn.train’, ‘r.learn.predict’, and ‘r.random’ with algorithms of supervised classification implemented from the Scikit-Learn libraries of Python. This approach provides a platform for processing spatiotemporal data and satellite image analysis. The objective is to determine the robustness of the “DecisionTreeClassifier” and “ExtraTreesClassifier” classification algorithms. The time series of satellite images covering northern Morocco consists of six Landsat scenes for 2023 with a bimonthly time interval. Land cover maps are produced based on the processed, classified, and analyzed images. The results demonstrated seasonal changes in vegetation and land cover types. The validation was performed using a land cover dataset from the Food and Agriculture Organization (FAO). This study contributes to environmental monitoring in North Africa using ML algorithms of satellite image processing. Using RS data combined with the powerful functionality of the GRASS GIS and FAO-derived datasets, the topographic variability, moderate-scale habitat heterogeneity, and bimonthly distribution of land cover types of northern Morocco in 2023 have been assessed for the first time.
Full article
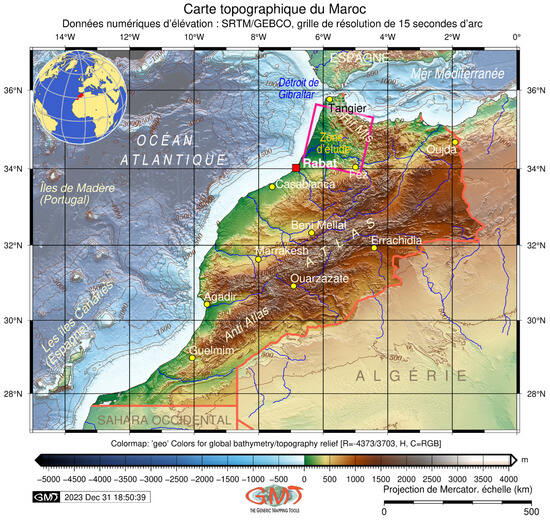
Figure 1
Open AccessArticle
Examining Deep Learning Pixel-Based Classification Algorithms for Mapping Weed Canopy Cover in Wheat Production Using Drone Data
by
Judith N. Oppong, Clement E. Akumu, Samuel Dennis and Stephanie Anyanwu
Geomatics 2025, 5(1), 4; https://doi.org/10.3390/geomatics5010004 - 10 Jan 2025
Abstract
Deep learning models offer valuable insights by leveraging large datasets, enabling precise and strategic decision-making essential for modern agriculture. Despite their potential, limited research has focused on the performance of pixel-based deep learning algorithms for detecting and mapping weed canopy cover. This study
[...] Read more.
Deep learning models offer valuable insights by leveraging large datasets, enabling precise and strategic decision-making essential for modern agriculture. Despite their potential, limited research has focused on the performance of pixel-based deep learning algorithms for detecting and mapping weed canopy cover. This study aims to evaluate the effectiveness of three neural network architectures—U-Net, DeepLabV3 (DLV3), and pyramid scene parsing network (PSPNet)—in mapping weed canopy cover in winter wheat. Drone data collected at the jointing and booting growth stages of winter wheat were used for the analysis. A supervised deep learning pixel classification methodology was adopted, and the models were tested on broadleaved weed species, winter wheat, and other weed species. The results show that PSPNet outperformed both U-Net and DLV3 in classification performance, with PSPNet achieving the highest overall mapping accuracy of 80%, followed by U-Net at 75% and DLV3 at 56.5%. These findings highlight the potential of pixel-based deep learning algorithms to enhance weed canopy mapping, enabling farmers to make more informed, site-specific weed management decisions, ultimately improving production and promoting sustainable agricultural practices.
