Metabolomics in Hyperuricemia and Gout
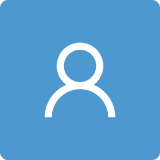
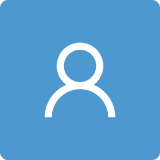
Round 1
Reviewer 1 Report
The current review describes metabolomic techniques and how they can be applied to gout and hyperuricemia. The review is well-written, and the text flows easily. Most of the content is complete and up to date. And the last section of the review offers novel insights and future directions. However, the authors may want to consider some minor suggestions to improve the quality of the review. 1) Introduction- Line 35. Be more specific about which kind of treatment of the inflammation is being referred to.
- Line 45. Perhaps delete the word "genome" since -to my knowledge- there is no strong evidence for changes in the genomic sequence by metabolites. If the authors think that there is solid evidence, please add references.
- Line 46. In the references provided, please add Boon et al., 2020 (PubMed ID: 33046909).
3) Metabolomics data analysis, interpretation, and sharing The authors mention the importance of sharing data and uploading experiment results to repositories. Among the data repositories publicly available, the authors can add GNPS (https://gnps.ucsd.edu/ProteoSAFe/static/gnps-splash.jsp) from University California, San Diego. 6) Metabolomics in experimental models The authors may want to include two recent papers on the effect of metabolic mediators in regulating inflammation during damage induced by MSUc (Renauding et al., 2020, PubMed ID: 32699039; and Cobo et al., 2022, PubMed ID: 35263587). Alternatively, the authors can add these two references in the Summary and future directions around Line 220. 7) Summary and future directions There is a typo in Line 238.
Author Response
Dear reviewer,
We appreciate your helpful comments and suggestion to improve the manuscript. Please see the point-to-point responses below:
Line 35. Be more specific about which kind of treatment of the inflammation is being referred to.
We changed the sentence to: "Urate lowering therapy (ULT) for HU or treatment of the inflammation by colchicine or non-steroidal anti-inflammatory drugs (NSAIDs) is the key in clinical gout management".
Line 45. Perhaps delete the word "genome" since -to my knowledge- there is no strong evidence for changes in the genomic sequence by metabolites. If the authors think that there is solid evidence, please add references.
We deleted the word "genome".
Line 46. In the references provided, please add Boon et al., 2020 (PubMed ID: 33046909).
Perhaps the authors may want to consider adding a reference to the effect of Histone lactylation during the response of Macrophages to various stimuli (Zhang et al., 2019; PubMed ID: 31645732). Histone lactylation is an excellent example of the effect of a metabolite in altering the epigenome and downstream gene expression.
We added the above references.
2) Analytical technology for metabolomics
The authors may want to consider adding some comments on the different types of columns used for targeted metabolomics in LC-MSpec. In addition, it is worth explaining that, when using MSpec, there are better-detected metabolites in Phase + vs Phase -. And to give some examples of metabolites that are often detected in one Phase but not in the other.
We added the comments on columns and Phase + (positive ion mode) and Phase – (negative ion mode): " The columns used for LC separations can affect metabolomics analysis. For example, the LC columns based on hydrophilic interaction chromatography (HILIC) or C18 are widely used to separate the aqueous metabolites, such as sugars [1, 2], while the C8 column is good for separation of less polar metabolites, such as free fatty acids [3] [4]. Besides, the ionization mode, either in the positive or negative ion mode, may impact the coverage of metabolites as well. In general, basic metabolites are readily detected in the positive ion mode, whereas acidic compounds, such as fatty acids, are amenable for ionization in negative ion mode. Thus, to improve the coverage of metabolomics analysis, both positive ionization and negative ionization are performed, either in separate acquisition or switching between both mode in one method[1]. "
3) Metabolomics data analysis, interpretation, and sharing
The authors mention the importance of sharing data and uploading experiment results to repositories. Among the data repositories publicly available, the authors can add GNPS (https://gnps.ucsd.edu/ProteoSAFe/static/gnps-splash.jsp) from University California, San Diego.
