Transition to Multicellularity and Peto Paradox
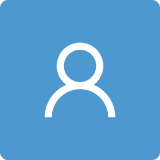
Round 1
Reviewer 1 Report
Comments and Suggestions for AuthorsThe paper "Transition to Multicellularity and the Peto Paradox" is an effort to explain the response to stress within an evolutionary framework. It utilizes aspects related to the multi-Lotka-Volterra equation, stochastic consideration of parameters, and, more generically, the Cauchy problem.
From a mathematical point of view, it is well-adapted to the journal. It is coherent and rigorous, deserving publication.
I have two suggestions for future work:
1. Spatial considerations are imperative, especially with regard to reaction-diffusion processes. Cells are spatial objects developing in two or three dimensions.
2. A biologist, an expert in the area, should be consulted by the author to ensure realistic results.
In summary, the manuscript deserves publication in Mathematics.
Author Response
I am grateful to Referees for useful comments and remarks. The major correction is
a subsection “Innovations” added according to Major Comment 1 in Report 3.
Moreover, I looked through manuscript again and corrected some misprints and also made minor
improvements in presentation.
ANSWER TO Report 1
Indeed, it would be interesting to extend this approach on reaction-diffusion systems. I have some results in this direction, but they do not seem very impressive to me, and to simplify the presentation, I decided to limit myself to simpler models.
Reviewer 2 Report
Comments and Suggestions for AuthorsThe author constructs a three-stage mathematical model aiming to describe the observation that cancer anticorrelates with the number of cells in organisms (Peto paradox). The model is, in its essence, stochastic and does not rely on any particular parametrisation. The three main ingredients are kinetics mechanisms, eventually compartmental, a genetic regulatory network and stochastic evolutionary dynamics. The introduction and the model construction are very well explained. In section 9, the author explains the approach to the Peto paradox and simulates the probability that cancer does not emerge as a function of the number of cells. Finally a discussion on the evolution of complex trails. Technical details are described in several appendices.
This paper is interesting from the mathematical and biological point of view.
Author Response
ANSWER TO REFEREES Comments
I am grateful to Referees for useful comments and remarks. The major correction is
a subsection “Innovations” added according to Major Comment 1 in Report 3.
Moreover, I looked through manuscript again and corrected some misprints and also made minor
improvements in presentation.
ANSWER TO Report 2
Thank you for this good estimate of my work!
Reviewer 3 Report
Comments and Suggestions for AuthorsMy comments are in the attached file.
Comments for author File: Comments.pdf
The writing is fluent but document should be edited and checked.
Author Response
ANSWER TO REFEREES Comments
I am grateful to Referees for useful comments and remarks. The major correction is
a subsection “Innovations” added according to Major Comment 1 in Report 3.
Moreover, I looked through manuscript again and corrected some misprints and also made minor
improvements in presentation.
ANSWER TO Report 3.
The manuscript is modified along Referee’s propositions.
Major comments
Comment 1: The author should include a new paragraph listing the innovative aspects of the paper and the strengthen the main differences relative to other references, specifically Vakulenko and Grigoriev (2021).
THANK YOU FOR THIS QUESTION!! Of course I thought about this question but apparently I didn’t write it in enough detail.
The new paragraph:
This article continues the series of works (see, for example, Vakulenko and Grigoriev,2006, Vakulenko and Grigoriev, 2021), where the idea of replicative stability is
developed proposed by M. Gromov and A. Carbone (Gromov and Carbone). It was
shown that some properties of evolution could be explained on the basis that biosystems
must maintain homeostasis under the influence of stress and fluctuations (both internal
and external), and this homeostasis support must be done by subsequent replications. In129
deed, many features of evolution can be explained in this way, for example, the tendency
to increase the number of genes (see a review in (Vakulenko, 2014)). The key question,
however, is to explain complexity of genetic networks. An attempt to resolve this prob132
lem was made in (Vakulenko and Grigoriev, 2021), where a model of the response of
the biochemical system to stress is introduced. In this work, the entropy of a stressful
environment is defined and it is shown that an effective response to stress is possible if
the binary logarithm of the size of the regulatory genetic network is not less than this entropy. Therefore, according to (Vakulenko and Grigoriev, 2021) the main reason, why
regulatory networks grow, is complexity and diversity of stress environment.