Full article
(This article belongs to the Topic Geographic Information and Remote Sensing Technology (GIRST))
►▼
Show Figures
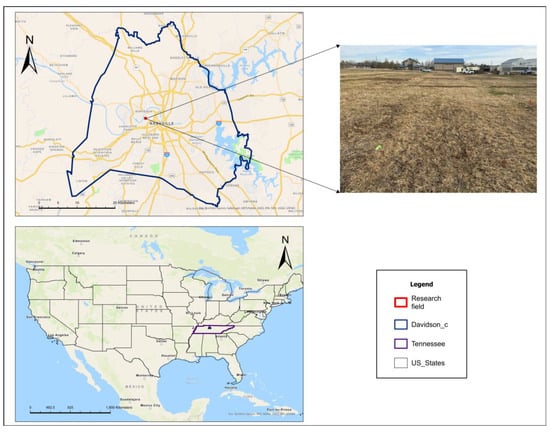
Figure 1
Open AccessArticle
Mapping Spatial Variability of Sugarcane Foliar Nitrogen, Phosphorus, Potassium and Chlorophyll Concentrations Using Remote Sensing
by
Ericka F. Picado, Kerin F. Romero and Muditha K. Heenkenda
Geomatics 2025, 5(1), 3; https://doi.org/10.3390/geomatics5010003 - 5 Jan 2025
Abstract
►▼
Show Figures
Various nutrients are needed during the sugarcane growing season for plant development and productivity. However, traditional methods for assessing nutritional status are often costly and time consuming. This study aimed to determine the level of nitrogen (N), phosphorus (P), potassium (K) and chlorophyll
[...] Read more.
Various nutrients are needed during the sugarcane growing season for plant development and productivity. However, traditional methods for assessing nutritional status are often costly and time consuming. This study aimed to determine the level of nitrogen (N), phosphorus (P), potassium (K) and chlorophyll of sugarcane plants using remote sensing. Remotely sensed images were obtained using a MicaSense RedEdge-P camera attached to a drone. Leaf chlorophyll content was measured in the field using an N-Tester chlorophyll meter, and leaf samples were collected and analyzed in the laboratory for N, P and K. The highest correlation between field samples and predictor variables (spectral bands, selected vegetation indices, and plant height from Light Detection and Ranging (LiDAR)), were noted.The spatial distribution of chlorophyll, N, P, and K maps achieved 60%, 75%, 96% and 50% accuracies, respectively. The spectral profiles helped to identify areas with visual differences. Spatial variability of nutrient maps confirmed that moisture presence leads to nitrogen and potassium deficiencies, excess phosphorus, and a reduction in vegetation density (93.82%) and height (2.09 m), compared to green, healthy vegetation (97.64% density and 3.11 m in height). This robust method of assessing foliar nutrients is repeatable for the same sugarcane variety at certain conditions and leads to sustainable agricultural practices in Costa Rica.
Full article
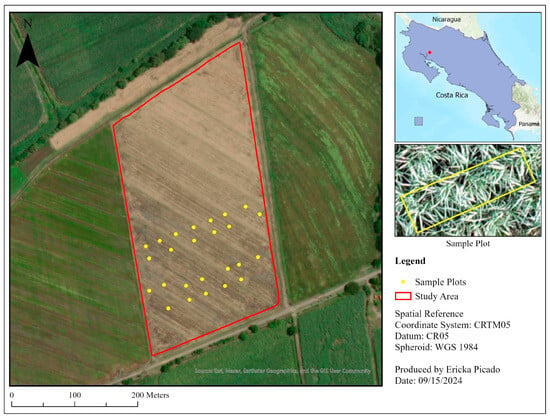
Figure 1
Open AccessArticle
Lessons Learned from the LBS2ITS Project—An Interdisciplinary Approach for Curricula Development in Geomatics Education
by
Günther Retscher, Jelena Gabela and Vassilis Gikas
Geomatics 2025, 5(1), 2; https://doi.org/10.3390/geomatics5010002 - 30 Dec 2024
Abstract
►▼
Show Figures
The LBS2ITS project, titled “Curricula Enrichment Delivered through the Application of Location-Based Services to Intelligent Transport Systems”, is a collaborative initiative funded by the Erasmus+ program of the European Union. The primary objectives of the project were twofold: to develop new curricula and
[...] Read more.
