Towards Machine Learning and Artificial Intelligence in the Farm-to-Fork Industry
A special issue of Applied Sciences (ISSN 2076-3417). This special issue belongs to the section "Agricultural Science and Technology".
Deadline for manuscript submissions: closed (30 June 2022) | Viewed by 3577
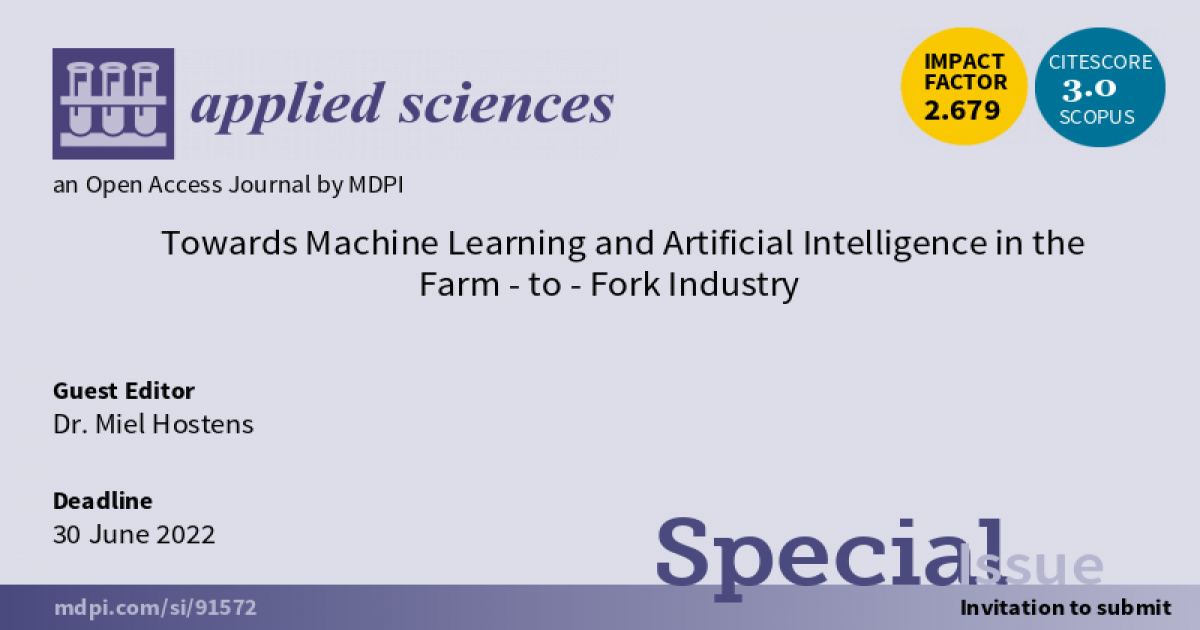
Special Issue Editor
Interests: dairy; regression modeling; dairy science; animal production; ruminant nutrition; animal nutrition; animal physiology; feed formulation; big data; data science
Special Issue Information
Dear Colleagues,
We are delighted to help organizing a Special Issue on “The Road towards Machine Learning and Artificial Intelligence for the Farm-to-Fork Industry” in Applied Sciences (MDPI).
Due to rapid development of precision livestock farming (PLF) and the availability of high-throughput information from sensors throughout the entire agricultural food chain, massive data has become available. Sensors produce data which represent the animal’s behavior as well as their environment (e.g. gas emissions, water quality) up to the transportation and processing of food. These PLF technologies have been proposed to help transition towards a more sustainable agriculture. The challenge of PLF technology nowadays is how to combine the enormous amount of (especially) heterogeneous data and subsequently model this data using novel techniques such as Machine Learning and Artificial Intelligence techniques.
The topics of interest for this Special Issue include, but are not limited to, the following:
- Advanced data-driven automated phenotyping using ML/AI
- Ontology design for Agrifood industry
- Data fusion techniques for Agrifood
- Machine learning using heterogenous PLF technology
- Improving traceability in the Agrifood using novel data driven techniques
Dr. Miel Hostens
Guest Editor
Manuscript Submission Information
Manuscripts should be submitted online at www.mdpi.com by registering and logging in to this website. Once you are registered, click here to go to the submission form. Manuscripts can be submitted until the deadline. All submissions that pass pre-check are peer-reviewed. Accepted papers will be published continuously in the journal (as soon as accepted) and will be listed together on the special issue website. Research articles, review articles as well as short communications are invited. For planned papers, a title and short abstract (about 100 words) can be sent to the Editorial Office for announcement on this website.
Submitted manuscripts should not have been published previously, nor be under consideration for publication elsewhere (except conference proceedings papers). All manuscripts are thoroughly refereed through a single-blind peer-review process. A guide for authors and other relevant information for submission of manuscripts is available on the Instructions for Authors page. Applied Sciences is an international peer-reviewed open access semimonthly journal published by MDPI.
Please visit the Instructions for Authors page before submitting a manuscript. The Article Processing Charge (APC) for publication in this open access journal is 2400 CHF (Swiss Francs). Submitted papers should be well formatted and use good English. Authors may use MDPI's English editing service prior to publication or during author revisions.
Keywords
- Big Data modeling
- machine learning
- Artificial Intelligence
- precision livestock farming