A Fine-Grained Ship-Radiated Noise Recognition System Using Deep Hybrid Neural Networks with Multi-Scale Features
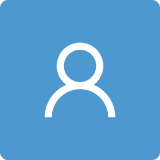
Round 1
Reviewer 1 Report
Comments for author File: Comments.pdf
Author Response
We would like to extend our sincere thanks and appreciation to you for your positive comments and helpful recommendations on our manuscript. Your expertise and attention to detail greatly contribute to the version of our work, and we are deeply grateful for your time and effort. All adjustments and improvements have been made to the revised paper according to your suggestions and comments. The attached document is our detailed responses.
Author Response File: Author Response.docx
Reviewer 2 Report
Technical Comments:
The introduction section is a nice one. It is architected very beautifully, while written fully academic and comprehend. I assume that any change in the introduction section is not necessary, but one of the important tasks after publishing a study is to increase its chance to be seen by the most possible number of researchers, so I would like to give two recommendations. First, to get your published study in the list of searched for papers based on keywords, I propose to increase variety of your keywords. In my viewpoint, they do not cover the whole topic of the study and are not widely searched words.
The study is comprehensive and requires large time to be read carefully and being reviewed. The theoretical background and also the method has been well explained in details, and the experiments and related models are well presented. However, I think more explanation about the optimization of the parameters are needed. Better explanation could result in a better understanding the whole procedure.
One of the methods in the publisher’s website that brings a publication on to the researchers, is based on the similar publications that they have read before. So, the more you cite similar publication, the more the chance that the search engine in the publisher website propose your paper to the researcher. Besides of that, it will also complete your introduction section. As another advantage, it rises new ideas to the researchers by combining various methods, or resolving drawback of one seen paper by reading the similar one, or extending the methodology to a fully automatic one. So, based on these points, I would like to ask to cite to the following similar publication in the manuscript: Khayer, et al. (2022). Target-Oriented Fusion of Attributes in Data Level for Salt Dome Geobody Delineation in Seismic Data. Natural resource research.
The authors should explain what limitations did they find out about the proposed method.
What about when the data are more complex with frequent type of noise? Please gives some explanation about the simplification of type of noises in your analysis, some explanation about the effect of various degree of simplifications and more importantly the effect of the image quality.
The abstract focusses mainly on the general problem and ignores the other items of the abstract such as the methodology, good introduction, results and conclusion.
Are the codes available for this research? As I found, there is no code available for this study, e. g. in Github. If the authors could make the codes available, the manuscript could be much better evaluated, not only for reviewers, but also for possible readers. When it is not possible to upload the code for public access, such as in Github, could they be provided for reviewer for better assessment of the study?
The result comparison parts are well organized and presented. The display way is good and the quantitative evaluation is well defined. But they are might be long, so I propose to make a brief summary of only quantitative comparison of results and methods in the conclusion section.
The authors should explain what limitations did they find out about the proposed method.
How did you evaluate the final result? How did you consider to finally selection a methodology for images with more ship’s diversity?
Does method work completely automatic? Is it in reasonable computation time? Please clear that if the method uses only a group of properties or attributes of image.
Will the method fail if the object boundary is not clear?
Best Regard
Author Response
We would like to express our sincere gratitude to your positive feedback on our manuscript. Your recognition of our research is greatly appreciated. We have carefully considered your comments and made the necessary revisions to improve the clarity and coherence of our paper. Your insightful suggestions have helped us to refine our work and make it more accessible to readers. Thank you again for your valuable suggestions, which has been instrumental in ensuring the quality of our manuscript. The attached document is our detailed responses.
Author Response File: Author Response.docx
Reviewer 3 Report
I looked to this paper from the point of an acoustician interested in real systems for acoustic ship classification. I have doubts about the usability of the suggested method. The paper does not reflect specifics of the ship signals and I cannot eliminate chances that the tested signals were taken in vary different conditions and their classification is based on the different background noise. I would suggest the following improvements that can show usability of developed method for acoustic ship classification.
1. The develop method uses three features that are connected with the acoustic signal amplitude and energy. The classification method does not use acoustic signal itself; it uses the electrical signals received by various hydrophones. These signals depend on the hydrophone gains and acquisition cards parameters. How do the used features took into account the parameters of hydrophones and the acquisition card.
2. I would like to see information about tested signals acoustic signatures: spectrograms of the signals, hydrophone gains, distances to ships, sea state and wind speed during measurements.
3. The usability of the method could be confirmed by the classification of the acoustic ship signatures mixed with the ambient noise. I would suggest to run the developed algorithm using noise mixed the acoustic signal for various levels of the noise. In this case the training has to be conducted with acoustic ship signal without noise. It could be interesting to find out noise level where the classification fails.
The suggested improvements may show usability of the developed methods for real signals, and I will be glad to review the improved manuscript.
Author Response
We would like to thank you for your careful reading, helpful comments, and constructive suggestions, which have significantly improved the presentation of our manuscript. We are dedicated to addressing your concerns and refining the applicability of our method. We apologize for not providing a detailed data description that caused confusion and it is provided below. We would like to clarify that the data used in our experiment were randomly selected and not specifically chosen based on the different background noise. The experiments have been conducted at various levels of noise to confirm the usability of our proposed method. We appreciate your insightful feedback and welcome any further suggestions or critiques you may have. Thank you once again for your invaluable contributions to this work. The attached document is our detailed responses.
Author Response File: Author Response.docx
Reviewer 4 Report
The paper proposed a fine-grained ship-radiated noise recognition scheme based on multiscale features using Modified Ensemble Empirical Mode Decomposition (MEEMD) and a combination of 1-D Convolutional Neural Network (1D CNN) and Long Short-Term Memory (LSTM) Network (MFAGNet). The method is evaluated and compared using the public DeepShip dataset showing improved performances to identify different specific ships. The paper is interesting and technically sound. However, the paper seems too long and out of focus. The authors need to accommodate the following revisions to improve the presentation.
1. Delete Figure 5 and add the references [1],[2]
[1]. van Houdt, G.; Mosquera, C.; Napoles, G. A review on the long short-term memory model. Artif. Intell. Rev. 2020, 53, 5929–5955.
[2]. Gers, F.A.; Schmidhuber, J.; Cummins, F. Learning to forget: Continual prediction with LSTM. Neural Comput. 2000, 12, 2451–2471.
2. Delete Tables 6,8,9,10,11,12. The use of all features (i.e. the six features) always provides the highest performance. So, it should be enough to say something like "Note that the results with any feature combination, are not shown here due to their lower performances than that obtained with all features."
3. Remove Section 4.2.2.
4. Move Section 2 (Data preprocessing) and 3.1.3 to Section 4 (Results and Discussion)
5. Figure 4 is not clear or well-presented. If it is not possible to improve then just delete it.
6. Equ. (23) is not correct.
Author Response
Thanks very much for the detailed review of our manuscript and your positive comments and suggestions. We are delighted that you consider our manuscript interesting and technical. We have revised accordingly and in detail by addressing each of the questions and points raised. The revised manuscript has undergone simplification to emphasize the key points. All adjustments and improvements have been made to the revised manuscript according to your suggestions and comments. The attached document is our detailed responses.
Author Response File: Author Response.docx
Round 2
Reviewer 3 Report
The paper was improved and I recommend it for publication.
Reviewer 4 Report
The paper is improved and revised accordingly.