The LBS2ITS project, titled “Curricula Enrichment Delivered through the Application of Location-Based Services to Intelligent Transport Systems”, is a collaborative initiative funded by the Erasmus+ program of the European Union. The primary objectives of the project were twofold: to develop new curricula and modernize existing programs at four universities in Sri Lanka. This effort was driven by the need to align educational offerings with the rapidly evolving fields of Location-Based Services (LBSs) and Intelligent Transport Systems (ITSs). A key feature of the LBS2ITS project is its interdisciplinary approach, which draws on expertise from a range of academic disciplines. The project has successfully developed curricula that integrate diverse fields such as geomatics, cartography, transport engineering, urban planning, environmental engineering, and computer science. By blending these perspectives, the curricula provide students with a holistic understanding of LBSs and ITSs, preparing them to address complex, real-world challenges that span multiple sectors. In this paper, the curriculum development and modernization process is detailed, with a particular focus on the two key phases: teacher training and curriculum development. The teacher training phase was crucial in equipping educators with the skills and knowledge necessary to deliver the new and updated courses. This phase also provided an opportunity for teachers to familiarize themselves with the latest trends and technologies in LBSs and ITSs, ensuring that they could effectively convey this information to students. The development phase focused on the creation of the curriculum itself, ensuring that it met both academic standards and industry needs. The curriculum was designed to be flexible and responsive to emerging technologies and methodologies, allowing for continuous improvement and adaptation. Additionally, the paper delves into the theoretical frameworks underpinning the methodologies employed in the project. These include Problem-Based Learning (PBL) and Problem-Based e-Learning (PBeL), both of which encourage active student engagement and foster critical thinking by having students tackle real-world problems. The emphasis on PBL ensures that students not only acquire theoretical knowledge but also develop practical problem-solving skills applicable to their future careers in LBSs and ITSs. Furthermore, the project incorporated rigorous quality assurance (QA) mechanisms to ensure that the teaching methods and curriculum content met high standards. This included regular feedback loops, stakeholder involvement, and iterative refinement of course materials based on evaluations from both students and industry experts. These QA measures are essential for maintaining the relevance, effectiveness, and sustainability of the curricula over time. In summary, the LBS2ITS project represents a significant effort to enrich and modernize university curricula in Sri Lanka by integrating cutting-edge technologies and interdisciplinary approaches. Through a combination of innovative teaching methodologies, comprehensive teacher training, and robust quality assurance practices, the project aims to equip students with the skills and knowledge needed to excel in the fields of LBSs and ITSs.
Full article
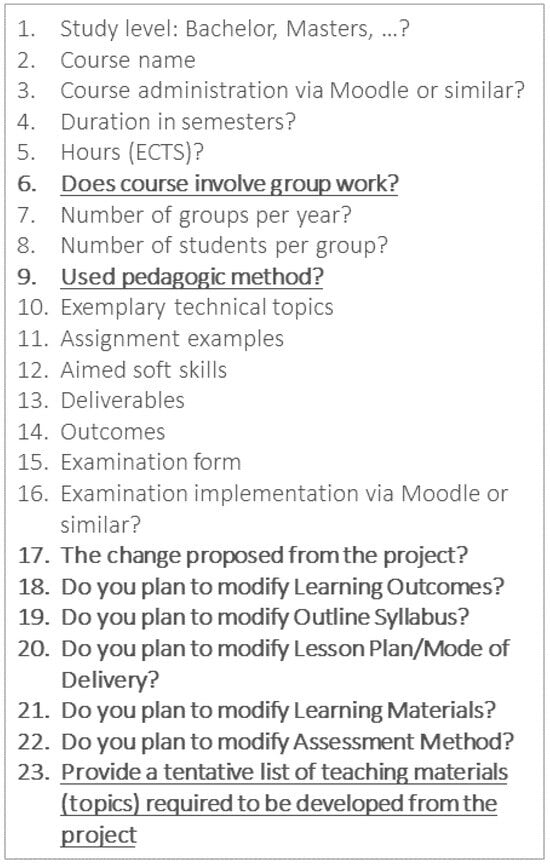
Figure 1
Open AccessArticle
Relationship Between Lithological and Morphometric Aspects of Mascasín Saline Watershed and Its Feeder Depositional Systems, San Juan and La Rioja Provinces, Argentina
by
Paula Santi Malnis and Luis Martin Rothis
Geomatics 2025, 5(1), 1; https://doi.org/10.3390/geomatics5010001 - 30 Dec 2024
Abstract
►▼
Show Figures
Understanding the relationships among watersheds and derived depositional products is critical to developing analog studies with the rock record, especially for continental intermontane basins. Also, it is crucial to study river flood occurrences. Multivariate statistics analysis allows for the comprehension of the relationship
[...] Read more.
