A Domain Generation Diagnosis Framework for Unseen Conditions Based on Adaptive Feature Fusion and Augmentation
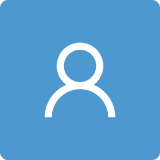
Round 1
Reviewer 1 Report
Comments and Suggestions for AuthorsThe work is intresting, some remarks are highlighted:
- Research finding with dataset(s) used and best result(s) is / are missing in the abstract.
- Theory of the relationship between domain adaptation and Transfer learning (specifying which sub-category of Transfer learning) is missing in the introduction,
- Some details and results are missing about the inner outer and balls fault detection using the proposed scheme such as preprocessing, data balancing,
confusion matrix, other metrics (F1-score, and False positive rate). The provided results are superficial.
Author Response
Comments 1: Research finding with dataset(s) used and best result(s) is / are missing in the abstract.
Response 1: Thank you for pointing this out. The authors are grateful for your comments, which is of great benefit to the article quality. Therefore, we have added the utilized datasets and our achieved best results in the abstract. The revised part of abstract can be fond in the Page 1, Line 20~22 marked in blue, which are shown as below.
…
“The feasibility of the proposed unseen conditions diagnostic framework is validated on the SDUST and PU datasets and achieved the highest diagnostic accuracy 94.15% and 93.27% separately.”
…
Comments 2: Theory of the relationship between domain adaptation and Transfer learning (specifying which sub-category of Transfer learning) is missing in the introduction.
Response 2: The authors are grateful for your comments concerning the introduction issues in our article, which is of great benefit to the article quality. Therefore, we have revised the Introduction part regarding the relationship between domain adaptation and Transfer learning. In the revision, the explanations of domain adaptation and transfer learning are added. In addition, other sentences and textual expressions have also been modified accordingly. The revised part of introduction can be found in the Page 2, Line 81~83 marked in blue, which are shown as below.
…
“Domain generation (DG) is a method of transfer learning which endeavors to generalize a trained model, leveraging a diverse array of source datasets, to accommodate unseen target domains.”
…
Comments 3: Some details and results are missing about the inner outer and balls fault detection using the proposed scheme such as preprocessing, data balancing, confusion matrix, other metrics (F1-score, and False positive rate). The provided results are superficial.
Response 3: The authors are grateful for your comments concerning the result issues in our article, which is of great benefit to the validation of the article. For the preprocessing issues, the normalized method is utilized for the original vibration signals, the number of every dataset in training data are controlled as 150 which is enough for model training. As for the results analysis, we have carried the confusion matrix evaluation for performance validation. Moreover, the Experiment part has been revised accordingly. The revised part of introduction can be found in the Page 7, Line 268~272 and Page 10, Line 349~354 marked in blue, which are shown as below.
…
“Within these datasets, we designate a specific working condition or machine as the unseen target domain, serving as the benchmark for evaluating the model's performance. The normalized method is utilized for data preprocess which making the data size within the same interval to ensure effective convergence of the model. The number of each dataset of training categories is controlled as 150 for data balancing.”
…
Furthermore, it demonstrates superior stability compared to other comparison methods in most tasks. Fig. 4 illustrates the classification accuracy of various diagnostic tasks for Case 1 and Case 2, providing a visual aid for comparing the diagnostic results. Fig.5 illustrates the confusion matrix of proposed methods in 4 diagnostic tasks of Case 1. A plausible explanation for this finding is that the data distribution without loads differs significantly from that with loads, thereby exacerbating the issue of distribution shift.
…
Author Response File: Author Response.pdf
Reviewer 2 Report
Comments and Suggestions for AuthorsThis manuscript proposed a domain generation diagnosis framework for bearing intelligent fault diagnosis, which is a hot topic in the field of machinery fault diagnosis. Experiments on two different datasets to validate the effectiveness of the proposed method. However, some problems should be addressed. The detailed comments are summarized as follows:
1. Question about the proposed method. Does the number of source domains have an impact on the experimental results?
2. Why is it necessary to expand the data samples, and how to evaluate the quality of the generated data?
3. How to set the values of tradeoff hyperparameters in the learning objective. What is the impact of changing the value of the tradeoff parameter on the experimental performance of the proposed method?
4. Some details require attention. For example, Page 5, line 189, Page 6, line 242, Page 8, line 313.
Comments on the Quality of English LanguageThere are some grammatical errors in this manuscript; please ask the author to carefully review the entire text.
Author Response
Comments 1: Question about the proposed method. Does the number of source domains have an impact on the experimental results?
Response 1: Thank you for pointing this out, the authors are very grateful for your comments. The impact of the number of source domains is quite an interesting question. On the one hand, according to the transfer learning and domain generalization theory [1], more source domains enhance the generalization ability of the diagnostic model for the unseen domain indicts the operation conditions and the working environment are unknown, hence, more source domain is beneficial to this kind of stations. On the other hand, in our proposed model, the data and the label need to be augmented for domain generalization model training, which mainly based on the heterogeneity of data information between source domains. In other words, the effectiveness of data augmentation can be enhanced as the more source domains are obtained. In this paper, for the domain generalization diagnostic model, the training requires at least 2 sources domains attribute to the loss structure, as the data augmentation is the prerequisite step for model training, hence in the proposed model in our paper, 3 source domains are requested. In the experiment and validation parts, due to the utilized datasets can be divided into 4 operation domains, 3 source domains can be at most utilized and the rest one using for validation. More source domains can improve the generalization ability of the diagnostic domains, however, for the test accuracy of the target domain there is a limitation, which can be further validated using more suitable datasets. As the aspect of industrial application, the qualified source domain datasets are hard to access, hence many operation conditions lack of enough data, it is unrealistic to use numerous datasets to improve model performance.
