Recurrent Neural Network-Based Multimodal Deep Learning for Estimating Missing Values in Healthcare
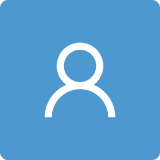
Round 1
Reviewer 1 Report
[Comment 1] Title
I suggest the authors include the "healthcare" term in the title because the paper focuses on the characteristics of healthcare data. Other applications might have different characteristics.
[Comment 2] Novelty
(End of Section 1) The authors must compare their study with previous studies here, then list the novelty (contribution) of their study. I suggest the authors provide the comparison in a table.
[Comment 3] Methodology
Between Tables 1 and 2, can the authors add another table showing which device measure which data type?
[Comment 4] Data and numerical experiments
[Subcomment 4a] I suggest the authors upload their data onto an online repository and share the link in their manuscript, to allow reproducibility by next researchers.
[Subcomment 4b] (Section 3.1) How do the authors deal with outlier data, e.g., data measured when the devices were not working properly? Please add such information as well.
[Subcomment 4c] To appropriately test the performance of the authors method when dealing with missing values, I suggest the authors conduct another experiment by purposely removing some values from a complete dataset to produce missing values, then try to reproduce those missing values with the proposed method, then measure the difference from the initial values. This way could be used to evaluate the proposed method well.
[Comment 5] Writing quality and clarity
[Subcomment 5a] For better understanding, please explain the contents of Figure 2. Some required explanations are: (1) what do the numbers mean below the "duplicate" word?, and (2) where are the missing values?
[Subcomment 5b] Please revise mistyped words, e.g., end of sentence, right above Table 6.
Author Response
Respond to Reviewers
We appreciated reviewer’s minor review. We revised the manuscript by addressing the comments of the reviewer.
Reviewer #1: Comments to the Author
[Comment 1] Title
I suggest the authors include the "healthcare" term in the title because the paper focuses on the characteristics of healthcare data. Other applications might have different characteristics.
Changes or rebuttal: We changes the title as the below.
- Recurrent Neural Network based Multi-modal Deep Learning for estimating Missing Values in Healthcare.
[Comment 2] Novelty
(End of Section 1) The authors must compare their study with previous studies here, then list the novelty (contribution) of their study. I suggest the authors provide the comparison in a table.
Table 1. RMSE of Number of Steps Estimation
Changes or rebutta: We added the contribution of the paper to Chapter 1. thank you
- Through the proposed method, it is possible to determine the direction of data integration in an environment where the types of wearable devices are diversifying and contribute to enabling continuous service to users. In addition, various studies to replace the missing values are in progress in academia, and the proposed method can be applied to the structure of an artificial neural room in a healthcare platform or to the application of machine learning techniques.
[Comment 3] Methodology
Between Tables 1 and 2, can the authors add another table showing which device measure which data type?
Changes or rebuttal: Thanks for the comment. Since there may be small differences depending on the product or the sensor used by the manufacturer, the device of the same company is provided to the user. However, in the case of a smartphone, due to the cost problem, it was not used separately, and the basic application included in the smartphone was used. Since we focused on finding missing values in the paper, we did not take into account the detailed specifications of the device or possible differences depending on the manufacturer.
thank you.
[Comment 4] Data and numerical experiments
[Subcomment 4a] I suggest the authors upload their data onto an online repository and share the link in their manuscript, to allow reproducibility by next researchers.
Changes or rebutta: It contains personal information and cannot be disclosed because the institution that conducted the actual verification does not agree to it.
[Subcomment 4b] (Section 3.1) How do the authors deal with outlier data, e.g., data measured when the devices were not working properly? Please add such information as well.
Changes or rebutta: The method proposed in this study handles problems from heterogeneous devices by replacing them with values predicted through RNN. This is described in Chapter 3.
[Subcomment 4c] To appropriately test the performance of the authors method when dealing with missing values, I suggest the authors conduct another experiment by purposely removing some values from a complete dataset to produce missing values, then try to reproduce those missing values with the proposed method, then measure the difference from the initial values. This way could be used to evaluate the proposed method well.
