The Impacts of Urban Air Pollution Emission Density on Air Pollutant Concentration Based on a Panel Model
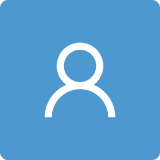
Round 1
Reviewer 1 Report
The authors if this manuscript have conducted an impressive study of urban characteristics on air pollution, incorporating a wide range of characteristics on three important air pollutants. The study is very well supported in the literature to construct a meaningful conceptual framework and is compared thoughtfully to previous findings in the discussion. The methods are appropriate and conducted well. This is a robust paper, but it does have several opportunities for improvement before publication.
In particular, there are several areas with high redundancy in this manuscript. Please reduce the overlap between your sections 1 and 2. There are several repeated statements here. I’d recommend reducing the amount of detail in section 1 for greater depth in section 2. Similarly, there is much overlap between your results and conclusions sections. I would encourage you to reduce the text devoted to restating your findings. Instead, please synthesize the findings here and explore recommendations related to them. What should urban planners and policymakers do to reduce air pollutant exposure? After such a thorough study using such diverse variables, you have an opportunity to make very direct recommendations here.
Additionally, I’d like to see a few aspects of your methods explained further. Each air pollutant has several different models. How were each of these models constructed? What led you to include or exclude particular variables from the first models to the last? Is it simply a matter of increasing complexity? Regarding the statistics, you also mention that it would help to use indices in the limitations section. Why did you not include indices in this study? Is there any risk of multicollinearity in the models you have created by not combining into indices or factors? Several studies that include so many variables will utilize a dimension reduction technique such as Principal Components Analysis to reduce the risk of multicollinearity. Is your model safe from this threat?
Finally, the limitations section is rather vague. You mention that more studies are needed, with more variables, but why? What specifically is lacking in your study that further research can address? The explanation you provide about a point measurement representing a whole city is valid and well needed, but I would like to see more precision in your own limitations regarding variables or methods.
Otherwise, I believe this is a strong paper worthy of publication after these revisions. Please see also some detailed notes below. Once complete, I would be happy to review the revision.
11 – “using panel model” to “using a panel model”
27 – unfamiliar with the term “social development” – perhaps be more clear with the benefit of urbanization here
44 – not sure what you mean by “spatial projected.” Perhaps “projection?” – even then, it is unclear what you mean
79 – change “it is needed” to “research is needed” or make a statement here that your study will fill these research gaps
108 – this is your first use of the term “yellow dust.” Please explain this prior to use in your research questions so the reader knows what you mean.
Section 4.2.1-3 – Can you speak more to how these models were constructed? How did you determine which variables to add in each iteration of the model?
398-399 – I don’t see tables as high as 19 or 20. Are these references correct?
526 – I’d recommend changing “against” to a more directly relational term such as “contrary to”
621 – do you mean “yellow dust” when you say “yellow sand” here?
746 – “conducts” to “conduct”
Author Response
Point 1: In particular, there are several areas with high redundancy in this manuscript. Please reduce the overlap between your sections 1 and 2. There are several repeated statements here. I’d recommend reducing the amount of detail in section 1 for greater depth in section 2. Similarly, there is much overlap between your results and conclusions sections. I would encourage you to reduce the text devoted to restating your findings. Instead, please synthesize the findings here and explore recommendations related to them. What should urban planners and policymakers do to reduce air pollutant exposure? After such a thorough study using such diverse variables, you have an opportunity to make very direct recommendations here.
Response 1: Thank you for your comment. As you suggested, we have revised the manuscript to make it more concise by getting rid of redundant statements in Chapter 1 and 2. Chapters 1 and 2 have been reorganized while mainly focusing on research questions. The main interest of this manuscript is to discuss the effects of density on air pollution in terms of urban planning and policy. Various studies have evaluated whether higher density induces compact development to decrease air pollution or compact development increases the density of pollutants. Chapters 1 and 2 have been revised to discuss the effects of the spatial density of urban air pollution emission on the concentration of air pollutants, which is the objective of this study, based on previous studies in line with this aspect.