We added the GNPS in the article: "…such as the Human Metabolome Database (HMDB)[5], MassBank[6], GNPS[7], LipidBlast[8] and METLIN[9]… "
6) Metabolomics in experimental models
The authors may want to include two recent papers on the effect of metabolic mediators in regulating inflammation during damage induced by MSUc (Renauding et al., 2020, PubMed ID: 32699039; and Cobo et al., 2022, PubMed ID: 35263587). Alternatively, the authors can add these two references in the Summary and future directions around Line 220.
We changed the paragraph in Line 220 (old version) and added the above references to Lines 50~53 in the new version.
7) Summary and future directions There is a typo in Line 238.
We corrected the typo in line 238.
References
- Shen, X., et al., Serum metabolomics identifies dysregulated pathways and potential metabolic biomarkers for hyperuricemia and gout. Arthritis Rheumatol, 2021.
- Zhang, Z., et al., Serine catabolism generates liver NADPH and supports hepatic lipogenesis. Nat Metab, 2021. 3(12): p. 1608-1620.
- Hui, S., et al., Glucose feeds the TCA cycle via circulating lactate. Nature, 2017. 551(7678): p. 115-118.
- Li, M., et al., Aldolase B suppresses hepatocellular carcinogenesis by inhibiting G6PD and pentose phosphate pathways. Nat Cancer, 2020. 1(7): p. 735-747.
- Wishart, D.S., et al., HMDB 5.0: the Human Metabolome Database for 2022. Nucleic Acids Research, 2022. 50(D1): p. D622-D631.
- Horai, H., et al., MassBank: a public repository for sharing mass spectral data for life sciences. Journal of Mass Spectrometry, 2010. 45(7): p. 703-714.
- Wang, M., et al., Sharing and community curation of mass spectrometry data with Global Natural Products Social Molecular Networking. Nat Biotechnol, 2016. 34(8): p. 828-837.
- Kind, T., et al., LipidBlast in silico tandem mass spectrometry database for lipid identification. Nature Methods, 2013. 10(8): p. 755-758.
- Montenegro-Burke, J.R., C. Guijas, and G. Siuzdak, METLIN: A Tandem Mass Spectral Library of Standards. 2020, Springer US. p. 149-163.
Reviewer 2 Report
Dear Authors,
Thank you for putting together the review for Gout metabolomics. I appreciate the effort you have invested in going through the literature and provide your expert connection and interpretation. You have introduce concisely the background of Gout biology, metabolomics platform and how both areas intersect.
On page 1, line 45: the authors stated that metabolites "... also impact genome,...". This could be misunderstood as metabolites can change the genome sequence instead of only interacting with it (epigenetics), effecting transcription (RNA levels) and transcription (protein expression). I suggest the use of "... also interact with ..." to avoid misinterpretation.
I was hoping to see more synthesis of data from individual studies and put together for a more wholistic view of Gout metabolomics. Perhaps another figure illustrate components of metabolites in pathways and cellular processes leading to disease would connect the data points from each publications in the review?
The last paragraph, for instance, was rather high-level that did not seem to add additional insights into the remainder of the manuscript and may read better if introduced earlier and more actionable details are added.
Thanks again for preparing the manuscript reviewing metabolomics in Gout. I hope my feedback was helpful in your revision and wish you all the best in getting the work published.
Author Response
Dear reviewer,
We appreciate your helpful comments and please see below for our point-to-point responses:
On page 1, line 45: the authors stated that metabolites "... also impact genome,...". This could be misunderstood as metabolites can change the genome sequence instead of only interacting with it (epigenetics), affecting transcription (RNA levels) and transcription (protein expression). I suggest the use of "... also interact with ..." to avoid misinterpretation.
We deleted the word "genome", and changed the sentence to: "... also interact with ..."
