Applying Fuzzy Inference and Machine Learning Methods for Prediction with a Small Dataset: A Case Study for Predicting the Consequences of Oil Spills on a Ground Environment
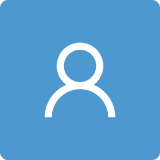
Round 1
Reviewer 1 Report
Why the authors worked on synthetic data? On page 18, last para, the small description was given but it is not very clear to me.
Did the authors tested their proposed system on real data?
What about the oil spills in sea or any other water body?
Author Response
Please, see the response to the reviewer's comments in the attached file.
Author Response File: Author Response.docx
Reviewer 2 Report
The paper is well written, and highly readable. The contents and their connectiosn are well established.
The application of FIS in this case is quite well established.
Author Response
Please, see the response to the reviewer's comments in the attached file.
Author Response File: Author Response.docx
Reviewer 3 Report
This paper is original, well written, and experiments are well designed. I only have a few minor comments/questions below:
1. In Table 6, the Ensembles didn't perform as good as linear regressors or decision trees in NMRSE, which in a normal sense ensembles should perform better considering the structure of the algorithm. I'm wondering which ensemble method has been used? It may be better specify the ensemble algorithm type in the table and show the results. Also, it may deserve to discuss a bit why the ensembles applied here didn't perform better than DT/LR.
2. Since the current study is based on a small dataset of oil spills, to extend the impact of this research, it may deserve to discuss a bit how this ANFIS algorithm can generalize to other datasets of same problem in the conclusion or methodology part -- even though data could be a limitation, what's the capability of the algorithm handling similar problems.
3. In terms of the writing, suggest to align the formats of equations in terms of font sizes and shapes (e.g., Eq(8), Eq(9), Eq(19) - Eq(23))
Author Response
Please, see the response to the reviewer's comments in the attached file.
Author Response File: Author Response.docx
Reviewer 4 Report
Primarily the article has attempted adequately and is easy to ponder. However, I request that authors should consider the broad audiences including domain experts and novice acquaintances in the area of an article. Therefore, kindly follow the highlighted points and incorporate them into the article.
1. What threshold has been used for tree impurity to select the variables? And what thresholds have been used for R-square and RMSE to set models' robustness.
2. The authors should rewrite the sentence “The best result showed GPR with the highest prediction accuracy among other ana-391 lysed algorithms” – in regression we test models based on R-square and RMSE/MSE measures rather than accuracies.
3. Overall, the paper is well written but lacks some rationales such as why ML techniques are suitable for identified problems, what are their advantages, why the regression task is suitable for this solution, and what the dependent variable has been predicted (clearly answer it).
4. If the authors did perform the cross-validation, considering small data in hand, please mention this.
I highly appreciate the author's time and efforts for this article and submission to this venue. Kindly, try to incorporate the highlighted suggestions, this will foster your effort to make this work a success. I am looking forward to the revised copy.
Author Response
Please, see the response to the reviewer's comments in the attached file.
Author Response File: Author Response.docx
Reviewer 5 Report
The article contains several technical errors, some of which are:
· The authors mix the different correlation calculation methods and calculate them all for all data types. This is incorrect, as each method can be applied to different data types. Moreover, the data in Table 3 are most certainly incorrect. Certainly, the r values cannot be equal to 0 in so many cases.
· Furthermore, the chi2 method has application conditions, which are not mentioned by the authors. This is particularly critical for small data sets!
· introduction the decision tree models (lines 294-299): The decision tree can contain not only binary cuts, so the introduction of this type of model is wrong (there are no left and right children). Furthermore, data are not divided into features, as it is written in the text. Additionally, other termination criteria can also be applied, and the recursive partitioning process does not stop only then when homogeneous partitions are resulting.
· The statement “Ensembles [75] combine several ML tree algorithms” is not true. These methods can work not only with trees.
· The manuscript does not present the parameter settings of the different machine larning methods, so the results can not be evaluated.
Other major comments:
· The contributions of the paper are not correctly determined. Some of them overlap with each other, while some of them are not fundamental contributions. For example, the third element of the list is only a generally applicable technique which was also applied in this study. I would not mean this as a contribution. Please think again about the main contributions of this article.
· The article completely fails to present the research on predicting the oil spills on the ground environment. As this is the main focus of the article, the related literature should be introduced in detail.
· Authors have to clarify how many data were generated by the mathematical model.
Minor comments:
· Equation (2): Please introduce the notation f_i related to Equation (2).
· Equation (3) is wrong. Please correct it.
· Equations 1-4: Please standardize the notations used (N vs. n) and give the meaning of each.
· Table 1 summarizes some problems that were investigated related to small datasets. This table is interesting, however, it does not contain the size of the datasets used. Furthermore, the number of features of the dataset would also be interesting for the users.
· The method presented in Figure 1 needs to be presented in detail in the text before the detailed information is given from each step later.
Author Response
Please, see the response to the reviewer's comments in the attached file.
Author Response File: Author Response.docx
Round 2
Reviewer 4 Report
Highly appreciate your revision for this manuscript. Thank you.
Author Response
Thank you for your comments, which help us to improve our paper and race its quality.
Reviewer 5 Report
- The manuscript still contains some technical errors. Please consider that the Pearson correlation coefficient should be used to determine the linear relationship between two continuous variables (e.g., Spilled oil volume; air temperature, ground moisture, etc.), and the Spearman coefficient and the Kendall tau measure the monotonic relation between two rank-ordered variables (in different ways). Between the nominal attributes, the chi-squared correlation coefficient has to be calculated. Please see, for example, https://medium.com/@outside2SDs/an-overview-of-correlation-measures-between-categorical-and-continuous-variables-4c7f85610365. Therefore, I do not understand the caption of Table 3. Please clearly clarify the data types in Table 2, and use the appropriate method to calculate the coefficients between the variables.
- Additionally, the results presented in Table 3 are uninformative. Please give the precise values of the coefficients.
- Statement in line 306 is not valid! “Consequently, the data is divided into features”. Data is divided into subsets but not into features.
- Line 260: Replace the decimal comma with a decimal point.
- Equations (8), (9), (17), (18) contain extra “?” character.
- Please correct the size of the equation (19)
- Line 287 vs Equation (11). Different epsilon notations were used.
Author Response
Thank you for your comments, which help us to improve our paper and race its quality.Author Response File: Author Response.docx