Changes or rebutta: This content was conducted through an experiment on sparse coding in Chapter 4.3.
[Comment 5] Writing quality and clarity
[Subcomment 5a] For better understanding, please explain the contents of Figure 2. Some required explanations are: (1) what do the numbers mean below the "duplicate" word?, and (2) where are the missing values?
Changes or rebutta: Duplicate is a variable that can be provided in common by multiple devices. In this situation, we conducted a study to check which device value the user should select and whether it is possible to compensate for missing values using duplicate values.
[Subcomment 5b] Please revise mistyped words, e.g., end of sentence, right above Table 6.
Changes or rebutta: Thank you. We fixed mistyped words.
Author Response File: Author Response.docx
Reviewer 2 Report
The manuscript proposes a technique to estimate missing values and omit redundant values coming from heterogeneous health platforms. Authors claimed to achieve higher accuracy than singular value decomposition while using RNN but with the cost of excessive computation and higher turnaround time. Later authors used sparse coding but that resulted in losing quite a bit of accuracy. In my opinion, the manuscript is suitable for publication in the “Applied Sciences” journal only after resolving the issues given below.
Major points:
1. A summary of the result in one or two sentences should be included at the end of the abstract. The abstract needs to be more organized and should be shortened without losing key points.
2. In related work (section 2), there must be a subsection discussing the literature survey related to the use of RNN for estimating missing values or omitting redundant values. There has been a significant amount of work done on these topics. Please go through those and point out the prominent ones with their contributions and limitations.
3. There must be an explanation regarding how the author’s proposed technique is an improvement over the existing technique.
4. Since the author proposed a technique with RNN, so authors are requested to cut down their discussion with the fuzzy model, ANFIS, or ANN.
5. In subsection 2.2, the authors have discussed various datasets. Unless authors have used one of those datasets (which I assume they have not), they could have just used reference and kept that discussion short.
6. Authors are requested to recheck the manuscript for redundant information (using the same information with rephasing and using it in different places) related to heterogeneous health and multimodal platform.
7. More detailed information needs to be placed about 26 patients. For example: do they have any preexisting conditions, how their age varies or means, etc.
8. What kind of preprocessing was done on the raw data from different devices to make sure they are free from noise and artifacts?
9. How authors have made sure about removing bias from the measurement from different types of devices. Also, how the reliability of those devices was ensured?
10. Do authors have created algorithm 1 (selection algorithm) by themselves or has it’s been taken from some other place?
11. Please check for redundant information at the beginning of 3.3 (first paragraph).
12. “According to an experiment” in section 3.3 – here need more details about the experiment.
13. Have authors tried to test their technique using another dataset?
14. Authors need to use another deep learning technique such as ANN to include in table 6. Their proposed technique should not be the only deep learning technique when they are comparing their performance.
15. “For this reason, the model that had a higher accuracy rate than the other estimation models and achieved the shortest turnaround time was selected.”- compare that with other existing deep learning-based missing value estimation techniques available in the literature.
Author Response
Respond to Reviewers
We appreciated reviewer’s minor review. We revised the manuscript by addressing the comments of the reviewer.
Reviewer #2: Comments to the Author
The manuscript proposes a technique to estimate missing values and omit redundant values coming from heterogeneous health platforms. Authors claimed to achieve higher accuracy than singular value decomposition while using RNN but with the cost of excessive computation and higher turnaround time. Later authors used sparse coding but that resulted in losing quite a bit of accuracy. In my opinion, the manuscript is suitable for publication in the “Applied Sciences” journal only after resolving the issues given below.
Major points:
- A summary of the result in one or two sentences should be included at the end of the abstract. The abstract needs to be more organized and should be shortened without losing key points.
Changes or rebutta: We added information about the experimental results to the summary. thank you
- According to the evaluation in terms of representative value selection, when a representative value was selected by using the mean or median, the most stable service was achieved. As a result of the evaluation according to the estimation method, the accuracy of the RNN-based multi-modal deep learning method is 3.91%p higher than that of the SVD method.
