Estimating Toll Road Travel Times Using Segment-Based Data Imputation
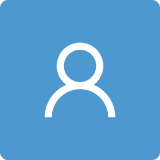
Round 1
Reviewer 1 Report
Dear Authors,a very interesting enquiry on a daily basis involving millions of persons.From sources of data to the delicate aspect of missing ones,your paperwork shows the solidity of the models proposed.A strong element which facilitates the comprehension,is the complete illustration trough graphics.The trace shows a leptocurtic obvious distribution,Central Limit convergence,in the relevant segments of high traffic density,as a support for models validation.
The convergence in general,between SVR and LSTM is well demonstrated.
I would suggest eventually to give a deeper analysis on the ARIMA models.MA component could eventually refer to the ground trip,while AR component,to the present traffic condition.Therefore,the d component on the characteristic ARIMA model could refer to the previous traffic conditions of the analysed segment.This suggests a further question,are we in a Bayesian context or not,which focuses on the possible presence of memory data within the model.It is also to see whether ARIMA results converges on LSTM.
Author Response
Response to Reviewer 1 Comments
I would suggest eventually to give a deeper analysis on the ARIMA models. MA component could eventually refer to the ground trip, while AR component, to the present traffic condition. Therefore, the d component on the characteristic ARIMA model could refer to the previous traffic conditions of the analysed segment. This suggests a further question, are we in a Bayesian context or not, which focuses on the possible presence of memory data within the model. It is also to see whether ARIMA results converges on LSTM.
Response: Thank you for your valuable feedback on the paper. We appreciate your suggestion to analyze ARIMA models in more depth. However, we would like to clarify that in our case, when deploying the travel time estimation model, we only had access to traffic speed and flow data, not travel time data (which were collected only temporarily using the Bluetooth readers). As a result, it was not feasible to utilize the ARIMA model for travel time estimation because the travel time ground truth data were not available.
Reviewer 2 Report
Overall, I feel the scientific and technical contents of this paper is not sufficient to be accepted for publication. The applied LSTM and SVM models are not novel, and their application for travel time/travel speed estimation are largely investigated.
1. In beginning, it said "Two models were developed for travel time estimation under a variety of traffic conditions...", I would expect to see some differences by using the models in a more complex traffic scenarios. However, nothing has been special in this regard.
2. It mentioned that the camera calibrated data has been used as samples for estimation, while the bluetooth reader data as groundtruth data for examination. I doubt how can process the camera data as segment-based data imputation, instead, the bluetooth data is more appropriate to be processed as segment travel time samples.
3. What is the prediction horizon for LSTM or SVM?
4. In abstract, "The sensitivity analysis 26 of the missing input data in this study also reveals that LSTM is more robust to the high degree of 27 missing data than the SVR.", which is not illustrated and verified in any cases showing the performance of LSTM in processing fluctuated data.
Please have an thorough English proofreading.
Author Response
Response to Reviewer 2 Comments
Point 1: Overall, I feel the scientific and technical contents of this paper is not sufficient to be accepted for publication. The applied LSTM and SVM models are not novel, and their application for travel time/travel speed estimation are largely investigated.
In beginning, it said "Two models were developed for travel time estimation under a variety of traffic conditions...", I would expect to see some differences by using the models in a more complex traffic scenarios. However, nothing has been special in this regard.
Response 1: Thank you for taking the time to review our paper. We appreciate your feedback, and we would like to address your concerns regarding the scientific and technical contents of the paper.
In our study, we aimed to contribute to the field in three specific areas. Firstly, we proposed a segment-based data imputation technique, which addresses the challenge of missing data in travel time estimation.
Secondly, we conducted a comprehensive comparison between LSTM and SVM models under various traffic conditions.
Lastly, our research focused on determining the sensitivity of these models to missing data. This aspect is crucial for understanding the robustness and reliability of the models when confronted with incomplete datasets—an issue that is often encountered in real-world applications.
Point 2: It mentioned that the camera calibrated data has been used as samples for estimation, while the bluetooth reader data as groundtruth data for examination. I doubt how can process the camera data as segment-based data imputation, instead, the bluetooth data is more appropriate to be processed as segment travel time samples.