Point 2: Additionally, I’d like to see a few aspects of your methods explained further. Each air pollutant has several different models. How were each of these models constructed? What led you to include or exclude particular variables from the first models to the last? Is it simply a matter of increasing complexity? Regarding the statistics, you also mention that it would help to use indices in the limitations section. Why did you not include indices in this study? Is there any risk of multicollinearity in the models you have created by not combining into indices or factors? Several studies that include so many variables will utilize a dimension reduction technique such as Principal Components Analysis to reduce the risk of multicollinearity. Is your model safe from this threat?
Response 2: Each air pollutant has a different source and the concentration characteristics of each pollutant vary according to the reactions in the atmosphere after being emitted. NO2 is affected by direct sources such as the fuel combustion of automobiles. Contrarily, O3 and PM10 are influenced by an external environment as well as a direct source. Furthermore, the principle of generating O3 in the atmosphere is different from that of producing PM10. The authors used a different set of variables for each air pollutant to analyze the spatial characteristics affecting each air pollutant. In order to evaluate the specific effects of density (among various spatial characteristics), we examined the effects of individual variables of density, rather than spatial or density characteristics.
We also calculated correlation coefficients and VIF to resolve the multicollinearity issue of independent variables that may appear when analyzing by using individual variables. In order to judge the independence of independent variables, the multicollinearity of all variables, used for each dependent variable, was tested (Table 13). Pearson's correlation was used to test multicollinearity. The coefficients of all independent variables except for that between sunshine duration and the percentage of sunshine were below 0.7, the threshold of this study (Anderson et al., 1996; Griffiths et al., 1993; Yang and Han, 2018), we could judge that there was no multicollinearity issue. Sunshine duration and the percentage of sunshine were used separately while considering the characteristics of a dependent variable due to their multicollinearity.
Meanwhile, an analysis of the variance inflation factor (VIF) was performed to confirm stricter variance inflection factor (VIF) values, and the results of the analysis showed that it was below 10, the reference value (Chatterjee and Price, 1991; Kennedy, 1992; Hair et al., 1998). In addition, since it did not exceed 5, the stricter threshold suggested by Hair et al. (2011), it was determined that there was no multicollinearity issue.
Point 3: Finally, the limitations section is rather vague. You mention that more studies are needed, with more variables, but why? What specifically is lacking in your study that further research can address? The explanation you provide about a point measurement representing a whole city is valid and well needed, but I would like to see more precision in your own limitations regarding variables or methods.
Response 3: This study evaluated specific characteristics such as density in terms of variables and methods. Therefore, future studies are needed to analyze causal relationships comprehensively. We present the limitations of this study and future research needs in this respect.
Point 4: 11 – “using panel model” to “using a panel model”
Response 4: It has been revised as suggested.
Point 5: 44 – not sure what you mean by “spatial projected.” Perhaps “projection?” – even then, it is unclear what you mean
Response 5: We have deleted the sentence.
Point 6: 79 – change “it is needed” to “research is needed” or make a statement here that your study will fill these research gaps
Point 7: 108 – this is your first use of the term “yellow dust.” Please explain this prior to use in your research questions so the reader knows what you mean.
Response 7: The authors have added additional description of yellow dust.
Point 8: Section 4.2.1-3 – Can you speak more to how these models were constructed? How did you determine which variables to add in each iteration of the model?
Response 8: Model development process is added to the “Conceptual Framework and Hypothesis” section.
Point 9: 398-399 – I don’t see tables as high as 19 or 20. Are these references correct?
Response 9: Table numbers have been revised.
Point 10: 526 – I’d recommend changing “against” to a more directly relational term such as “contrary to”
Response 10: We maintained the expression because I thought that the context to is more appropriate.
