Advanced Deep Learning and Neural Network Technologies for Image Recognition
A special issue of Electronics (ISSN 2079-9292). This special issue belongs to the section "Artificial Intelligence".
Deadline for manuscript submissions: closed (28 February 2022) | Viewed by 12583
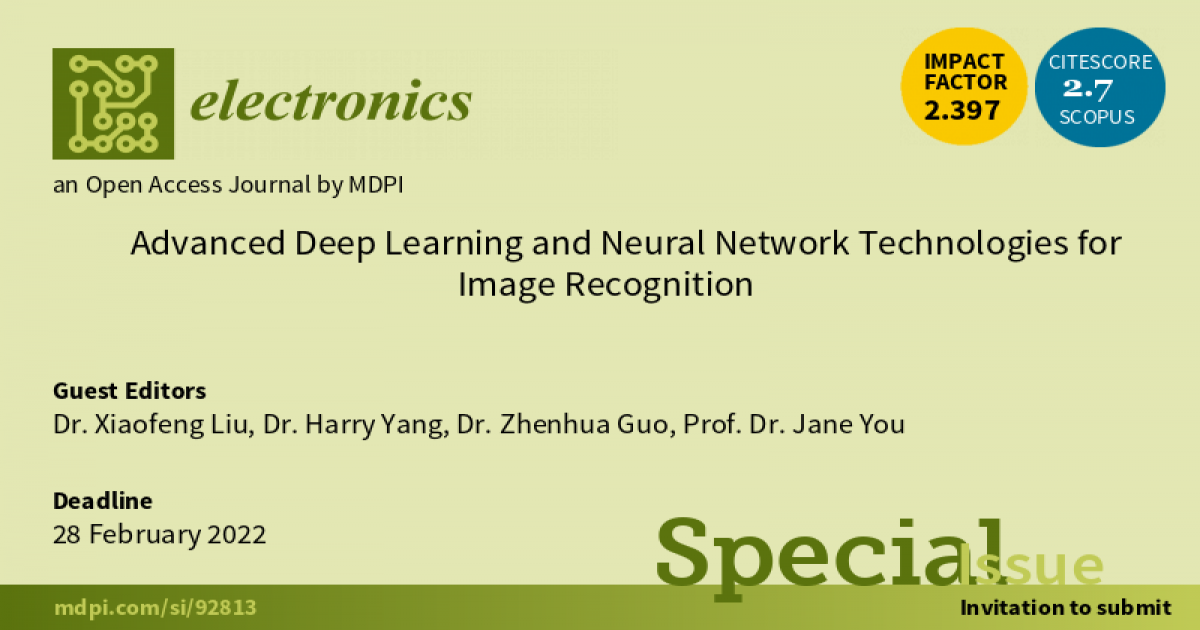
Special Issue Editors
Interests: deep learning; machine learning; medical informatics; physics-informed data-driven methods
Special Issues, Collections and Topics in MDPI journals
Interests: AI and computer vision; DeepFake generation and detection
Interests: pattern recognition; computer vision; biometrics; object detection
Special Issue Information
Dear Colleagues,
Visual recognition is a fundamental cognitive ability in humans which is essential for identifying objects/people. The in-depth explorations of deep learning and neural networks have facilitated advances in understanding the high-level semantic of visual contents. However, despite these advances, there are still many challenges remaining. For example, modern deep learning systems are data starved and rely greatly on the i.i.d. assumption of training and testing tasks. The common image corruptions and the recent presence of adversarial noise or attacks have raised higher requirements of robustness. In addition, application in biometrics, medical image analysis, and autonomous driving scenes require inducing non-trivial domain knowledge.
The main aim of this Special Issue is to seek original contributions that address the above challenges or highlight emerging applications in image recognition. The topics of interest include but are not limited to:
- Robust recognition with perturbations;
- Explainable deep learning;
- Multimodal or multiview recognition;
- Weakly supervised learning;
- Self-supervision learning;
- Privacy-aware recognition;
- Data augmentation;
- Transfer learning and domain adaptation;
- Few/one shot learning;
- GANs for image recognition;
- Deepfake detection;
- Distance metric learning;
- Uncertainty estimation;
- Out-of-distribution detection;
- Activity and online learning;
- Advanced and novel biometrics systems;
- Practical and reliable medical image recognition
Dr. Xiaofeng Liu
Dr. Harry Yang
Dr. Zhenhua Guo
Prof. Dr. Jane You
Guest Editors
Manuscript Submission Information
Manuscripts should be submitted online at www.mdpi.com by registering and logging in to this website. Once you are registered, click here to go to the submission form. Manuscripts can be submitted until the deadline. All submissions that pass pre-check are peer-reviewed. Accepted papers will be published continuously in the journal (as soon as accepted) and will be listed together on the special issue website. Research articles, review articles as well as short communications are invited. For planned papers, a title and short abstract (about 100 words) can be sent to the Editorial Office for announcement on this website.
Submitted manuscripts should not have been published previously, nor be under consideration for publication elsewhere (except conference proceedings papers). All manuscripts are thoroughly refereed through a single-blind peer-review process. A guide for authors and other relevant information for submission of manuscripts is available on the Instructions for Authors page. Electronics is an international peer-reviewed open access semimonthly journal published by MDPI.
Please visit the Instructions for Authors page before submitting a manuscript. The Article Processing Charge (APC) for publication in this open access journal is 2400 CHF (Swiss Francs). Submitted papers should be well formatted and use good English. Authors may use MDPI's English editing service prior to publication or during author revisions.
Keywords
- Robust recognition with perturbations
- Explainable deep learning
- Multimodal or multiview recognition
- Weakly supervised learning
- Self-supervision learning
- Privacy-aware recognition
- Data augmentation
- Transfer learning and domain adaptation
- Few/one shot learning
- GANs for image recognition
- Deepfake detection
- Distance metric learning
- Uncertainty estimation
- Out-of-distribution detection
- Activity and online learning
- Advanced and novel biometrics systems
- Practical and reliable medical image recognition
Benefits of Publishing in a Special Issue
- Ease of navigation: Grouping papers by topic helps scholars navigate broad scope journals more efficiently.
- Greater discoverability: Special Issues support the reach and impact of scientific research. Articles in Special Issues are more discoverable and cited more frequently.
- Expansion of research network: Special Issues facilitate connections among authors, fostering scientific collaborations.
- External promotion: Articles in Special Issues are often promoted through the journal's social media, increasing their visibility.
- e-Book format: Special Issues with more than 10 articles can be published as dedicated e-books, ensuring wide and rapid dissemination.
Further information on MDPI's Special Issue polices can be found here.