Response 2: Our segment-based data imputation method assumes that the traffic patterns at different points on the same segment are more similar, compared with traffic patterns on the different segments. Therefore, when traffic data are missing, such gaps can be imputed using nearby cameras within the same road segment.
Bluetooth devices were installed temporarily only to collect the training dataset (actual travel times) for travel time model development. As a result, the real-time Bluetooth data were not collected when the models were deployed.
We added some description in Section 3.1 to clarify the reviewer’s concern.
Point 3: What is the prediction horizon for LSTM or SVM?
Response 3: In this study, we developed travel time estimation models; they are not prediction models. Notably, the data were aggregated to every 1 minute.Point 4: In abstract, "The sensitivity analysis of the missing input data in this study also reveals that LSTM is more robust to the high degree of missing data than the SVR.", which is not illustrated and verified in any cases showing the performance of LSTM in processing fluctuated data.
Response 4: We apologize for not making this clear. We revised the last paragraph in Section 6.4 to provide an explanation of sensitivity analysis.
“For example, the MAPEs of the MLR and IM models increased substantially when the missing data level exceeded 60% while the MAPEs of the LSTM and SVR models increased relatively slowly. Additionally, the LSTM model had a lower MAPE than the SVR model, especially for a higher range of missing data, implying that the LSTM was more robust to missing data than the SVR model.”
Point 5: Please have a thorough English proofreading. Response 5: The revised manuscript has been proofread by a professional English editor. Please see attached for the certificate.Author Response File: Author Response.pdf
Reviewer 3 Report
The bibliographic study is complex and up to date.
The authors of this article analyze travel times using machine learning by using support vector regression (SVR) and a long short-term memory (LSTM) models.
The case study conducted on a busy road connecting central and northern Bangkok takes input data directly from traffic using video cameras and Bluetooth readers.
These data were entered into the developed models and trained until their validation.
Thus, the authors compared the travel time estimation performance of the two machine learning methods (SVR and LSTM) with other methods used, namely: multiple linear regression (MLR) and instantaneous model (IM).
The machine learning models (SVR and LSTM) thus performed better than the benchmark models when raw input data and imputed input data were used.
In conclusion, it is a well analyzed and mathematically modeled article, and the solutions found are relevant and provide a starting point for the development of other models regarding the estimation of travel times.
Author Response
Response to Reviewer 3 Comments
The bibliographic study is complex and up to date.
The authors of this article analyze travel times using machine learning by using support vector regression (SVR) and a long short-term memory (LSTM) models.
The case study conducted on a busy road connecting central and northern Bangkok takes input data directly from traffic using video cameras and Bluetooth readers.
These data were entered into the developed models and trained until their validation.
Thus, the authors compared the travel time estimation performance of the two machine learning methods (SVR and LSTM) with other methods used, namely: multiple linear regression (MLR) and instantaneous model (IM).
The machine learning models (SVR and LSTM) thus performed better than the benchmark models when raw input data and imputed input data were used.
In conclusion, it is a well analyzed and mathematically modeled article, and the solutions found are relevant and provide a starting point for the development of other models regarding the estimation of travel times.
Response: Thank you for your kind comments on our article.
Reviewer 4 Report
General evaluation of the article:
· Title: Suitable
· Keywords: the first and fourth words are adequate, but the second and third keywords must be added by the word "applications"
· Abstract: Suitable.
· Itemization: Correct
· Text: clear and objective
· Clear equations related to the object of study.
· Bibliographic research: objective and related to the article theme
· Technical/academic content of the article presents a study of interest for evaluating traffic flow.
· Conclusions: Consistent with the content.
Specific Comments:
· The text aroused several doubts:
a. What would be the relationship of this study developed with Sustainability? Why should this article be published?
b. What would be the travel times and average travel speeds by direct measurements performed by the authors? What would be the dispersions between these measures and the estimated values in the article?
