Predictive Analytics in Healthcare
A special issue of Applied Sciences (ISSN 2076-3417). This special issue belongs to the section "Biomedical Engineering".
Deadline for manuscript submissions: closed (20 May 2024) | Viewed by 14628
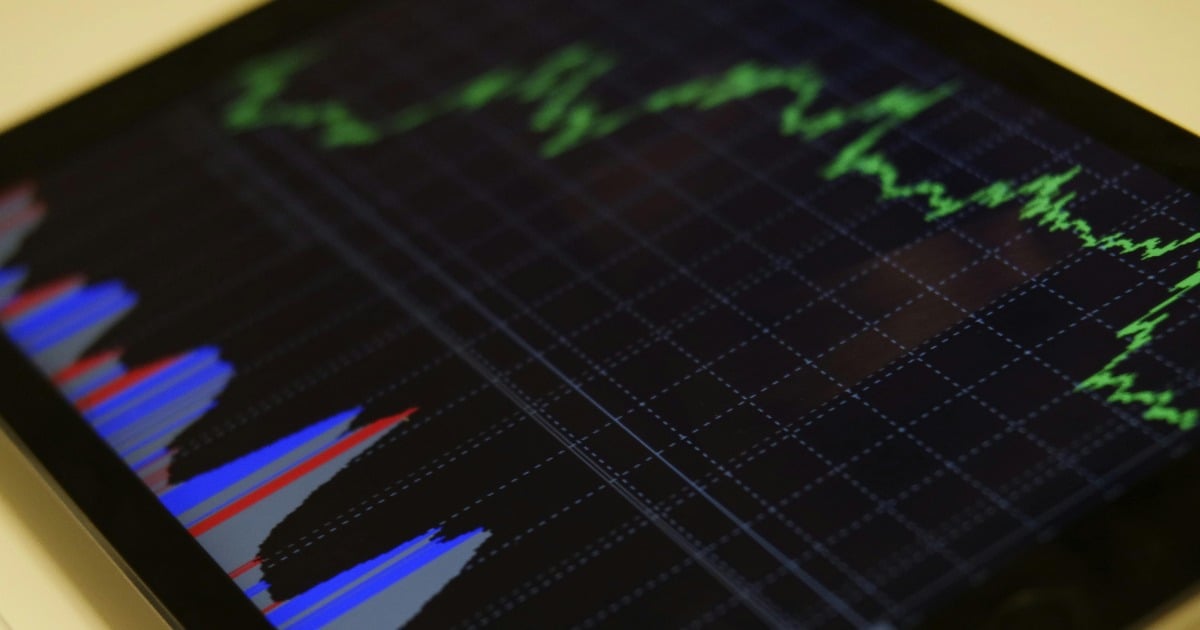
Special Issue Editor
Interests: quality of life; PROMs; rare diseases; clinical quality registries; clinical trials; new drugs and treatments
Special Issues, Collections and Topics in MDPI journals
Special Issue Information
Dear Colleagues,
Predictive analytics aims to alert clinicians and caregivers of the likelihood of events and outcomes before they occur, helping them to prevent and cure health issues. It utilises various techniques including modelling, data mining, and statistics, as well as artificial intelligence to evaluate historical and real-time data and make predictions about the future. These predictions offer a unique opportunity to see into the future and identify future trends in patient care both at an individual level and at a cohort scale.
We are interested in articles that explore predictive analysis. Potential topics include, but are not limited to, the following:
- Data mining techniques and machine learning in healthcare;
- Benefits and significance of predictive analytics;
- Challenges and opportunities of big data analytics;
- Impact on care;
- Legal and ethical considerations of predictive analytics in healthcare.
Dr. Rasa Ruseckaite
Guest Editor
Manuscript Submission Information
Manuscripts should be submitted online at www.mdpi.com by registering and logging in to this website. Once you are registered, click here to go to the submission form. Manuscripts can be submitted until the deadline. All submissions that pass pre-check are peer-reviewed. Accepted papers will be published continuously in the journal (as soon as accepted) and will be listed together on the special issue website. Research articles, review articles as well as short communications are invited. For planned papers, a title and short abstract (about 100 words) can be sent to the Editorial Office for announcement on this website.
Submitted manuscripts should not have been published previously, nor be under consideration for publication elsewhere (except conference proceedings papers). All manuscripts are thoroughly refereed through a single-blind peer-review process. A guide for authors and other relevant information for submission of manuscripts is available on the Instructions for Authors page. Applied Sciences is an international peer-reviewed open access semimonthly journal published by MDPI.
Please visit the Instructions for Authors page before submitting a manuscript. The Article Processing Charge (APC) for publication in this open access journal is 2400 CHF (Swiss Francs). Submitted papers should be well formatted and use good English. Authors may use MDPI's English editing service prior to publication or during author revisions.
Keywords
- data mining
- big data
- prediction
- machine learning
- optimization
- healthcare
Benefits of Publishing in a Special Issue
- Ease of navigation: Grouping papers by topic helps scholars navigate broad scope journals more efficiently.
- Greater discoverability: Special Issues support the reach and impact of scientific research. Articles in Special Issues are more discoverable and cited more frequently.
- Expansion of research network: Special Issues facilitate connections among authors, fostering scientific collaborations.
- External promotion: Articles in Special Issues are often promoted through the journal's social media, increasing their visibility.
- e-Book format: Special Issues with more than 10 articles can be published as dedicated e-books, ensuring wide and rapid dissemination.
Further information on MDPI's Special Issue polices can be found here.