In this paper, the main innovation with respect to these works, is that, in order
to describe a response to stresses, compartment model is used. It allows us to take into account the organism modular structure and stem cell activity. The organism
response to stress goes via populations of cells in the compartments, and dynamics of
these populations is determined by the standard Lotka-Volterra model. Such approach
permits to advance the one of key biological problems, the Peto paradox, because it will
be shown that then the organism size directly affects stress response mechanisms. The
second innovation with respect to (Vakulenko and Grigoriev,2021) is that we no longer
need the complexity of the environment to explain the complexity of an organism’s
genetic network. If the organismenvironment fluctuates, then this is enough to initiate an
increase in the complexity of the genetic network. At last, the third intriguing innovative
element is that when an organismis divided into compartments, there is no longer a need
to fine-tune genetic regulatory networks (as in connectionist models (Mjolsness et al.)).
Instead, it is enough to adjust an appropriate interaction between the compartments.
Minor comments
Comment 1. All minor changes have made according to Referee remarks.
The most of these errors are trivial misprints, for example, instead of $N_{cell}$ was $M_{cell}$ etc.
At line 310 in the sentence “Of course the model from are toy …” . This sentence does not contain a reference to the works cited above. This is modified so:
“The models from [Vakulenko, Grigoriev 2022] and [Sudakov et al. ] look as toy ones,
nonetheless one can suppose that this conclusion on the number of coding genes
is valid for more realistic models”.
after Eq. (52) - explain the meaning of Mcell ;
after Eq. (54) - explain the meaninng of Prc,mod ;
Here should be N_{cell} and P_{c, mod}.
Comment 2 I suggest editing the inline references. Examples: Koonin (2011, p. 250), Ventsel and Freidlin (1970).
I changed the style of references, unfortunately, I am not so familiar with these things.
Comment 3 I suggest replacing the transpose operator “tr” by the one that commonly used in Mathematics - “⊺” - all over the manuscript.
Fixed.
Comment 4: page 4 - consider “... for all t ∈ (−∞, +∞) ...”. I see no reason for using the physically consistent interval t ∈ [0, +∞). In case the author wants to emphasize the generalization of the representation I suggest to include a note to explicitly clarify the assumption.
Comment 5: line 136 - comment the reason for having t > 0 and not t ≥ 0. I agree that vi (0) is not exactly a solution; it is an initial condition, but on the other hand it must also conform to the conditions of the problem. Please check.
I changed the interval: t ∈ (−∞, +∞) is replaced on t ∈ [0, +∞).
Comment 6 please provide more details on the function χ(v) and how it is modeled every-
where. It seems to be an heavy-side function holding in distinct domains.
The old text:
where $\chi(v)=0$ outside of an open neighborhood W_{delta} of an equilibrium, which is a subset a
homeostasis domain
This text is modified so:
where $\chi(v)=0$ is defined as follows. The $\chi$ is a Heaviside-like function,
which is equal to 0 outside of an open neighborhood W_{\delta} of an equilibrium and
$\chi(v)$ is equal to 1 inside this neighborhood. Here we assume that the neighborhood radius $\delta$ is so small that this neighborhood $W_{\delta}$ lies within the homeostasis domain.
Comment 7. please, provide some reasoning for ∆τ >> ∆t.
In this small paragraph I have changed the bad notation and slightly changed the presentation.
Now it looks so:
To describe the trend h(t), we use the model of subsequent environmental shocks.