Understanding the relationships among watersheds and derived depositional products is critical to developing analog studies with the rock record, especially for continental intermontane basins. Also, it is crucial to study river flood occurrences. Multivariate statistics analysis allows for the comprehension of the relationship among substrate, climate, and depositional products of the watersheds that feed the endorheic Mascasin Saline Basin, San Juan and La Rioja provinces, Argentina. Using a GIS platform, geomorphological, stratigraphic, morphometric, and structural analysis gave a dataset of variables for defining clusters. Under a similar climate, clustering analysis permits defining two main controls on watersheds and depositional products: parent rock composition and geological structures (faults and lineaments). The results underscore the critical role that lithology and structural controls play in basin morphometry and emphasize the need to quantify these variables for landscape evolution models.
Full article
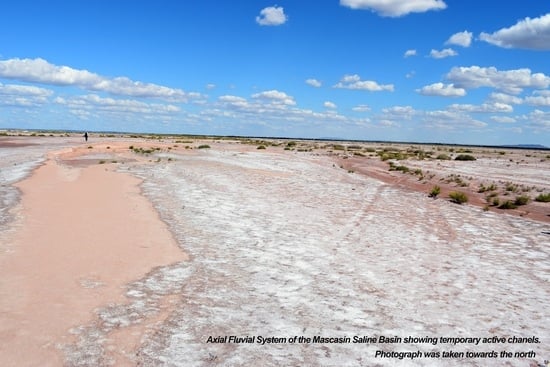
Graphical abstract
Open AccessEditorial
Advancements in Ocean Mapping and Nautical Cartography
by
Giuseppe Masetti, Ian Church, Anand Hiroji and Ove Andersen
Geomatics 2024, 4(4), 433-436; https://doi.org/10.3390/geomatics4040023 - 28 Nov 2024
Abstract
Ocean mapping and nautical cartography are foundational to understanding and managing marine environments [...]
Full article
(This article belongs to the Special Issue Advances in Ocean Mapping and Nautical Cartography)
Open AccessArticle
Deep Learning for Urban Tree Canopy Coverage Analysis: A Comparison and Case Study
by
Grayson R. Morgan, Danny Zlotnick, Luke North, Cade Smith and Lane Stevenson
Geomatics 2024, 4(4), 412-432; https://doi.org/10.3390/geomatics4040022 - 14 Nov 2024
Abstract
Urban tree canopy (UTC) coverage, or area, is an important metric for monitoring changes in UTC over large areas within a municipality. Several methods have been used to obtain these data, but remote sensing image classification is one of the fastest and most
[...] Read more.
Urban tree canopy (UTC) coverage, or area, is an important metric for monitoring changes in UTC over large areas within a municipality. Several methods have been used to obtain these data, but remote sensing image classification is one of the fastest and most reliable over large areas. However, most studies have tested only one or two classification methods to accomplish this while using costly satellite imagery or LiDAR data. This study seeks to compare three urban tree canopy cover classifiers by testing a deep learning U-Net convolutional neural network (CNN), support vector machine learning classifier (SVM) and a random forests machine learning classifier (RF) on cost-free 2012 aerial imagery over a small southern USA city and midsize, growing southern USA city. The results of the experiment are then used to decide the best classifier and apply it to more recent aerial imagery to determine canopy changes over a 10-year period. The changes are subsequently compared visually and statistically with recent urban heat maps derived from thermal Landsat 9 satellite data to compare the means of temperatures within areas of UTC loss and no change. The U-Net CNN classifier proved to provide the best overall accuracy for both cities (89.8% and 91.4%), while also requiring the most training and classification time. When compared spatially with city heat maps, city periphery regions were most impacted by substantial changes in UTC area as cities grow and the outer regions get warmer. Furthermore, areas of UTC loss had higher temperatures than those areas with no canopy change. The broader impacts of this study reach the urban forestry managers at the local, state/province, and national levels as they seek to provide data-driven decisions for policy makers.