Author Response
Response to Reviewer 4 Comments
Point 1: Keywords: the first and fourth words are adequate, but the second and third keywords must be added by the word "applications". Response 1: Thank you for your comments. We have revised the second and third keywords as per your suggestion. Keywords: travel time estimation; long short-term memory applications; support vector regression applications; toll roadsPoint 2: What would be the relationship of this study developed with Sustainability?
Response 2: Accurate travel time estimation on the tollway supports sustainable transportation through the optimization of traffic flow, the reduction of vehicle emissions, the promotion of sustainable mode choice and shift, and the informed planning and design of transportation infrastructure. Collectively, these factors contribute to a more efficient, environmental-friendly, and sustainable transportation system.
We have edited the abstract and the first paragraph of the introduction (Section 1) to demonstrate the relationship of this study to sustainability.
Abstract: "Efficient and sustainable transportation is crucial for addressing the environmental and social challenges associated with urban mobility. Accurate estimation of travel time plays a pivotal role in traffic management and trip planning. This study focused on leveraging machine learning models to enhance travel time estimation accuracy on toll roads under diverse traffic conditions. [..]"Introduction: "Travel time, a key parameter in intelligent transportation systems, plays a vital role in promoting sustainability. Road operators rely on real-time travel time information to effectively manage traffic in various situations, including normal conditions, roadworks, and incidents. Historical travel time data supports planning processes and facilitates performance evaluation. Additionally, travelers utilize en route travel times within advanced traveler information systems to make informed decisions regarding their journeys, such as choosing the optimal departure time and route. By optimizing travel time information, sustainable transportation practices are fostered, leading to improved traffic management and enhanced travel planning for a greener and more efficient transportation system."
Point 3: Why should this article be published?Response 3: Thank you for raising the question regarding the publication value of our article. We appreciate the opportunity to explain why we believe our paper should be considered for publication.
In our study, we aimed to contribute to the field in three specific areas. Firstly, we proposed a segment-based data imputation technique, which addresses the challenge of missing data in travel time estimation.
Secondly, we conducted a comprehensive comparison between LSTM and SVM models under various traffic conditions.
Lastly, our research focused on determining the sensitivity of these models to missing data. This aspect is crucial for understanding the robustness and reliability of the models when confronted with incomplete datasets—an issue that is often encountered in real-world applications.
Point 4: What would be the travel times and average travel speeds by direct measurements performed by the authors?
Response 4: We collected direct measurements of travel times and average travel speeds using Bluetooth readers, as described in Section 3. We apologize for any confusion and we can provide more details if needed.
Point 5: What would be the dispersions between these measures and the estimated values in the article?
Response 5: To address the differences between the direct measurements and the estimated values, we evaluated the models using a common metric, the mean percentage error (MAPE), as described in Section 5.3.
Reviewer 5 Report
The MS titled "Estimating Travel Times on the Toll Road with Segment-Based Data Imputation" aims to define travel times using two machine learning models, Long Short-Term Memory (LSTM) and Support Vector Regression (SVR), in different traffic conditions. The study provides valuable knowledge for researchers dealing with road safety. However, minor changes in the writing style could improve the paper.
In the introduction (line 31, page 1), the topics are described exhaustively. I suggest incorporating the section titled "Related Works" (line 71, page 2) into the introduction section as a sub-paragraph (1. Introduction; 1.1. Related Works).
I recommend incorporating the seventh section, "Effect of Missing Data on Model Performance" (line 485, page 22), as a sub-paragraph of the "Results" section (e.g., 6.4. Effect of Missing Data on Model Performance).
Finally, the "Discussion and Conclusion" section (line 501, page 23) accurately presents the results obtained in this study. It could be useful to expand future perspectives by suggesting other important variables that could be taken into consideration in future studies (line 532, page 23), such as features of the driving environment (e.g., urban vs rural roads, number of lanes, etc.).
Minor editing of English language is needed
Author Response
Response to Reviewer 5 Comments
Point 1: In the introduction (line 31, page 1), the topics are described exhaustively. I suggest incorporating the section titled "Related Works" (line 71, page 2) into the introduction section as a sub-paragraph (1. Introduction; 1.1. Related Works).