We assume that h(t) are piecewise constant functions. Let t_1, t_2, ..., t_k, ... be an infinite
increasing sequence of time moments t_j. Let Δτ = min (t_{j+1} - t_j). We suppose
Δτ >> Δtr and Δτ >> Δtd , where Δtr Δtd are characteristic times of gene regulation
functioning and biochemical model dynamics, respectively. At the moments tj we have
1environment shocks, when an interaction between the environment and the biosystem
changes. The assumptions on Δτ, Δtr Δtd are natural. It means that changes in the
1organism environment are not very frequent and, therefore, organisms have time to react
to them. Indeed, otherwise it is difficult to expect that evolution could be successful.
Comment 8 I suggest including a comment on the dependence (or independence) of
ith reactant on the others. That is, comment the models’ structure regarding the coupling / decoupling
Within the framework of this weakly nonlinear approximation, all information about the interaction (coupling-decoupling) of reactants is contained in the matrix $A$. We can define
the coupling graph $(V, E)$, where the set $V$ of vertices is the set of reactants, and an edge $e={i,j}$ lies in the edge set $ E$ if $i$-th reactant interacts with $j$-th one. One can consider various cases of interaction of reactants that are relevant from a biological point of view. For example, we can consider the case of a Michaelis-Menten reaction chain. In this case, the analysis of the spectrum of the operator specified by the matrix $A$ is relatively simple.
Another, a more complex option is several parallel reactions or parallel chains of reactions like
Michaelis-Menten.
For systems with a large number of reactants, the case of random pairing (of a random $E$) is also interesting. Such situations were considered in works on large ecological communities (see for example, Allesina and Tang, 2012).
Comment 9 line 202 - please provide arguments for considering the domain of the function is also convex.
We suppose that the domain is convex because we would like to deal with a convex optimization problem, where global minimum is unique. In such problems, the objective function is a convex function and the feasible set is a convex set. The most natural domain in our biological context is the simplex $X_k \ge 0$, $\sum X_k < C_x$, where $C_x>0$ is a constant.
Comment 10
I suggest an additional analysis to assess the impact of η on Psur
The question of the dependence of the survival probability $P_{sur}$ on the parameter $\eta$ is complex. The parameter determines the type of stress (cooling or, conversely, heat shock, exposure to toxins, radiation, etc.) and stress level (for example, concentration of toxins). The probability of survival depends on $\eta$ through the parameters $a_0, p_0$ (see relation (55)). It is difficult to determine this dependence within the framework of the simple model, considered in this section. In the calculations, the parameters were chosen so that the survival probability $P_{sur}$ takes on more or less realistic values between 0 and 1. To investigate this dependence, we need more advanced spatially extended models taking into account immune reactions and other phenomena.
Comment 11
Use “Appendix A”. Consider using the piece of code in Latex
Thank you very much for this proposition and the code!! I pasted this, and the text has become better.
ANSWER TO REFEREES Comments
I am grateful to Referees for useful comments and remarks. The major correction is
a subsection “Innovations” added according to Major Comment 1 in Report 3.
Moreover, I looked through manuscript again and corrected some misprints and also made minor
improvements in presentation.
ANSWER TO Report 1
Indeed, it would be interesting to extend this approach on reaction-diffusion systems. I have some results in this direction, but they do not seem very impressive to me, and to simplify the presentation, I decided to limit myself to simpler models.
ANSWER TO Report 2
Thank you for this estimate of my work!
ANSWER TO Report 3.
The manuscript is modified along Referee’s propositions.
Major comments
Comment 1: The author should include a new paragraph listing the innovative aspects of the paper and the strengthen the main differences relative to other references, specifically Vakulenko and Grigoriev (2021).
THANK YOU FOR THIS QUESTION!! Of course I thought about this question but apparently I didn’t write it in enough detail.
