Machine Learning and Soft Computing: Current Trends and Applications
A special issue of Applied Sciences (ISSN 2076-3417). This special issue belongs to the section "Computing and Artificial Intelligence".
Deadline for manuscript submissions: 31 August 2024 | Viewed by 1383
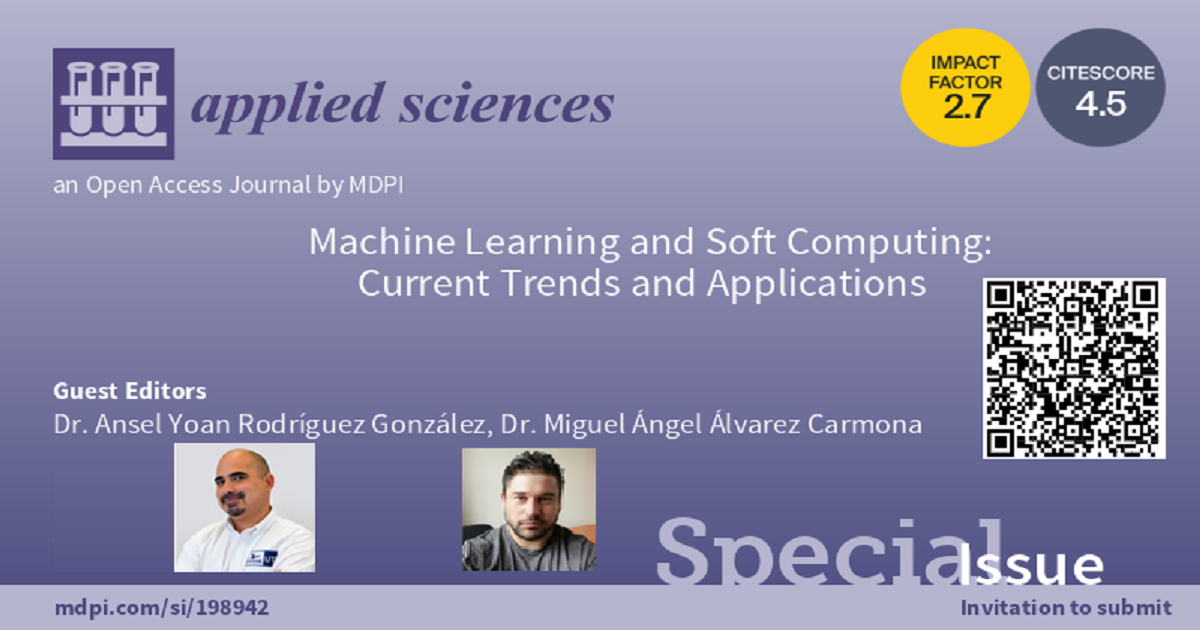
Special Issue Editors
Interests: data mining; pattern recognition; machine learning; evolutionary computation
Special Issues, Collections and Topics in MDPI journals
Special Issue Information
Dear Colleagues,
In contemporary times, the adoption of machine learning and soft computing techniques has established itself as a customary practice across research domains and industries such as healthcare, finance, manufacturing, energy, education, business management, and beyond. These methodologies have significantly bolstered decision-making capabilities and facilitated the automation of numerous processes. In order to stay abreast of the prevailing approaches within these realms, this Special Issue is dedicated to examining contributions that elucidate the latest trends and contemporary methodologies, within both industry and academia. Contributions encompass, though are not confined to, the following:
- Advanced Machine Learning Algorithms
- Deep learning architectures.
- Reinforcement learning.
- Ensemble methods.
- Transfer learning.
- Soft Computing Paradigms
- Fuzzy systems.
- Genetic algorithms.
- Neural networks.
- Swarm intelligence.
- Natural Language Processing
- Sentiment analysis.
- Topic modeling.
- Named entity recognition.
- Interdisciplinary Applications
- Healthcare informatics.
- Financial prediction and analysis.
- Image and speech recognition.
- Explainability and Interpretability
- Interpretable machine learning models.
- Explainable AI approaches.
- Ethical Considerations in Machine Learning
- Bias and fairness.
- Transparency and accountability.
We welcome both original research articles and comprehensive reviews that contribute to the understanding and development of machine learning and soft computing. Researchers are encouraged to present innovative solutions, share practical experiences, and highlight challenges and opportunities in this rapidly evolving field.
Yours sincerely,
Dr. Ansel Yoan Rodríguez González
Dr. Miguel Ángel Álvarez Carmona
Guest Editors
Manuscript Submission Information
Manuscripts should be submitted online at www.mdpi.com by registering and logging in to this website. Once you are registered, click here to go to the submission form. Manuscripts can be submitted until the deadline. All submissions that pass pre-check are peer-reviewed. Accepted papers will be published continuously in the journal (as soon as accepted) and will be listed together on the special issue website. Research articles, review articles as well as short communications are invited. For planned papers, a title and short abstract (about 100 words) can be sent to the Editorial Office for announcement on this website.
Submitted manuscripts should not have been published previously, nor be under consideration for publication elsewhere (except conference proceedings papers). All manuscripts are thoroughly refereed through a single-blind peer-review process. A guide for authors and other relevant information for submission of manuscripts is available on the Instructions for Authors page. Applied Sciences is an international peer-reviewed open access semimonthly journal published by MDPI.
Please visit the Instructions for Authors page before submitting a manuscript. The Article Processing Charge (APC) for publication in this open access journal is 2400 CHF (Swiss Francs). Submitted papers should be well formatted and use good English. Authors may use MDPI's English editing service prior to publication or during author revisions.
Keywords
- advanced machine learning algorithms
- deep learning architectures
- reinforcement learning
- ensemble methods
- transfer learning soft computing paradigms
- fuzzy systems
- genetic algorithms
- neural networks
- swarm intelligence natural language processing
- sentiment analysis
- topic modeling
- named entity recognition interdisciplinary applications
- healthcare informatics
- financial prediction and analysis
- image and speech recognition explainability and interpretability
- interpretable machine learning models
- explainable AI approaches ethical considerations in machine learning
- bias and fairness
- transparency and accountability
Benefits of Publishing in a Special Issue
- Ease of navigation: Grouping papers by topic helps scholars navigate broad scope journals more efficiently.
- Greater discoverability: Special Issues support the reach and impact of scientific research. Articles in Special Issues are more discoverable and cited more frequently.
- Expansion of research network: Special Issues facilitate connections among authors, fostering scientific collaborations.
- External promotion: Articles in Special Issues are often promoted through the journal's social media, increasing their visibility.
- e-Book format: Special Issues with more than 10 articles can be published as dedicated e-books, ensuring wide and rapid dissemination.
Further information on MDPI's Special Issue polices can be found here.