Response 1: Thank you for your suggestion regarding the organization of the manuscript. We appreciate your feedback. However, considering the current structure and coherence of the manuscript, it would be preferable to maintain the existing layout. This ensures that each section can provide a comprehensive and focused discussion of its respective topic.Point 2: I recommend incorporating the seventh section, "Effect of Missing Data on Model Performance" (line 485, page 22), as a sub-paragraph of the "Results" section (e.g., 6.4. Effect of Missing Data on Model Performance).
Response 2: We have revised the Results section and its sub-paragraph accordingly.Point 3: Finally, the "Discussion and Conclusion" section (line 501, page 23) accurately presents the results obtained in this study. It could be useful to expand future perspectives by suggesting other important variables that could be taken into consideration in future studies (line 532, page 23), such as features of the driving environment (e.g., urban vs rural roads, number of lanes, etc.).
Response 3: Thank you for your comment. In our revisions, we included discussion on these aspects and highlighted the potential directions for future studies in the last paragraph of Section 8. Point 4: Minor editing of English language is needed. Response 4: The revised manuscript has been proofread by a professional English editor (certificate attached).Author Response File: Author Response.pdf
Round 2
Reviewer 2 Report
The paper compares LSTM and SVR for estimating travel time on toll roads under diverse traffic conditions. The study focuses on Don Muang Tollway in Bangkok and addresses the issue of missing data through a segment-based imputation method. However, several aspects need attention and improvement.
1. In section 4.1, it provides detailed information on handling data loss, but due to the complexity of road segments, there may also be anomalous data that does not accurately reflect the road network status. It is recommended to conduct data cleaning to address anomalous data.
2. In Section 5.1, it mentions the rationale for choosing RBF as the kernel function and cites previous studies on travel time estimation as support. However, further details and explanations can enhance the understanding of the rationale behind the selection.
3. While MAPE serves as an evaluation metric that provides some information, it is not sufficient to comprehensively assess the performance of the methods. It is advisable for the authors to consider using other commonly used evaluation metrics, such as Mean Absolute Error (MAE) and Root Mean Square Error (RMSE), to supplement the evaluation of the estimation methods.
The paper compares LSTM and SVR for estimating travel time on toll roads under diverse traffic conditions. The study focuses on Don Muang Tollway in Bangkok and addresses the issue of missing data through a segment-based imputation method. However, several aspects need attention and improvement.
1. In section 4.1, it provides detailed information on handling data loss, but due to the complexity of road segments, there may also be anomalous data that does not accurately reflect the road network status. It is recommended to conduct data cleaning to address anomalous data.
2. In Section 5.1, it mentions the rationale for choosing RBF as the kernel function and cites previous studies on travel time estimation as support. However, further details and explanations can enhance the understanding of the rationale behind the selection.
3. While MAPE serves as an evaluation metric that provides some information, it is not sufficient to comprehensively assess the performance of the methods. It is advisable for the authors to consider using other commonly used evaluation metrics, such as Mean Absolute Error (MAE) and Root Mean Square Error (RMSE), to supplement the evaluation of the estimation methods.
Author Response
Please see the attachment.
Author Response File: Author Response.pdf
Round 3
Reviewer 2 Report
Although the manuscript has been modified accordingly compared to the original submision. There are several shortcoming as follows,
1. The input factors of SVM model or LSTM model is not clear, especially how samples were splited/processed as inputs in different time horizons? Although in Fig. 6, the results shown how the missing data had been imputated, the inputs consisted of several historical data set in previous time intervals were not well illustrated.
2. Some related works are suggested for reviewing and discussing.
Liu, Y., Xia, J. and Phatak, A., 2020. Evaluating the accuracy of bluetooth-based travel time on arterial roads: a case study of perth, western Australia. Journal of Advanced Transportation, 2020, pp.1-19.
Peng, L., Li, Z., Wang, C. and Sarkodie-Gyan, T., 2020. Evaluation of roadway spatial-temporal travel speed estimation using mapped low-frequency AVL probe data. Measurement, 165, p.108150.
Author Response
Please see the attachment.
Author Response File: Author Response.pdf