- In related work (section 2), there must be a subsection discussing the literature survey related to the use of RNN for estimating missing values or omitting redundant values. There has been a significant amount of work done on these topics. Please go through those and point out the prominent ones with their contributions and limitations.
Changes or rebutta: Our research goal was to process data in a heterogeneous device environment. A study was conducted on how to handle missing values in the future, and as a separate study, related contents were omitted from this paper. thank you.
- There must be an explanation regarding how the author’s proposed technique is an improvement over the existing technique.
Changes or rebutta: The purpose of the study was added in the introduction section.
- In a situation where various devices have been developed and distributed to people, people may feel confused due to overlapping information, and accordingly, research on a method for integrated management of information is needed.
- Since the author proposed a technique with RNN, so authors are requested to cut down their discussion with the fuzzy model, ANFIS, or ANN.
Changes or rebutta: We proposed a technique with RNN, but confirmed that the problem posed by artificial neural networks can be solved. We plan to explore different and possibly better ways to resolve missing values in the future. And also added in Chapter 3.1 why we use RNNs.
- The healthcare data appears continuously over time, and accordingly, RNN, which shows an advantage for continuity among various artificial neural networks, is used.
- In subsection 2.2, the authors have discussed various datasets. Unless authors have used one of those datasets (which I assume they have not), they could have just used reference and kept that discussion short.
Changes or rebutta: Our study added to current healthcare data to discuss issues that may arise with the healthcare platform. In our discussion, we wanted to leverage data collected from different devices. The healthcare data set in 2.2 was not appropriate because it had different observations.
- Authors are requested to recheck the manuscript for redundant information (using the same information with rephasing and using it in different places) related to heterogeneous health and multimodal platform.
Changes or rebutta: The method of processing data collected from individual devices in the healthcare platform has been conducted in several existing studies, but the process of integrating data collected from different devices and raising and solving problems that occur in the process of processing it has been I think it's different from research.
- More detailed information needs to be placed about 26 patients. For example: do they have any preexisting conditions, how their age varies or means, etc.
Changes or rebutta: We have modified that part as follows.
- This is the data of 26 people, including 10 men in their 20s, 5 women in their 20s, 7 men in their 30s and 3 women in their 30s who are interested in health care, 10 sleeping mats, 6 smartwatches, 17 health bands, 26 smartphones, and blood glucose meters. It is health care empirical data collected for 300 days using 11 sphygmomanometers, 11 sphygmomanometers, and mobile applications.
- What kind of preprocessing was done on the raw data from different devices to make sure they are free from noise and artifacts?
Changes or rebutta: A study was conducted on how to deal with noise and artifacts occurring in a heterogeneous environment. I think it's a different study than how to deal with noise and artifacts for one device.
- How authors have made sure about removing bias from the measurement from different types of devices. Also, how the reliability of those devices was ensured?
Changes or rebutta: With the development of IT technology, many companies are developing and selling healthcare devices. We conducted a study on data duplication that may occur accordingly, and the evaluation of the reliability of the device should be conducted as a separate study.
- Do authors have created algorithm 1 (selection algorithm) by themselves or has it’s been taken from some other place?
Changes or rebutta: Algorithm 1 was arbitrarily written to solve the problem raised in the paper.
- Please check for redundant information at the beginning of 3.3 (first paragraph).
Changes or rebutta: We have deleted the duplicates below.
- The variables that influence human health are diverse. They include temperature, humidity, weather, fine dust, nutrition contents, activity, and family history. According to the prediction target, it is necessary to design diverse variables. For this reason, soft computing cannot easily predict an answer accurately [1,2,11-14]. The platform utilizes users’ surrounding circumstances and activity for generating daily healthcare predictions.
- “According to an experiment” in section 3.3 – here need more details about the experiment.
Changes or rebutta: We have added below.
- As a result of learning by changing the number of hidden layers in the proposed network, when the number of hidden layers is small, it appears sensitive to missing values, and when the number of hidden layers increases, it appears that excessive learning is required.
- Have authors tried to test their technique using another dataset?