Point 11: 621 – do you mean “yellow dust” when you say “yellow sand” here?
Response 11: The term has been unified as “yellow dust”. Yellow dust refers to a phenomenon that describes the slowly descending fine sand dust, which is firstly blown up by wind from the arid red clay region mainly located in northern China or Mongolia, dispersed in the atmosphere, and covers the sky or the descending cloud of dust. It generally occurs from Mar to May, when the activity of low pressure is vigorous, and sometimes it flies to Japan, the Pacific Ocean, and North America through Korea by being carried by strong westerly wind. When a severe yellow dust phenomenon occurs, the sun appears yellowish-brown because sunlight is scattered or blocked in the air. Moreover, sand dust often deposits on the ground.
The size of loess or red clay, which is the main component of yellow dust, is between 0.2 to 20μm. Yellow dust flown to South Korea is mostly between 1 to 10μm. The yellow dust phenomenon has existed for a long time. However, people react to it more sensitively because it passes through rapidly industrialized regions in China before it arrives in South Korea. As a result, the yellow dust contains higher concentrations of heavy metals such as silicon, lead, cadmium, nickel, and chromium.
Point 12: 746 – “conducts” to “conduct”
Response 12: The authors have been changed “conducts” to “conduct”
Reviewer 2 Report
This study has explored the relationship between land use, urban structure and urban environment in South Korea. The topic is interesting and important when evaluating the effect of urban expansion. The results show that different pollutants respond differently to meteorological conditions and local variables, which reveals the complex mechanism between air pollutant concentrations and other factors. The overall results are consistent but there are some parts that need more clarifications. Therefore, I would suggest major revision before it could be considered for publication.
Comments
- It is necessary to show a spatial map of the 35 municipalities so that readers who are not familiar with South Korea could have a better idea about their locations.
- The analysis in this work is done for all 35 municipalities. Do the authors check the results for each municipality? Are they consistent?
- The monthly data are used for analysis but it seems hourly air pollutant concentrations are available. For pollutants like O3 and NO2 that have relatively short lifetime, using monthly data is less likely to reveal its dependence on other factors considered in this work. Thus I think the authors should present some additional results using there high-frequency data.
- Add descriptions about the variables used in Table 3.
- The independent variables used in the regression models are not really “independent” in my opinion. For example, there is a clear dependence between temperature and precipitation. I suspect that the collinearity of independent variables would bias the conclusions in this work. Some justifications like checking the correlations between independent variables or excluding the correlated variables in the regression model are expected.
- At line 363 and line 400, why do NO2 and O3 have opposite correlations with the meteorological variables? For example, why increase of wind speed would lead to a decrease of NO2 but an increase of O3?
- The regression model in this work is basically a linear model and the non-linear effect between different variables is neglected (e.g., missing the terms like TP*WB, TP*MP, etc). Hence I would like to know how much variance of the response variables (i.e., air pollutant concentrations in this work) could be explained by the selected independent variables? If the selected variables could not explain most variance of response variables, then it is dangerous to make a conclusion about the relationship between land use, urban structure and urban environment based on the current results.
Author Response
Point 1: It is necessary to show a spatial map of the 35 municipalities so that readers who are not familiar with South Korea could have a better idea about their locations.
Response 1: Thank you for your good comment. We have generated a map showing the geographic location of these 35 municipalities and added it in the manuscript.
Point 2: The analysis in this work is done for all 35 municipalities. Do the authors check the results for each municipality? Are they consistent?
Response 2: We have checked the consistency of data by creating time-series graphs for each variable and each municipality in the exploratory analysis stage.
Point 3: The monthly data are used for analysis but it seems hourly air pollutant concentrations are available. For pollutants like O3 and NO2 that have relatively short lifetime, using monthly data is less likely to reveal its dependence on other factors considered in this work. Thus I think the authors should present some additional results using there high-frequency data.
