Quality of Open Data
A special issue of Information (ISSN 2078-2489). This special issue belongs to the section "Information Processes".
Deadline for manuscript submissions: closed (30 January 2020) | Viewed by 48407
Special Issue Editors
Interests: information quality; open data; NLP; semantic web; fake news
Special Issues, Collections and Topics in MDPI journals
Interests: data quality in linked data; time-related quality dimensions; semantic web; information extraction; big data
Special Issue Information
Dear Colleagues,
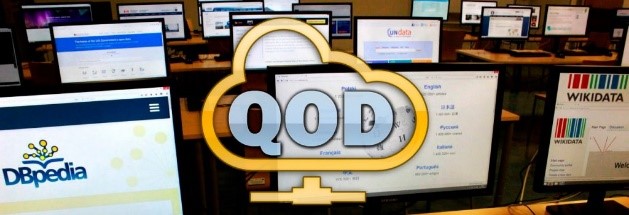
The 2nd International Workshop on Quality of Open Data (QOD 2019) will be held in June 2019 in Seville in conjunction with the 22nd International Conference on Business Information Systems. The goal of the workshop is to bring together different communities working on quality in Wikipedia, DBpedia, Wikidata, OpenStreetMap, Wikimapia, and other open knowledge bases. The workshop calls for sharing research experiences and knowledge related to quality assessment in open data. We invite papers that provide methodologies and techniques which can help to verify and enrich various community-based services in different languages. This Special Issue of Information intends to attract submissions on the topic of quality issues in Open Data. There is no Article Processing Charge for extended version of the accepted papers at the QOD 2019 workshop.
QOD 2019 website: http://qod.bisconf.info
Dr. Włodzimierz Lewoniewski
Dr. Anisa Rula
Dr. Krzysztof Węcel
Guest Editors
Manuscript Submission Information
Manuscripts should be submitted online at www.mdpi.com by registering and logging in to this website. Once you are registered, click here to go to the submission form. Manuscripts can be submitted until the deadline. All submissions that pass pre-check are peer-reviewed. Accepted papers will be published continuously in the journal (as soon as accepted) and will be listed together on the special issue website. Research articles, review articles as well as short communications are invited. For planned papers, a title and short abstract (about 100 words) can be sent to the Editorial Office for announcement on this website.
Submitted manuscripts should not have been published previously, nor be under consideration for publication elsewhere (except conference proceedings papers). All manuscripts are thoroughly refereed through a single-blind peer-review process. A guide for authors and other relevant information for submission of manuscripts is available on the Instructions for Authors page. Information is an international peer-reviewed open access monthly journal published by MDPI.
Please visit the Instructions for Authors page before submitting a manuscript. The Article Processing Charge (APC) for publication in this open access journal is 1600 CHF (Swiss Francs). Submitted papers should be well formatted and use good English. Authors may use MDPI's English editing service prior to publication or during author revisions.
Keywords
- Data quality
- Open data
- LOD
- Information enrichment
- Geospatial data
- Knowledge base
- Wikipedia
- DBpedia
- Wikidata
- OpenStreetMap
- Wikimapia
Benefits of Publishing in a Special Issue
- Ease of navigation: Grouping papers by topic helps scholars navigate broad scope journals more efficiently.
- Greater discoverability: Special Issues support the reach and impact of scientific research. Articles in Special Issues are more discoverable and cited more frequently.
- Expansion of research network: Special Issues facilitate connections among authors, fostering scientific collaborations.
- External promotion: Articles in Special Issues are often promoted through the journal's social media, increasing their visibility.
- e-Book format: Special Issues with more than 10 articles can be published as dedicated e-books, ensuring wide and rapid dissemination.
Further information on MDPI's Special Issue polices can be found here.