The new paragraph:
This article continues the series of works (see, for example, Vakulenko and Grigoriev,2006, Vakulenko and Grigoriev, 2021), where the idea of replicative stability is
developed proposed by M. Gromov and A. Carbone (Gromov and Carbone). It was
shown that some properties of evolution could be explained on the basis that biosystems
must maintain homeostasis under the influence of stress and fluctuations (both internal
and external), and this homeostasis support must be done by subsequent replications. In129
deed, many features of evolution can be explained in this way, for example, the tendency
to increase the number of genes (see a review in (Vakulenko, 2014)). The key question,
however, is to explain complexity of genetic networks. An attempt to resolve this prob132
lem was made in (Vakulenko and Grigoriev, 2021), where a model of the response of
the biochemical system to stress is introduced. In this work, the entropy of a stressful
environment is defined and it is shown that an effective response to stress is possible if
the binary logarithm of the size of the regulatory genetic network is not less than this entropy. Therefore, according to (Vakulenko and Grigoriev, 2021) the main reason, why
regulatory networks grow, is complexity and diversity of stress environment.
In this paper, the main innovation with respect to these works, is that, in order
to describe a response to stresses, compartment model is used. It allows us to take into account the organism modular structure and stem cell activity. The organism
response to stress goes via populations of cells in the compartments, and dynamics of
these populations is determined by the standard Lotka-Volterra model. Such approach
permits to advance the one of key biological problems, the Peto paradox, because it will
be shown that then the organism size directly affects stress response mechanisms. The
second innovation with respect to (Vakulenko and Grigoriev,2021) is that we no longer
need the complexity of the environment to explain the complexity of an organism’s
genetic network. If the organismenvironment fluctuates, then this is enough to initiate an
increase in the complexity of the genetic network. At last, the third intriguing innovative
element is that when an organismis divided into compartments, there is no longer a need
to fine-tune genetic regulatory networks (as in connectionist models (Mjolsness et al.)).
Instead, it is enough to adjust an appropriate interaction between the compartments.
Minor comments
Comment 1. All minor changes have made according to Referee remarks.
The most of these errors are trivial misprints, for example, instead of $N_{cell}$ was $M_{cell}$ etc.
At line 310 in the sentence “Of course the model from are toy …” . This sentence does not contain a reference to the works cited above. This is modified so:
“The models from [Vakulenko, Grigoriev 2022] and [Sudakov et al. ] look as toy ones,
nonetheless one can suppose that this conclusion on the number of coding genes
is valid for more realistic models”.
after Eq. (52) - explain the meaning of Mcell ;
after Eq. (54) - explain the meaninng of Prc,mod ;
Here should be N_{cell} and P_{c, mod}.
Comment 2 I suggest editing the inline references. Examples: Koonin (2011, p. 250), Ventsel and Freidlin (1970).
I changed the style of references, unfortunately, I am not so familiar with these things.
Comment 3 I suggest replacing the transpose operator “tr” by the one that commonly used in Mathematics - “⊺” - all over the manuscript.
Fixed.
Comment 4: page 4 - consider “... for all t ∈ (−∞, +∞) ...”. I see no reason for using the physically consistent interval t ∈ [0, +∞). In case the author wants to emphasize the generalization of the representation I suggest to include a note to explicitly clarify the assumption.
Comment 5: line 136 - comment the reason for having t > 0 and not t ≥ 0. I agree that vi (0) is not exactly a solution; it is an initial condition, but on the other hand it must also conform to the conditions of the problem. Please check.
I changed the interval: t ∈ (−∞, +∞) is replaced on t ∈ [0, +∞).
Comment 6 please provide more details on the function χ(v) and how it is modeled every-
where. It seems to be an heavy-side function holding in distinct domains.
The old text:
where $\chi(v)=0$ outside of an open neighborhood W_{delta} of an equilibrium, which is a subset a
homeostasis domain
This text is modified so:
where $\chi(v)=0$ is defined as follows. The $\chi$ is a Heaviside-like function,
which is equal to 0 outside of an open neighborhood W_{\delta} of an equilibrium and
$\chi(v)$ is equal to 1 inside this neighborhood. Here we assume that the neighborhood radius $\delta$ is so small that this neighborhood $W_{\delta}$ lies within the homeostasis domain.
