Online Joint Optimization of Virtual Network Function Deployment and Trajectory Planning for Virtualized Service Provision in Multiple-Unmanned-Aerial-Vehicle Mobile-Edge Networks
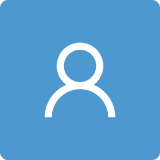
Round 1
Reviewer 1 Report
Comments and Suggestions for AuthorsThis paper aimed to maximize the accepted requests’ number meanwhile minimizing both the energy consumption and the cost of accepting requests with the constraints of UAVs’ resources and real-time requests’ latency requirement. To solve this problem, a DRL-based approach is proposed. The reviewer thinks the paper is interesting. However, some concerns need to be addressed:
1. How can ensure that the 16 constraints are met based on the use of reinforcement learning?
2. Related work is insufficient, and some work on reinforcement learning, UAV, and VNFs has been missed, such as.
[1] https://ieeexplore.ieee.org/abstract/document/10032267
[2] https://ieeexplore.ieee.org/abstract/document/8432464
[3] https://ieeexplore.ieee.org/abstract/document/8845184
3. It would be good to show or introduce the advantages of your online DRL-based approach.
4. It would be good to introduce the multi-agent process.
5. Please check the full paper. There are many spelling and grammatical errors, and please adjust the format of the article (especially the figures).
Comments on the Quality of English LanguagePlease check the full text, there are many spelling and grammatical errors, and please adjust the format of the article (especially the figures).
Author Response
Please see the attachment.
Author Response File: Author Response.pdf
Reviewer 2 Report
Comments and Suggestions for AuthorsBased on the content of the paper titled "Online Joint Optimization of VNFs deployment and Trajectory Planning for Virtualized Service Provision in Multi-UAVs Mobile Edge Networks," here are some minor revisions comments:
· The paper presents a complex topic involving UAVs, VNFs, and DRL. Ensure each section clearly defines and explains the concepts and terminologies used.
· While the paper provides a comprehensive review of related work, it would be beneficial to include a more explicit discussion on how this work extends or diverges from existing research.
· The proposed DRL approach is intriguing, but the paper could provide more specifics on the neural network architectures, hyperparameters, and the training process.
· The performance evaluation is well-executed, but additional simulations comparing the proposed approach with more baseline methods could offer a more robust validation of the algorithm's effectiveness.
· While the simulations provide insights into the algorithm's performance, a section discussing the potential challenges and considerations for real-world implementation would be beneficial.
- Main Research Question: The research addresses the challenge of optimizing the deployment of Virtual Network Functions (VNFs) and trajectory planning of Unmanned Aerial Vehicles (UAVs) in multi-UAV mobile edge networks to maximize service provision to ground users while minimizing energy consumption and operational costs.
- Originality and Relevance: The manuscript introduces an innovative approach by jointly optimizing discrete (VNF deployment) and continuous (UAV trajectory planning) actions using an improved online Deep Reinforcement Learning (DRL) scheme. T.
- Contribution to the Field: The proposed method advances the field by addressing the computational complexity and real-time operational challenges of VNF deployment and UAV trajectory planning in a unified framework.
- Methodological Improvements: The authors should consider providing more detailed information on the DRL algorithm, including the architecture of the neural networks, the specific reinforcement learning algorithms used, and the parameter settings. Including a sensitivity analysis of the key parameters would also strengthen the methodology section.
- Consistency of Conclusions: The conclusions are broadly consistent with the evidence and arguments presented; however, the manuscript would benefit from a more detailed discussion on the limitations of the proposed approach and how it compares to existing methods in terms of scalability, adaptability to dynamic environments, and real-world feasibility.
- References Appropriateness: The references appear to be appropriate and relevant, covering key areas of UAV networks, VNF deployment, and reinforcement learning.
- Tables, Figures, and Data Quality: The quality of the tables and figures is generally good, providing clear visual representations of the proposed architecture, system model, and results.
Author Response
Please see the attachment.
Author Response File: Author Response.pdf
Round 2
Reviewer 1 Report
Comments and Suggestions for AuthorsThanks for the response letter, the paper is good and can be accepted now.
Comments on the Quality of English LanguageThe writing is clear and good now.