Human Health Monitoring Using Emerging Technologies: Towards Proper Usage of Genomics and Epigenetics in Molecular and Bio-Signaling Data
A special issue of Applied Sciences (ISSN 2076-3417). This special issue belongs to the section "Applied Biosciences and Bioengineering".
Deadline for manuscript submissions: closed (31 October 2022) | Viewed by 5952
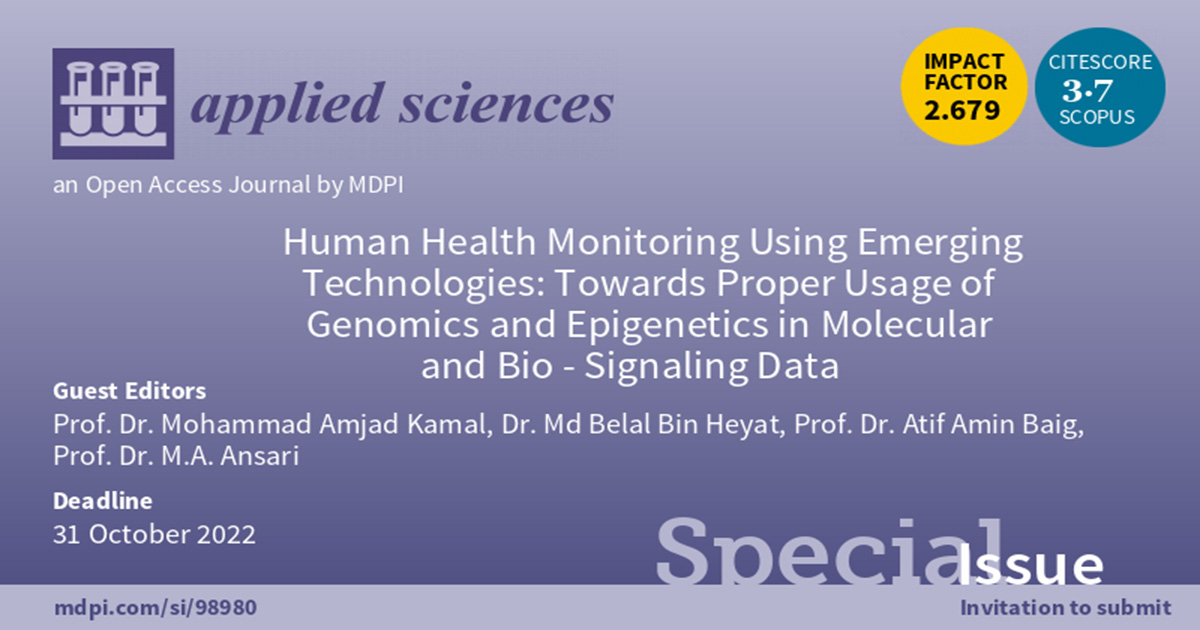
Special Issue Editors
2. King Fahd Medical Research Center, King Abdulaziz University, Jeddah 21589, Saudi Arabia
3. Department of Pharmacy, Faculty of Allied Health Sciences, Daffodil International University, Dhaka 1207, Bangladesh
4. Enzymoics, 7 Peterlee Place, Hebersham, NSW 2770, Australia
5. Novel Global Community Educational Foundation, Hebersham, Australia
Interests: biochemistry; neuroscience; enzymology; toxicology; metabolomics; nanomedicines; manual lymph drainage and miRNA; leadership in managing staff performance and chaplaincy
Special Issues, Collections and Topics in MDPI journals
2. Novel Global Community Educational Foundation, Hebersham, NSW 2770, Australia
Interests: medical machine learning; biosignal processing; cardiac disease; neurological disorders
Interests: human physiology; treatment; diabetic; yoga
Special Issue Information
Dear Colleagues,
Health monitoring of a complex human body organization is necessary to deal with vital physiological and pathological changes. In the current era of the Industrial Revolution 4.0, where digitalization has been overtaking all fields of science and medicine globally, there is a growing challenge when it comes to monitoring human health under interconnected fabrics of anatomy, physiology, biochemistry, genomics, epigenetics, and artificial intelligence due to the many social implications of health monitoring devices. Current inventions and innovations focusing on wearable wireless sensors have been helping toward curation, collection, and association at a big data scale. However, our lack of understanding and data for precision medicine at the genomic and epigenetic level means that we need to integrate big data for predictions, estimates, and treatment of underlying causes of different disorders and diseases in humans. Complementing the current emerging techniques with machine learning, deep learning, internet sources, blockchain, and quantum technology aligned with genomics and epigenetics at the molecular level could help toward a specific, better understanding of biomolecular signaling involved in monitoring health metabolomics. The subtopics to be covered in this Special Issue include but are not limited to:
- Health monitoring systems;
- Wearable devices;
- Molecular signaling;
- Biosignal and image processing;
- Psychoneurological human behavior such as stress, depression, anxiety, and fatigue;
- Arrhythmia, stroke, blood pressure, congenital heart disease, heart muscle disease, and pericardial disease;
- mental disorder, sleep disorder, motor imagery, Parkinson, Alzheimer, and schizophrenia;
- Diabetes, addiction, COVID-19, and encephalitis;
- Detection, localization, prediction, and treatment;
- ECG, EKG, EEG, and EOG;
- MRI and fMRI;
- Machine learning, deep learning, blockchain, computer vision;
- Quantum technology;
- Yoga
Prof. Dr. Mohammad Amjad Kamal
Dr. Md Belal Bin Heyat
Prof. Dr. Atif Amin Baig
Prof. Dr. M.A. Ansari
Guest Editors
Manuscript Submission Information
Manuscripts should be submitted online at www.mdpi.com by registering and logging in to this website. Once you are registered, click here to go to the submission form. Manuscripts can be submitted until the deadline. All submissions that pass pre-check are peer-reviewed. Accepted papers will be published continuously in the journal (as soon as accepted) and will be listed together on the special issue website. Research articles, review articles as well as short communications are invited. For planned papers, a title and short abstract (about 100 words) can be sent to the Editorial Office for announcement on this website.
Submitted manuscripts should not have been published previously, nor be under consideration for publication elsewhere (except conference proceedings papers). All manuscripts are thoroughly refereed through a single-blind peer-review process. A guide for authors and other relevant information for submission of manuscripts is available on the Instructions for Authors page. Applied Sciences is an international peer-reviewed open access semimonthly journal published by MDPI.
Please visit the Instructions for Authors page before submitting a manuscript. The Article Processing Charge (APC) for publication in this open access journal is 2400 CHF (Swiss Francs). Submitted papers should be well formatted and use good English. Authors may use MDPI's English editing service prior to publication or during author revisions.