Changes or rebutta: We would like to check in other datasets, but there are difficulties because there is not enough healthcare data collected in heterogeneous situations. We plan to expand the demonstration in the future.
- Authors need to use another deep learning technique such as ANN to include in table 6. Their proposed technique should not be the only deep learning technique when they are comparing their performance.
Changes or rebutta: Our experiment was an attempt at how to deal with data duplication and missing values in a heterogeneous environment. Since the method of simply handling missing values is covered in other papers, it will be conducted as a separate study. In addition, the proposed structure does not separate RNN and ANN separately in a combined form of RNN and ANN.
- “For this reason, the model that had a higher accuracy rate than the other estimation models and achieved the shortest turnaround time was selected.”- compare that with other existing deep learning-based missing value estimation techniques available in the literature.
Changes or rebutta: Existing studies are mostly focused on estimating missing values. We aimed to find solutions to problems that may arise in the healthcare platform.
Author Response File: Author Response.docx
Round 2
Reviewer 1 Report
[Comment 1] Novelty
The authors have not responded to my previous comment:
"The authors must compare their study with previous studies here, then list the novelty (contribution) of their study. I suggest the authors provide the comparison in a table."
The reason why I asked for such a table is so the authors could compare their paper with specific previous studies along with the clear explanations about the different aspects, e.g., instead of only writing "carious studies to replace the missing values are in progress", the authors need to list the studies, and show how this paper covers the research gap unfilled by those existing studies.
[Comment 2] Writing quality and clarity
The authors responded for my previous subcomment 5a as follows:
“Duplicate is a variable that can be provided in common by multiple devices. In this situation, we conducted a study to check which device value the user should select and whether it is possible to compensate for missing values using duplicate values.”
Such explanation should be added in the text. It is still unclear about what the numbers below “duplicate” represent. Please give an example in the text for clarity. Please give an example of the missing value case based on Figure 2 as well.
Author Response
We appreciated reviewer’s minor review. We revised the manuscript by addressing the comments of the reviewer.
Reviewer #1: Comments to the Author
[Comment 1] Novelty
The authors have not responded to my previous comment:
"The authors must compare their study with previous studies here, then list the novelty (contribution) of their study. I suggest the authors provide the comparison in a table."
The reason why I asked for such a table is so the authors could compare their paper with specific previous studies along with the clear explanations about the different aspects, e.g., instead of only writing "carious studies to replace the missing values are in progress", the authors need to list the studies, and show how this paper covers the research gap unfilled by those existing studies.
Changes or rebuttal: We tried to write it as a table as you suggested, but it lacks expressive power. Thus we added the list of contributions instead to convey clear words. thank you
The contributions of the proposed method are as follows:
- It is possible to determine the direction of data integration in an environment where the types of wearable devices are diversifying and contribute to enabling continuous service to users.
- This is a method for dealing with the data duplication that occurs in a heterogeneous healthcare environment.
- Previous studies on the imputation of missing values were conducted on one device or one data set Previous studies on the imputation of missing values were conducted on one device or one data set in healthcare.
- The proposed method is more suitable for healthcare environments by the imputation of missing values in a structure where different devices complement each other.
- The proposed method can flexibly integrate the data collected in the perfume healthcare environment.
This study consists of the following: Section 2 describes the research trends of soft computing and the data characteristics in heterogeneous health platforms. Section 3 de-scribes the proposed RNN based multi-modal deep learning for estimating missing values in healthcare. Section 4 describes the experimental results and performance evaluation. Finally, Section 5 draws conclusions.
[Comment 2] Writing quality and clarity
The authors responded for my previous subcomment 5a as follows:
“The duplicate is a variable that can be provided in common by multiple devices. In this situation, we conducted a study to check which device value the user should select and whether it is possible to compensate for missing values using duplicate values.”
Such explanation should be added in the text. It is still unclear about what the numbers below “duplicate” represent. Please give an example in the text for clarity. Please give an example of the missing value case based on Figure 2 as well.
Changes or rebuttal: Thank you. We added it to Chapter 3.