I was hoping to see more synthesis of data from individual studies and put together for a more wholistic view of Gout metabolomics. Perhaps another figure illustrate components of metabolites in pathways and cellular processes leading to disease would connect the data points from each publications in the review?
We completely agree with the reviewer in this regard. However, it is quite difficult to synthesize the published metabolomic data to give a wholistic review due to lack of standardized study protocol. It remains a tremendous challenge to replicate the metabolomic data in separate studies. Nonetheless, we added some metabolites info in Table 1 and integrated previously reported biomarkers in new Figure 2.
The last paragraph, for instance, was rather high-level that did not seem to add additional insights into the remainder of the manuscript and may read better if introduced earlier and more actionable details are added.
We agree with you and moved some sentences in the last paragraph to the introduction, which might read better.
Reviewer 3 Report
This is a timely review that summarizes the current knowledge on differentially abundant metabolites and their biomarker potential in gout and asymptomatic hyperuricemia. This review integrates recent and very recent metabolomic studies in humans and rodents and spells out the need for further work in this direction + multi-omics data integration in gout and hyperuricemia. Some issues would help the reader to navigate the paper more easily:
1. Compared to the extensive description of metabolomic approaches (section 2 and 3), section 4 could benefit from a more elaborate discussion on the discovered metabolites that may be relevant in gout and HU and speculation on causes and mechanisms of these metabolome changes. Would it be possible for the authors to outline how these metabolites may become differentially abundant in these clinical situations (ex. purine synthesis pathway, dietary implications, other hypotheses) and via which mechanism this may contribute to gout, inflammatory consequences, etc.
2. A visual representation of the metabolic pathways identified in these studies would be extremely useful for the reader to get an overview of the pathways that are modulated and how the different metabolic branches integrate. Stressing out the metabolite changes that are supported by several reports is encouraged.
3. In table 1, it would be useful to have an indication of whether the metabolite biomarkers and “alterations in metabolism” are upregulated or downregulated.
4. In section 7, a discussion on sample sizes and reproducibility of study findings would be good. What is the opinion of authors about sample sizes in future metabolomic studies and potentially multi-omics data integration with respect to risk of type 1 or type 2 statistical errors?
5. Since gout and hyperuricemia are often associated to other comorbidities, a discussion about potential patient variability (or not) in the original studies would be useful (ex. are there any covariates that have been or have not been considered in the original studies).
Minor points:
Rechecking the text may be useful: e.g., line 15 (In?) “Recent years”; line 20 “systematic metabolism”(?); line 110 “statistically significant (differentially abundant?) metabolites”; line 130 “data sharing and re-analyze” (re-analysis?); line 146 “observed in urine and fecal (samples?) from gout patients”; line 149 “threonine metabolism and some of lipid” (unclear); line 177 “consistent with metabolomic data”; line180 “a combination of microbiome and metabolome analysis …consider moving here the ending part of the sentence “in feces from gout patients”; line 2016 – consider not referring to HUA as “common metabolic disease”; line 242 “have yet been (to be?) established”, etc.
Some abbreviations are not written in full at first use: HUA, GA, ALDH16A1, PC, lysoPC, etc.
Author Response
Dear reviewer,
We appreciate your helpful comments and please see our point-to-point responses.
- Compared to the extensive description of metabolomic approaches (section 2 and 3), section 4 could benefit from a more elaborate discussion on the discovered metabolites that may be relevant in gout and HU and speculation on causes and mechanisms of these metabolome changes. Would it be possible for the authors to outline how these metabolites may become differentially abundant in these clinical situations (ex. purine synthesis pathway, dietary implications, other hypotheses) and via which mechanism this may contribute to gout, inflammatory consequences, etc.
Based on your suggestion, we updated Table 1 and added Figure 2 by integrating previously reported metabolite biomarkers in metabolomic studies.
- A visual representation of the metabolic pathways identified in these studies would be extremely useful for the reader to get an overview of the pathways that are modulated and how the different metabolic branches integrate. Stressing out the metabolite changes that are supported by several reports is encouraged.