Comment 7. please, provide some reasoning for ∆τ >> ∆t.
In this small paragraph I have changed the bad notation and slightly changed the presentation.
Now it looks so:
To describe the trend h(t), we use the model of subsequent environmental shocks.
We assume that h(t) are piecewise constant functions. Let t_1, t_2, ..., t_k, ... be an infinite
increasing sequence of time moments t_j. Let Δτ = min (t_{j+1} - t_j). We suppose
Δτ >> Δtr and Δτ >> Δtd , where Δtr Δtd are characteristic times of gene regulation
functioning and biochemical model dynamics, respectively. At the moments tj we have
1environment shocks, when an interaction between the environment and the biosystem
changes. The assumptions on Δτ, Δtr Δtd are natural. It means that changes in the
1organism environment are not very frequent and, therefore, organisms have time to react
to them. Indeed, otherwise it is difficult to expect that evolution could be successful.
Comment 8 I suggest including a comment on the dependence (or independence) of
ith reactant on the others. That is, comment the models’ structure regarding the coupling / decoupling
Within the framework of this weakly nonlinear approximation, all information about the interaction (coupling-decoupling) of reactants is contained in the matrix $A$. We can define
the coupling graph $(V, E)$, where the set $V$ of vertices is the set of reactants, and an edge $e={i,j}$ lies in the edge set $ E$ if $i$-th reactant interacts with $j$-th one. One can consider various cases of interaction of reactants that are relevant from a biological point of view. For example, we can consider the case of a Michaelis-Menten reaction chain. In this case, the analysis of the spectrum of the operator specified by the matrix $A$ is relatively simple.
Another, a more complex option is several parallel reactions or parallel chains of reactions like
Michaelis-Menten.
For systems with a large number of reactants, the case of random pairing (of a random $E$) is also interesting. Such situations were considered in works on large ecological communities (see for example, Allesina and Tang, 2012).
Comment 9 line 202 - please provide arguments for considering the domain of the function is also convex.
We suppose that the domain is convex because we would like to deal with a convex optimization problem, where global minimum is unique. In such problems, the objective function is a convex function and the feasible set is a convex set. The most natural domain in our biological context is the simplex $X_k \ge 0$, $\sum X_k < C_x$, where $C_x>0$ is a constant.
Comment 10
I suggest an additional analysis to assess the impact of η on Psur
The question of the dependence of the survival probability $P_{sur}$ on the parameter $\eta$ is complex. The parameter determines the type of stress (cooling or, conversely, heat shock, exposure to toxins, radiation, etc.) and stress level (for example, concentration of toxins). The probability of survival depends on $\eta$ through the parameters $a_0, p_0$ (see relation (55)). It is difficult to determine this dependence within the framework of the simple model, considered in this section. In the calculations, the parameters were chosen so that the survival probability $P_{sur}$ takes on more or less realistic values between 0 and 1. To investigate this dependence, we need more advanced spatially extended models taking into account immune reactions and other phenomena.
Comment 11
Use “Appendix A”. Consider using the piece of code in Latex
Thank you very much for this proposition and the code!! I pasted this, and the text has become better.
ANSWER TO REFEREES Comments
I am grateful to Referees for useful comments and remarks. The major correction is
a subsection “Innovations” added according to Major Comment 1 in Report 3.
Moreover, I looked through manuscript again and corrected some misprints and also made minor
improvements in presentation.
ANSWER TO Report 1
Indeed, it would be interesting to extend this approach on reaction-diffusion systems. I have some results in this direction, but they do not seem very impressive to me, and to simplify the presentation, I decided to limit myself to simpler models.
ANSWER TO Report 2
Thank you for this estimate of my work!
