AI-Enabled Cyber Defence in IoT Deployments: Challenges and Opportunities
A special issue of Applied Sciences (ISSN 2076-3417). This special issue belongs to the section "Electrical, Electronics and Communications Engineering".
Deadline for manuscript submissions: closed (30 December 2023) | Viewed by 8969
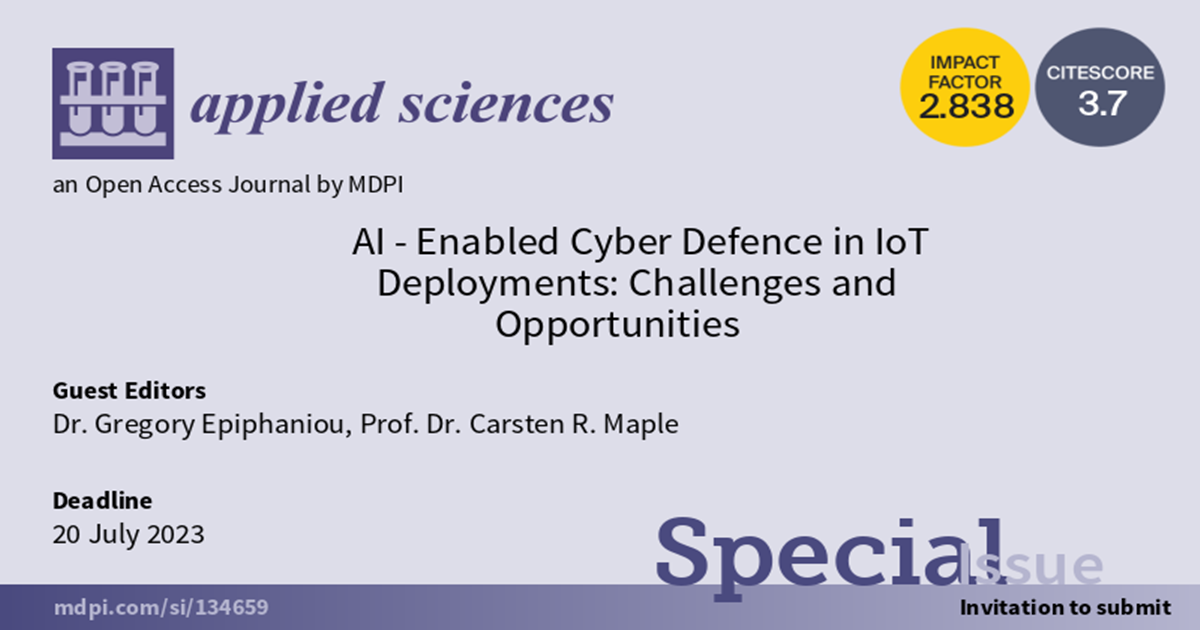
Special Issue Editors
Interests: cyber resilience; proactive network defence; physical layer security
Special Issues, Collections and Topics in MDPI journals
Interests: cyber-physical systems; cyber resilience
Special Issues, Collections and Topics in MDPI journals
Special Issue Information
Dear Colleagues,
As a result of the massive growth in cyber threats, the operational environment is rapidly shifting, resulting in major gaps in cyber decision-making skills with regard to defence. The existing legal and regulatory compliance procedures were not intended to work in cyberspace, increasing the need for cyber-resilient, mission-critical systems. Cyber–physical systems and the Internet of Things have developed as a new battlefield in warfare, with assaults against them no longer restricted to cyberspace. This tendency is further supported by our limited ability to precisely evaluate the immediate security risk of these mission-critical systems, given the fast change and variety of threat landscapes and related vulnerabilities in contemporary cyberspaces. In addition, the majority of our legacy cyber–physical systems are only protected in isolation from the designs of the information systems with which they must eventually interact with. The transition and sustainability of these structures demand a broader system design approach as a reference for the interaction of these systems with networks and for comprehensive security from component-level to systems-level integration of cyberspace-enabled applications.
This Special Issue focuses on emerging topics in employing intelligent operations, algorithms, and processes that support a broad range of applications, with an emphasis on the following research directions: (a) IoT cyber resilience; (b) risk-and-attack surface-exposure analyses; (c) security management processes and legal and regulatory implications in modern cyberinfrastructures; (d) AI-enabled cyber threats and proactive defences.
This Special Issue is devoted to presenting cutting-edge research addressing the many research trends and concerns in utilising AI to defend modern cyberinfrastructures, as well as providing academics and practitioners with valuable insights into the efficiencies of these innovations in modern cyber defence.
Topics of interest for this Special Issue include, but are not limited to, the following:
- Cyber resilience and system security assessments using AI;
- Cyber security risk in transdisciplinary settings;
- DL-based cyberthreat and effects modelling;
- Cyber defence taxonomy and DL-enabled risk-estimation models;
- AI-enhanced cyber threats and defences;
- Advancements in RegTech services for IoT;
- Cyber modeling and simulation for cyber incident analysis;
- Privacy and data control using intelligent operations;
- Secure quantum communications;
- Adversarial machine learning;
- IoT/CPS cybersecurity;
- Distributed technologies for defensive cyber operations;
- Intelligent threat network degradation
- Cyber law and ethics;
- Cyberthreat information presentation
- Cyber threat intelligence- and information-acquisition systems;
- Compliance-aware security engineering.
Dr. Gregory Epiphaniou
Prof. Dr. Carsten R. Maple
Guest Editors
Manuscript Submission Information
Manuscripts should be submitted online at www.mdpi.com by registering and logging in to this website. Once you are registered, click here to go to the submission form. Manuscripts can be submitted until the deadline. All submissions that pass pre-check are peer-reviewed. Accepted papers will be published continuously in the journal (as soon as accepted) and will be listed together on the special issue website. Research articles, review articles as well as short communications are invited. For planned papers, a title and short abstract (about 100 words) can be sent to the Editorial Office for announcement on this website.
Submitted manuscripts should not have been published previously, nor be under consideration for publication elsewhere (except conference proceedings papers). All manuscripts are thoroughly refereed through a single-blind peer-review process. A guide for authors and other relevant information for submission of manuscripts is available on the Instructions for Authors page. Applied Sciences is an international peer-reviewed open access semimonthly journal published by MDPI.
Please visit the Instructions for Authors page before submitting a manuscript. The Article Processing Charge (APC) for publication in this open access journal is 2400 CHF (Swiss Francs). Submitted papers should be well formatted and use good English. Authors may use MDPI's English editing service prior to publication or during author revisions.
Keywords
- CPS
- IoT
- AI
- threat and risk modelling
- DLT
- cyber defence