Full article
(This article belongs to the Topic Geocomputation and Artificial Intelligence for Mapping)
►▼
Show Figures
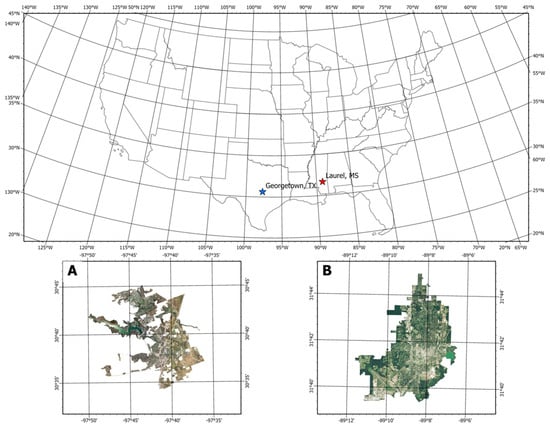
Figure 1
Open AccessArticle
Application of GIS in Introducing Community-Based Biogas Plants from Dairy Farm Waste: Potential of Renewable Energy for Rural Areas in Bangladesh
by
Kohinur Aktar, Helmut Yabar, Takeshi Mizunoya and Md. Monirul Islam
Geomatics 2024, 4(4), 384-411; https://doi.org/10.3390/geomatics4040021 - 6 Nov 2024
Abstract
►▼
Show Figures
Dairy production is one of the most important economic sectors in Bangladesh. However, the traditional management of dairy cow manure and other wastes results in air pollution, eutrophication of surface water, and soil contamination, highlighting the urgent need for more sustainable waste management
[...] Read more.
Dairy production is one of the most important economic sectors in Bangladesh. However, the traditional management of dairy cow manure and other wastes results in air pollution, eutrophication of surface water, and soil contamination, highlighting the urgent need for more sustainable waste management solutions. To address the environmental problems of dairy waste management, this research explored the potential of community-based biogas production from dairy cow manure in Bangladesh. This study proposed introducing community-based biogas plants using a geographic information system (GIS). The study first applied a restriction analysis to identify sensitive areas, followed by a suitability analysis to determine feasible locations for biogas plants, considering geographical, social, economic, and environmental factors. The final suitable areas were identified by combining the restriction and suitability maps. The spatial distribution of dairy farms was analyzed through a cluster analysis, identifying significant clusters for potential biogas production. A baseline and proposed scenario were designed for five clusters based on the input and output capacities of the biogas plants, estimating the location and capacity for each cluster. The study also calculated electricity generation from the proposed scenario and the net greenhouse gas (GHG) emissions reduction potential of the biogas plants. The findings provide a land-use framework for implementing biogas plants that considers environmental and socio-economic criteria. Five biogas plants were found to be technically and spatially feasible for electricity generation. These plants can collectively produce 31 million m3 of biogas annually, generating approximately 200.60 GWh of energy with a total electricity capacity of 9.8 MW/year in Bangladesh. Implementing these biogas plants is expected to increase renewable energy production by at least 1.25%. Furthermore, the total GHG emission reduction potential is estimated at 104.26 Gg/year CO2eq through the annual treatment of 61.38 thousand tons of dairy manure.
Full article
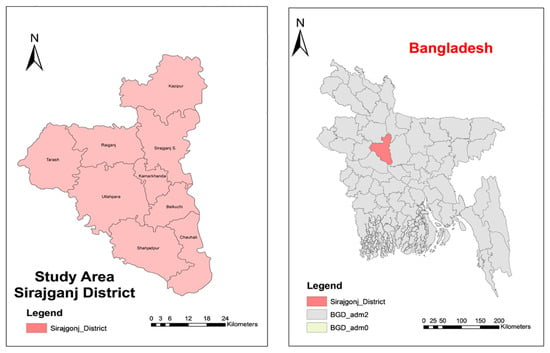
Figure 1
Open AccessEditorial
Advancing Geomatics: Innovation, Inclusivity, and Global Perspectives
by
Christophe Claramunt
Geomatics 2024, 4(4), 382-383; https://doi.org/10.3390/geomatics4040020 - 5 Oct 2024
Abstract
In the past few years since its launch, Geomatics has addressed various areas that form the core of the interdisciplinary field of geomatics [...]
Full article
Open AccessArticle
Spatiotemporal Analysis of Land Use and Land Cover Dynamics of Dinderesso and Peni Forests in Burkina Faso
by
Alphonse Maré David Millogo, Boalidioa Tankoano, Oblé Neya, Fousseni Folega, Kperkouma Wala, Kwame Oppong Hackman, Bernadin Namoano and Komlan Batawila
Geomatics 2024, 4(4), 362-381; https://doi.org/10.3390/geomatics4040019 - 4 Oct 2024
Cited by 1
Abstract
►▼
Show Figures
The sustainable management of protected areas has increasingly become difficult due to the lack of updated information on land use and land cover transformations caused by anthropogenic pressures. This study investigates the spatiotemporal dynamics of the Dinderesso and Peni classified forests in Burkina
[...] Read more.