ANSWER TO Report 3.
The manuscript is modified along Referee’s propositions.
Major comments
Comment 1: The author should include a new paragraph listing the innovative aspects of the paper and the strengthen the main differences relative to other references, specifically Vakulenko and Grigoriev (2021).
THANK YOU FOR THIS QUESTION!! Of course I thought about this question but apparently I didn’t write it in enough detail.
The new paragraph:
This article continues the series of works (see, for example, Vakulenko and Grigoriev,2006, Vakulenko and Grigoriev, 2021), where the idea of replicative stability is
developed proposed by M. Gromov and A. Carbone (Gromov and Carbone). It was
shown that some properties of evolution could be explained on the basis that biosystems
must maintain homeostasis under the influence of stress and fluctuations (both internal
and external), and this homeostasis support must be done by subsequent replications. In129
deed, many features of evolution can be explained in this way, for example, the tendency
to increase the number of genes (see a review in (Vakulenko, 2014)). The key question,
however, is to explain complexity of genetic networks. An attempt to resolve this prob132
lem was made in (Vakulenko and Grigoriev, 2021), where a model of the response of
the biochemical system to stress is introduced. In this work, the entropy of a stressful
environment is defined and it is shown that an effective response to stress is possible if
the binary logarithm of the size of the regulatory genetic network is not less than this entropy. Therefore, according to (Vakulenko and Grigoriev, 2021) the main reason, why
regulatory networks grow, is complexity and diversity of stress environment.
In this paper, the main innovation with respect to these works, is that, in order
to describe a response to stresses, compartment model is used. It allows us to take into account the organism modular structure and stem cell activity. The organism
response to stress goes via populations of cells in the compartments, and dynamics of
these populations is determined by the standard Lotka-Volterra model. Such approach
permits to advance the one of key biological problems, the Peto paradox, because it will
be shown that then the organism size directly affects stress response mechanisms. The
second innovation with respect to (Vakulenko and Grigoriev,2021) is that we no longer
need the complexity of the environment to explain the complexity of an organism’s
genetic network. If the organismenvironment fluctuates, then this is enough to initiate an
increase in the complexity of the genetic network. At last, the third intriguing innovative
element is that when an organismis divided into compartments, there is no longer a need
to fine-tune genetic regulatory networks (as in connectionist models (Mjolsness et al.)).
Instead, it is enough to adjust an appropriate interaction between the compartments.
Minor comments
Comment 1. All minor changes have made according to Referee remarks.
The most of these errors are trivial misprints, for example, instead of $N_{cell}$ was $M_{cell}$ etc.
At line 310 in the sentence “Of course the model from are toy …” . This sentence does not contain a reference to the works cited above. This is modified so:
“The models from [Vakulenko, Grigoriev 2022] and [Sudakov et al. ] look as toy ones,
nonetheless one can suppose that this conclusion on the number of coding genes
is valid for more realistic models”.
after Eq. (52) - explain the meaning of Mcell ;
after Eq. (54) - explain the meaninng of Prc,mod ;
Here should be N_{cell} and P_{c, mod}.
Comment 2 I suggest editing the inline references. Examples: Koonin (2011, p. 250), Ventsel and Freidlin (1970).
I changed the style of references, unfortunately, I am not so familiar with these things.
Comment 3 I suggest replacing the transpose operator “tr” by the one that commonly used in Mathematics - “⊺” - all over the manuscript.
Fixed.
Comment 4: page 4 - consider “... for all t ∈ (−∞, +∞) ...”. I see no reason for using the physically consistent interval t ∈ [0, +∞). In case the author wants to emphasize the generalization of the representation I suggest to include a note to explicitly clarify the assumption.
Comment 5: line 136 - comment the reason for having t > 0 and not t ≥ 0. I agree that vi (0) is not exactly a solution; it is an initial condition, but on the other hand it must also conform to the conditions of the problem. Please check.
