Applications of Image Processing and Pattern Recognition in Biometrics
A special issue of Applied Sciences (ISSN 2076-3417). This special issue belongs to the section "Computing and Artificial Intelligence".
Deadline for manuscript submissions: 20 November 2024 | Viewed by 8580
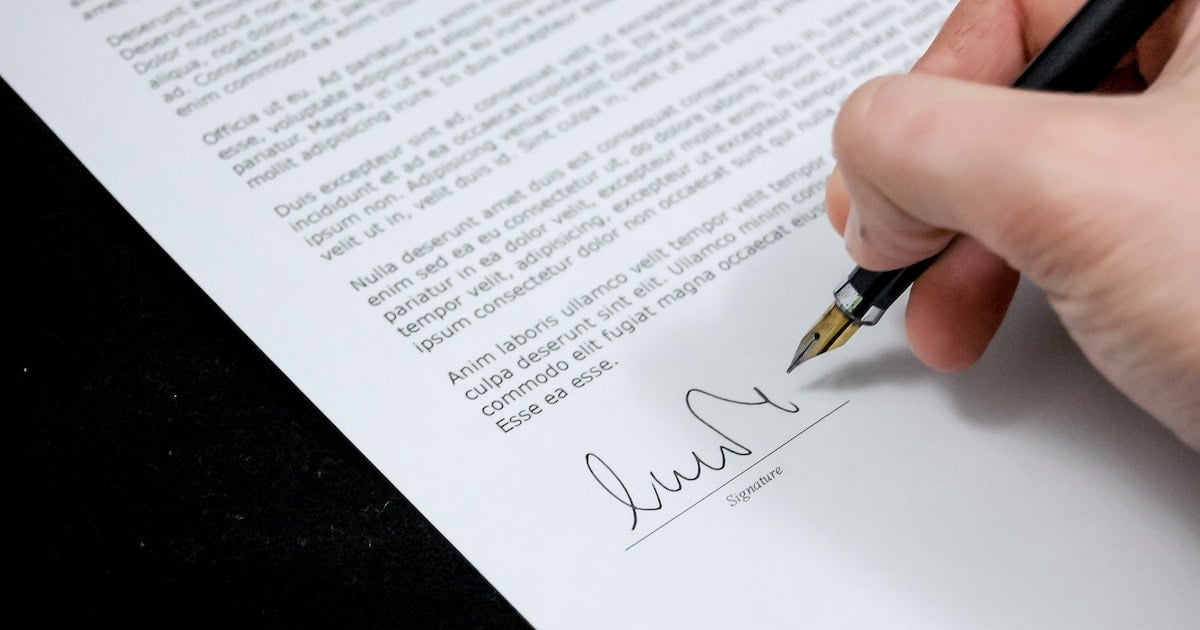
Special Issue Editors
Interests: digital signal; image processing; computer vision; pattern recognition; handwriting biometry
Special Issues, Collections and Topics in MDPI journals
Special Issue Information
Dear Colleagues,
We would like to invite you to submit your research work to our Special Issue, “Applications of Image Processing and Pattern Recognition in Biometrics”.
This Special Issue focuses upon the interdisciplinary processing, extraction of information, and recognition of all types of biometric content contained in digital images, with important applications in high-level understanding. Biometrics is the task of identifying individuals with the use of their physiological or behavioral traits. A number of relative scientific disciplines describes this topic; among others acquisition, image analysis, machine learning, computer vision are some notable examples. This Special Issue aims to bring together and present recent advances in theory, comprehensive studies, and surveys, as well as contemporary and new research ideas in order to process and understand the biometric content of images as a whole or as a part of a combined infrastructure. Submissions should provide progress and advance the state-of-the-art at image processing, computer vision, and pattern recognition/machine learning fields with a primary focus on physiological and behavioral biometric attributes.
Broad topics/keywords and areas of interest include, but are not limited to:
- Individual biometric modalities, including established and traditional, contemporary modalities, as well as any type of their fusion.
- Hardware technologies for biometric image processing and pattern recognition.
- Core groundwork theory and applications on image processing, pattern recognition, machine learning, and computer vision techniques relevant to biometrics processing and analysis.
- Datasets, evaluation, and benchmarking.
- Image processing, pattern recognition, machine learning, and computer vision for biometrics in healthcare, banking, IOT, etc.
- Image processing, pattern recognition, machine learning, and computer vision for biometrics in forensic applications.
Dr. Elias N. Zois
Prof. Dr. Dimitrios Kalivas
Guest Editors
Manuscript Submission Information
Manuscripts should be submitted online at www.mdpi.com by registering and logging in to this website. Once you are registered, click here to go to the submission form. Manuscripts can be submitted until the deadline. All submissions that pass pre-check are peer-reviewed. Accepted papers will be published continuously in the journal (as soon as accepted) and will be listed together on the special issue website. Research articles, review articles as well as short communications are invited. For planned papers, a title and short abstract (about 100 words) can be sent to the Editorial Office for announcement on this website.
Submitted manuscripts should not have been published previously, nor be under consideration for publication elsewhere (except conference proceedings papers). All manuscripts are thoroughly refereed through a single-blind peer-review process. A guide for authors and other relevant information for submission of manuscripts is available on the Instructions for Authors page. Applied Sciences is an international peer-reviewed open access semimonthly journal published by MDPI.
Please visit the Instructions for Authors page before submitting a manuscript. The Article Processing Charge (APC) for publication in this open access journal is 2400 CHF (Swiss Francs). Submitted papers should be well formatted and use good English. Authors may use MDPI's English editing service prior to publication or during author revisions.
Benefits of Publishing in a Special Issue
- Ease of navigation: Grouping papers by topic helps scholars navigate broad scope journals more efficiently.
- Greater discoverability: Special Issues support the reach and impact of scientific research. Articles in Special Issues are more discoverable and cited more frequently.
- Expansion of research network: Special Issues facilitate connections among authors, fostering scientific collaborations.
- External promotion: Articles in Special Issues are often promoted through the journal's social media, increasing their visibility.
- e-Book format: Special Issues with more than 10 articles can be published as dedicated e-books, ensuring wide and rapid dissemination.
Further information on MDPI's Special Issue polices can be found here.