[1] Zhao, C. and W.M. Shen, Adaptive open set domain generalization network: Learning to diagnose unknown faults under unknown working conditions. Reliability Engineering & System Safety, 2022. 226: p. 12.
Comments 2: Why is it necessary to expand the data samples, and how to evaluate the quality of the generated data?
Response 2: We are very grateful for your comments which can be beneficial to the quality of our paper. The augmentation of the data samples by data fusion and label fusion can expanded the discrete data domains to form continuous data spaces making the domain generalization model learn sufficient mappings between data and labels. The effectiveness of the data expend are validated by discussing the hyperparameter and of the data augmentation process as shown in Equ. (3) and (4), the results are shown in Fig. (8). In order to facilitate understanding and display of results, we have made corresponding modifications to the content of the article. The revised part is in Page 12, line 412-417 which are marked in blue.
…
The hyperparameter in the data augmentation module regulates the extent of interpolation between original feature vector pairs. The coefficient , derived from the Beta (, ) distribution, as shown in Equ.(4), remains constant for each minibatch, after which the mixup method is implemented on the shuffled minibatch. Consequently, we explored the influence of gradually varying α from 0.1 to 1 on cross-domain diagnostic performance. For comparison, we also considered fixed λ values ranging from 0.1 to 1.
…
Comments 3: How to set the values of tradeoff hyperparameters in the learning objective. What is the impact of changing the value of the tradeoff parameter on the experimental performance of the proposed method?
Response 3: We are grateful for pointing out the determination of hyperparameters selection in the machine learning applications. The selected hyperparameters is essentially important the network training. We have made further explanations in the revision, such as the detailed reasons of learning rate dropping method and the advantages of utilized optimizer Adam. Moreover, the selection of Batch size and the structural hyperparameters of network are further illustrated based on the loss curve in the training process. The revised part in the paper is marked Blue in the revision. The 3 main reasons of the hyperparameter selection are listed below.
(1) The initial learning rate is set as 0.001, and Learning Rate Schedule is set as decrease by a factor of 0.1 every 20 epochs, after 100 epochs of training, the learning rate has dropped to 1e-8. Such optimization accuracy is fine enough for tensor optimization of type double. The phasing down of the learning rate allows the optimization process of the neural network to converge to a finer neighborhood of the optimal solution. Therefore, the initial learning rate does not need to be set very small, so that the search range for the optimal solution of the neural network parameter optimization can be increased in the initial phase of training.
(2) The Adam optimizer is used in the neural network optimization process. The Adam optimizer combines the advantages of Adagrad's goodness in dealing with sparse gradients and RMSprop's goodness in dealing with non-smooth objectives, and is able to automatically adjust the learning rate, converge faster, and perform better in complex networks.
(3) Batch size and Training epochs: The choice of Batch size is based on the size of each input data volume as a percentage of the overall data volume and the amount of memory capacity of the neural network training hardware, we use a GPU model 24GB-RTX4090, according to the training loss curve and the test curve to select the appropriate Batch size, the training curve are shown below. As shown in the figure, the loss of training and testing decays well, the training and test accuracy increases in first 30 epochs and eventually remains above 90%. The loss and the accuracy converge to acceptable accuracy and no overfitting. Furthermore, the loss and the accuracy hasn't changed excessively after 100 epochs, so select 100 as the training epoch is considerable in terms of training time.
In order to facilitate understanding and display of results, we have made corresponding modifications to the content of the article. The revised part is in Page 7, line 256-269 which are marked in blue.
…
The label loss in source domain is calculated as and the domain fusion loss is calculated as in every epoch. The optimizer Adam is utilized to optimize the parameters of feature extractor and the parameters of domain classifiers, the learning rate is set as 0.001. The explicit steps of adversarial transfer learning are shown in Table 1. The Learning Rate Schedule is set as decrease by a factor of 0.1 every 20 epochs. The Adam optimizer is used in the neural network optimization process. The Adam optimizer combines the advantages of Adagrad's goodness in dealing with sparse gradients and RMSprop's goodness in dealing with non-smooth objectives, and is able to automatically adjust the learning rate, converge faster, and perform better in complex networks. Considering the amount of data in each training batch as a proportion of the overall amount of data, and the savings in training time, the batch size is set as 128 and the training epochs is set as 100.
…
Comments 4: Some details require attention. For example, Page 5, line 189, Page 6, line 242, Page 8, line 313.
Response 4: Thank you for your comments, the problem mentioned above is truly noticeable. According to the reviewer’s comments, we have made relevant revisions and we have checked the rest part of the article make sure the article’s writing details.
Author Response File: Author Response.pdf
Round 2
Reviewer 1 Report
Comments and Suggestions for AuthorsGreat !! the paper has been considerably improved.