Hard Disk Failure Prediction Based on Blending Ensemble Learning
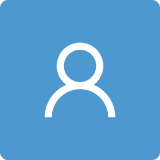
Round 1
Reviewer 1 Report
1-Missing literature review, shall add at least 8 papers and compare between them as a table.
2- The method used in the manuscript shall clearly describe the parameter used for every five methods.
3- the contribution is not clear.
4- result needs to be improved.
5- How the authors used TP, TN, FP, and FN what is the reason? what is TP mean in your case? as well as the other confusion matrix in your case?
6- the conclusion is missing.
7- needed to update the references.
Author Response
Thank you very much for taking time out of your busy schedule to put forward valuable suggestions for my article.
Please see the attachment
Author Response File: Author Response.pdf
Reviewer 2 Report
Reply to reviewers
I appreciate the authors attempting the hard disk failure prediction based on ensemble learning.
· Fig. 5 is not clear. It is a table not figures. Modify the table and don’t paste the content instead mention reworked after corresponding citation.
· In table 1 mention the reference.
· In section 4.2. how data preprocessing has been done? In what basis classification has been done? Any algorithm or special techniques applied in failure prediction.
· In BP neural network model- Why there are lot of mismatches in Fig. 11 and Fig. 12.? What are the parameters used in applying BPNN?
· Blending ensemble learning is an interesting topic of study and it is a fruitful approach, but authors have to address the mathematical part of blending learning in details mentioned in fig. 4.
· Pseudo code can be provided to make the readers to understand the logic evidently.
Comments for author File: Comments.pdf
Author Response
Thank you very much for taking time out of your busy schedule to put forward valuable suggestions for my article.
Please see the attachment
Author Response File: Author Response.pdf
Round 2
Reviewer 1 Report
Still, the methodology is not transparent. How can predict without any delay time for prediction (shall get a delay time to train the model and based on the training get to expect for next time, for example shall delay the input for 2T then train the model to get the third time as predict)
Author Response
Thank you very much for taking time out of your busy schedule to put forward valuable suggestions for my article again.
Please see the attachment
Author Response File: Author Response.pdf