Response 3: The concentrations of air pollutants have a relatively short lifetime, compared to the long lifetime of urban spaces. Additionally, meteorological characteristics have a different lifetime than these two. This study used a monthly time step to derive a model that combines these various characteristics. Most studies on the topic used the cross-sectional patterns of annual mean values, and the temporal unit of this study can be a major distinction of this study compared to other studies. The Ministry of Environment of the Republic of Korea processes and discloses only highly reliable monthly data from data produced on an hourly basis in order to utilize the data for the research and policy. However, as pointed out, this study presented a monthly panel graph.
Point 4: Add descriptions about the variables used in Table 3.
Response 4: We added descriptions about the variables in Table 3.
Point 5: The independent variables used in the regression models are not really “independent” in my opinion. For example, there is a clear dependence between temperature and precipitation. I suspect that the collinearity of independent variables would bias the conclusions in this work. Some justifications like checking the correlations between independent variables or excluding the correlated variables in the regression model are expected.
Response 5: We calculated correlation coefficients and VIF to diagnose the independence of independent variables and to resolve the multicollinearity issue. Although the two variables pointed out by the reviewer have a significant correlation, the multicollinearity test showed that there was no multicollinearity issue. Therefore, these two variables were used in this study.
Point 6: At line 363 and line 400, why do NO2 and O3 have opposite correlations with the meteorological variables? For example, why increase of wind speed would lead to a decrease of NO2 but an increase of O3?
Response 6: We believe that the results were due to seasonal and spatial effects. While NO2 is emitted more in the winter due to heating, O3 increases in the summer when photochemical reactions are likely to occur. In Korea, wind speed is generally stronger in the winter than in the summer. Moreover, the mean wind speed, unlike other meteorological variables, has a greater spatial difference than a temporal change. It seems that NO2 is low and O3 increases due to the effects of diffusion as well as seasonal effects in regions with strong wind speeds.
In general, NO2 is generated from a line source (although it can be affected by a point source depending on an environment. As a result, there is a concentration gradient of NO2 in the atmosphere. When the wind blows, the concentration can decrease due to dispersion. Contrarily, O3 is mostly generated by photochemical reactions. When PM10 is high in the atmosphere such as Seoul in South Korea, the wind can clear the sky by dispersing these particles. Consequently, it increases ozone concentration by stimulating photochemical reactions.
Point 7: The regression model in this work is basically a linear model and the non-linear effect between different variables is neglected (e.g., missing the terms like TP*WB, TP*MP, etc). Hence I would like to know how much variance of the response variables (i.e., air pollutant concentrations in this work) could be explained by the selected independent variables? If the selected variables could not explain most variance of response variables, then it is dangerous to make a conclusion about the relationship between land use, urban structure and urban environment based on the current results.
Response 7: The basic regression equation of this study has a nonlinear relationship between the explanatory variable and the dependent variable. However, it is a linear model for parameters. Therefore, the model of this study is a multiple regression linear model, and it can be estimated using the ordinary least squares (OLS) method. However, in the case of the panel data used in this study, when constant terms and individual effects (error term; ui) are stochastic variables and it is estimated using OLS, it can have the first-order autocorrelation (AR(1)) issue. Therefore, the estimation model should be tested first to resolve the first-order autocorrelation issue of the error terms and obtain efficient estimators. As a result, this study tested the validity of each model while using a pooled OLS model and a two-way error component panel model as candidate estimation models for panel data analysis.
When intra- and inter-subject effects were compared, these characteristics could be explained in more detail. Additionally, when the explanatory coefficients of NO2-total source density model, O3-urban sprawl model, and PM10-source density model were compared, the explanatory coefficients of NO2, PM10, and O3 were 0.457, 0.359, and 0.412, respectively. The explanatory coefficients within regions were 0.333, 0.495, and 0.467 for NO2, PM10, and O3, respectively. Moreover, the explanatory coefficients between regions were 0.619, 0.353, and 0.154 for NO2, PM10, and O3, respectively.