- The duplication is a variable that can be provided in common by multiple devices. In Figure 2, heart rate is collected from a smart band, heart rate monitor, smartwatch, and smartphone to represent the four duplications.
Reviewer 2 Report
The authors need to clarify the following points as their responses to points number 14 and 15 raised more questions than answers. Authors are requested to provide answers with rationale rather than only stating their actions.
Major issues:
1. “Several missing value estimation methods exist, including collaborative filtering (CF), regression model (RM), k-nearest neighbor (KNN), and deep learning (DL) [10,31]. The missing value estimation method proposed in this study uses RNN-based multimodal deep learning (RMD). These methods were compared in terms of accuracy and turnaround time” – Authors mentioned these at the beginning of section 4.2.
The authors need to clarify several issues regarding this section:
a. So, since they have chosen algorithms (for comparison) those deal with missing value estimation, why do they think CF, RM and KNN are sufficient to compare their result with. Since they have mentioned that “Several missing value estimation methods exist”.
b. Authors have chosen an RNN-based multi-modal deep learning (RMD) technique, but they have not either experimented with using ANN or compared it with any other deep learning technique. They need to either provide results using ANN or clearly explain the rationale behind their choice of avoiding doing that.
The authors have replied to the queries but their response to point number 14 and 15 need further clarification before the manuscript become suitable for acceptance.
Author Response
We appreciated reviewer’s minor review. We revised the manuscript by addressing the comments of the reviewer.
Reviewer #2: Comments to the Author
The authors need to clarify the following points as their responses to points number 14 and 15 raised more questions than answers. Authors are requested to provide answers with rationale rather than only stating their actions.
Major issues:
- “Several missing value estimation methods exist, including collaborative filtering (CF), regression model (RM), k-nearest neighbor (KNN), and deep learning (DL) [10,31]. The missing value estimation method proposed in this study uses RNN-based multimodal deep learning (RMD). These methods were compared in terms of accuracy and turnaround time” – Authors mentioned these at the beginning of section 4.2.
The authors need to clarify several issues regarding this section:
- So, since they have chosen algorithms (for comparison) those deal with missing value estimation, why do they think CF, RM and KNN are sufficient to compare their result with. Since they have mentioned that “Several missing value estimation methods exist”.
Changes or rebutta: There are numerous studies based on CF, RM, and KNN, but compared with traditional methods to know the experimental results more intuitively. We have added the following to Chapter 4.2. Thank you.
- CF, RM, and KNN are widely used as traditional prediction methods commonly used in data mining. A large amount of data is required to train a deep learning model, and in the real world, data analysts or companies mainly use traditional methods before data is obtained for this situation. The traditional method does not require a long learning time like deep learning and has an acceptable range of error. In addition, it is widely used in real environments.
- Authors have chosen an RNN-based multi-modal deep learning (RMD) technique, but they have not either experimented with using ANN or compared it with any other deep learning technique. They need to either provide results using ANN or clearly explain the rationale behind their choice of avoiding doing that.
The authors have replied to the queries but their response to point number 14 and 15 need further clarification before the manuscript become suitable for acceptance.
Changes or rebutta: We further described the reasons for using RNNs and DNNs as neural networks. We added the following to Chapter 2.2. and Chapter 3.
Chapter 2.2.
- ANN is classified into DNN, CNN, and RNN according to the characteristics of the neural network, and RNN is most suitable for healthcare data with time-series features. When the variables show the time-series feature, most frequently, RNNs are used to predict a change in the current state of healthcare [17]. Each variable is collected in a different cycle, and therefore, a basic time step is ambiguous in RNN learning. Therefore, the variables not collected in the same cycle learn the RNN separately.
Chapter 3.
- To reflect time-series characteristics, the predictive model for each variable is learned with RNN, which is an ANN structure that can reflect the passage of time, and the cell uses GRU at this time. The correlation between each variable is used as a DNN structure emphasizing interconnectivity in ANN.
Round 3
Reviewer 1 Report
Thank you for your revisions.
Reviewer 2 Report
Authors have answered satisfactorily to all queries .