We added Figure 2 to illustrate the metabolic biomarkers in published metabolomics studies in hyperuricemia and gout.
- In table 1, it would be useful to have an indication of whether the metabolite biomarkers and “alterations in metabolism” are upregulated or downregulated.
Thanks for this suggestion and we updated Table 1 by specifically indicating the metabolites are upregulated or down regulated. In addition, we also revised the text accordingly.
- In section 7, a discussion on sample sizes and reproducibility of study findings would be good. What is the opinion of authors about sample sizes in future metabolomic studies and potentially multi-omics data integration with respect to risk of type 1 or type 2 statistical errors?
We appreciated your comments and added more discussions in Lines 268~273 " In clinical metabolomic studies, sample size, demographic characteristics and clinical parameters, are key factors for identifying reproducible metabolites and pathways associated with metabolic diseases, including hyperuricemia and gout. Furthermore, statistics are important for omics analysis, and covariates should be considered and strict multiple hypothesis tests should be performed to minimize the risk of type 1 statistical errors. "
- Since gout and hyperuricemia are often associated to other comorbidities, a discussion about potential patient variability (or not) in the original studies would be useful (ex. are there any covariates that have been or have not been considered in the original studies).
Thanks for this comment and in the previous studies we cited, few of them considered covariates. But we agree with the reviewer that it is necessary for omics analysis and this point is discussed in Summary section.
Minor points: Rechecking the text may be useful:
Thank you very much for pointing out the mistakes in our manuscript and we have corrected these mistakes in the reversion.
line 15 (In?) “Recent years”;
We changed the sentence to: “In recent years”
line 20 “systematic metabolism”(?);
We changed the sentence to: “systemic metabolic changes”
line 110 “statistically significant (differentially abundant?) metabolites”;
We changed the sentence to: “Differential metabolites of statistical significance”
line 130 “data sharing and re-analyze” (re-analysis?);
We changed the sentence to: “data sharing and re-analysis”
line 146 “observed in urine and fecal (samples?) from gout patients”;
We changed the sentence to: “observed in urine and fecal samples from gout patients”
line 149 “threonine metabolism and some of lipid” (unclear);
We changed the sentence to: “and some lipid metabolism”
line 177 “consistent with metabolomic data”;
We changed the sentence to: “consistent with metabolomic data”
line180 “a combination of microbiome and metabolome analysis …consider moving here the ending part of the sentence “in feces from gout patients”;
We changed the sentence to: “Thus, in feces from gout patients, a combination of microbiome and metabolome analysis revealed up-regulation of microbiota, such as Bacteroides, Porphyromonadaceae Rhodococcus, Erysipelatoclostridium and Anaerolineaceae, as well as altered metabolites involved in uric acid excretion, purine metabolism, and inflammatory responses”
line 216 – consider not referring to HUA as “common metabolic disease”;
We deleted the sentence: “As common metabolic diseases”
line 242 “have yet been (to be?) established”, etc.
We changed the sentence to: “have yet to be established”
Some abbreviations are not written in full at first use: HUA, GA, ALDH16A1, PC, lysoPC, etc.
We spelled out the abbreviations in full at first use, such as “HU”, and “GA” to “gouty arthritis (GA)”, “ALDH16A1” to “aldehyde dehydrogenase 16A1 (ALDH16A1) ”, “PC” “phosphatidylcholine (PC) ”, “lysoPC” “lysophosphatidylcholine (LysoPC) ”.
Round 2
Reviewer 2 Report
Dear Authors,
Thank you for turning around a revision quickly.
I appreciate your time and thoughtfulness in editing the manuscript and find the Venn diagram in Figure 2 was helpful. One small comment I would make is please consider using a different color palette that is more inclusive of the red-green color bind readers and is softer on the eyes (I found the red/green tints to be very sharp and straining).
I wish you all the best with the publication.
Author Response
Dear reviewer,
We appreciate your helpful comments and choose a different color palette which is softer on the eyes.