Cyber-Physical Systems for Intelligent Transportation Systems
A special issue of Applied Sciences (ISSN 2076-3417). This special issue belongs to the section "Transportation and Future Mobility".
Deadline for manuscript submissions: closed (20 August 2023) | Viewed by 4531
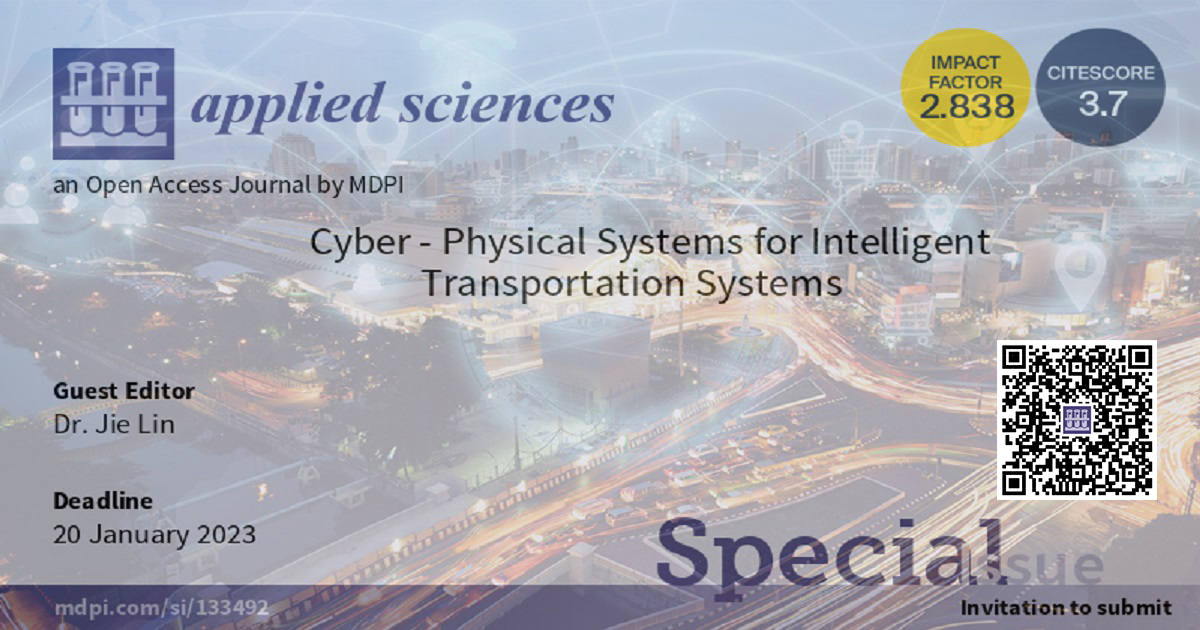
Special Issue Editor
Special Issue Information
Dear Colleagues,
Cyber-Physical Systems for Intelligent Transportation Systems is referred to as the system that can efficiently integrate both cyber and physical components through the integration of the modern computing and communication
Technologies for smart transportation. This poses new challenges in the advancement of computer and communication technologies as well as regarding software solutions. Therefore, this Special Issue is intended for the presentation of new ideas and experimental results in the field of perception, computation and communication of CPS for ITS from design, service, and theory to its practical use.
This Special Issue will publish high-quality, original research papers, in the overlapping fields of:
- Cyber-Physical Systems for Intelligent Transportation Systems;
- Parallel and distributed computing;
- Artificial intelligence, machine learning and deep learning;
- Computational and data science;
- Big data applications, algorithms, and systems;
- Vehicular networks;
- Cloud/edge/fog computing;
- Other algorithms, theory and applications related with CPS for ITS;
Dr. Jie Lin
Guest Editor
Manuscript Submission Information
Manuscripts should be submitted online at www.mdpi.com by registering and logging in to this website. Once you are registered, click here to go to the submission form. Manuscripts can be submitted until the deadline. All submissions that pass pre-check are peer-reviewed. Accepted papers will be published continuously in the journal (as soon as accepted) and will be listed together on the special issue website. Research articles, review articles as well as short communications are invited. For planned papers, a title and short abstract (about 100 words) can be sent to the Editorial Office for announcement on this website.
Submitted manuscripts should not have been published previously, nor be under consideration for publication elsewhere (except conference proceedings papers). All manuscripts are thoroughly refereed through a single-blind peer-review process. A guide for authors and other relevant information for submission of manuscripts is available on the Instructions for Authors page. Applied Sciences is an international peer-reviewed open access semimonthly journal published by MDPI.
Please visit the Instructions for Authors page before submitting a manuscript. The Article Processing Charge (APC) for publication in this open access journal is 2400 CHF (Swiss Francs). Submitted papers should be well formatted and use good English. Authors may use MDPI's English editing service prior to publication or during author revisions.
Keywords
- cyber-physical systems for intelligent transportation systems
- vehicular network
- artificial intelligence, machine learning and deep learning
- edge/cloud computing
- application specific, reconfigurable, IoT, mobile and embedded architectures
- architecture modelling and performance evaluation
- machine learning systems, including algorithms/system co-design