I changed the interval: t ∈ (−∞, +∞) is replaced on t ∈ [0, +∞).
Comment 6 please provide more details on the function χ(v) and how it is modeled every-
where. It seems to be an heavy-side function holding in distinct domains.
The old text:
where $\chi(v)=0$ outside of an open neighborhood W_{delta} of an equilibrium, which is a subset a
homeostasis domain
This text is modified so:
where $\chi(v)=0$ is defined as follows. The $\chi$ is a Heaviside-like function,
which is equal to 0 outside of an open neighborhood W_{\delta} of an equilibrium and
$\chi(v)$ is equal to 1 inside this neighborhood. Here we assume that the neighborhood radius $\delta$ is so small that this neighborhood $W_{\delta}$ lies within the homeostasis domain.
Comment 7. please, provide some reasoning for ∆τ >> ∆t.
In this small paragraph I have changed the bad notation and slightly changed the presentation.
Now it looks so:
To describe the trend h(t), we use the model of subsequent environmental shocks.
We assume that h(t) are piecewise constant functions. Let t_1, t_2, ..., t_k, ... be an infinite
increasing sequence of time moments t_j. Let Δτ = min (t_{j+1} - t_j). We suppose
Δτ >> Δtr and Δτ >> Δtd , where Δtr Δtd are characteristic times of gene regulation
functioning and biochemical model dynamics, respectively. At the moments tj we have
1environment shocks, when an interaction between the environment and the biosystem
changes. The assumptions on Δτ, Δtr Δtd are natural. It means that changes in the
1organism environment are not very frequent and, therefore, organisms have time to react
to them. Indeed, otherwise it is difficult to expect that evolution could be successful.
Comment 8 I suggest including a comment on the dependence (or independence) of
ith reactant on the others. That is, comment the models’ structure regarding the coupling / decoupling
Within the framework of this weakly nonlinear approximation, all information about the interaction (coupling-decoupling) of reactants is contained in the matrix $A$. We can define
the coupling graph $(V, E)$, where the set $V$ of vertices is the set of reactants, and an edge $e={i,j}$ lies in the edge set $ E$ if $i$-th reactant interacts with $j$-th one. One can consider various cases of interaction of reactants that are relevant from a biological point of view. For example, we can consider the case of a Michaelis-Menten reaction chain. In this case, the analysis of the spectrum of the operator specified by the matrix $A$ is relatively simple.
Another, a more complex option is several parallel reactions or parallel chains of reactions like
Michaelis-Menten.
For systems with a large number of reactants, the case of random pairing (of a random $E$) is also interesting. Such situations were considered in works on large ecological communities (see for example, Allesina and Tang, 2012).
Comment 9 line 202 - please provide arguments for considering the domain of the function is also convex.
We suppose that the domain is convex because we would like to deal with a convex optimization problem, where global minimum is unique. In such problems, the objective function is a convex function and the feasible set is a convex set. The most natural domain in our biological context is the simplex $X_k \ge 0$, $\sum X_k < C_x$, where $C_x>0$ is a constant.
Comment 10
I suggest an additional analysis to assess the impact of η on Psur
The question of the dependence of the survival probability $P_{sur}$ on the parameter $\eta$ is complex. The parameter determines the type of stress (cooling or, conversely, heat shock, exposure to toxins, radiation, etc.) and stress level (for example, concentration of toxins). The probability of survival depends on $\eta$ through the parameters $a_0, p_0$ (see relation (55)). It is difficult to determine this dependence within the framework of the simple model, considered in this section. In the calculations, the parameters were chosen so that the survival probability $P_{sur}$ takes on more or less realistic values between 0 and 1. To investigate this dependence, we need more advanced spatially extended models taking into account immune reactions and other phenomena.
Comment 11
Use “Appendix A”. Consider using the piece of code in Latex
Thank you very much for this proposition and the code!! I pasted this, and the text has become better.