The sustainable management of protected areas has increasingly become difficult due to the lack of updated information on land use and land cover transformations caused by anthropogenic pressures. This study investigates the spatiotemporal dynamics of the Dinderesso and Peni classified forests in Burkina Faso from 1986 to 2022. First, a data driven method was adopted to investigate these forests degradation dynamics. Hence, relevant Landsat images data were collected, segmented, and analyzed using QGIS SCP plugin Random Forest algorithm. Ninety percent of the overall adjusted classification accuracies were obtained. The analysis also showed significant degradation and deforestation with high wooded vegetation classes such as clear forest and wooded savannah (i.e., tree savannah) converging to lower vegetation classes like shrub savannah and agroforestry parks. A second investigation carried out through surveys and field trips revealed key anthropogenic drivers including agricultural expansion, demographic pressure, bad management, wood cutting abuse, overexploitation, overgrazing, charcoal production, and bushfires. These findings highlight the critical need for better management to improve these protected areas.
Full article
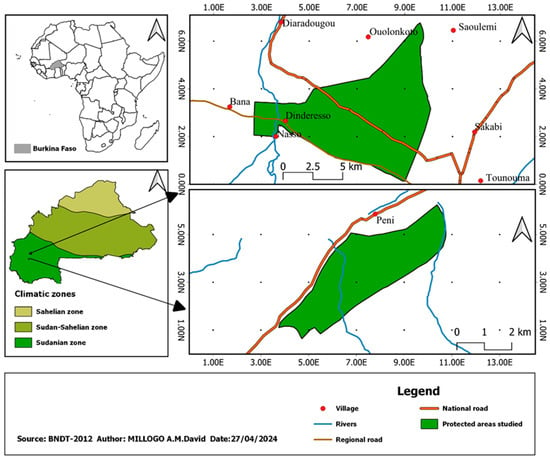
Figure 1
Highly Accessed Articles
Latest Books
E-Mail Alert
News
Topics
Topic in
Remote Sensing, Sensors, Smart Cities, Vehicles, Geomatics
Information Sensing Technology for Intelligent/Driverless Vehicle, 2nd Edition
Topic Editors: Yan Huang, Yi Ren, Penghui Huang, Jun Wan, Zhanye Chen, Shiyang TangDeadline: 31 May 2025
Topic in
Electronics, Geomatics, Remote Sensing, Sensors, Smart Cities, Technologies
Smartphone Positioning, Navigation and Timing: Advances and Challenges
Topic Editors: Vincenzo Capuano, Fabio DovisDeadline: 31 August 2025
Topic in
Forests, Geomatics, Remote Sensing, Sensors
Vegetation Characterization and Classification With Multi-Source Remote Sensing Data
Topic Editors: Baoxin Hu, Linhai JingDeadline: 30 September 2025
Topic in
Geosciences, Minerals, Geomatics
Future Trends in Mapping Potential Zones of Critical Minerals Using Advanced Imagery Techniques
Topic Editors: Amin Beiranvand Pour, Mazlan Hashim, Shojaeddin Niroomand, Basem Zoheir, Jong Kuk Hong, Hojjatollah RanjbarDeadline: 31 October 2025

Conferences
Special Issues
Special Issue in
Geomatics
Advances in Ocean Mapping and Hydrospatial Applications
Guest Editors: Anand Hiroji, Ian Church, Giuseppe MasettiDeadline: 31 July 2025
Special Issue in
Geomatics
Open-Source Geoinformation Software Tools in Environmental Modelling
Guest Editors: George P. Petropoulos, Prashant Srivastava, Daniela Silva-FuzzoDeadline: 31 July 2025
Special Issue in
Geomatics
The Role of Remote Sensing and GIS in Mapping the Earth’s 2D and 3D Geometry
Guest Editors: Mehmet Alkan, Elisa Mariarosaria Farella, Ismail ColkesenDeadline: 15 October 2025
Special Issue in
Geomatics
The State-of-the-Art of Critical Infrastructures (CI) Monitoring/Protection
Guest Editors: Salvatore Stramondo, Maurizio PollinoDeadline: 31 October 2025