State-of-the-Art of Intelligent Unmanned Systems
A special issue of Applied Sciences (ISSN 2076-3417). This special issue belongs to the section "Robotics and Automation".
Deadline for manuscript submissions: closed (31 December 2022) | Viewed by 2483
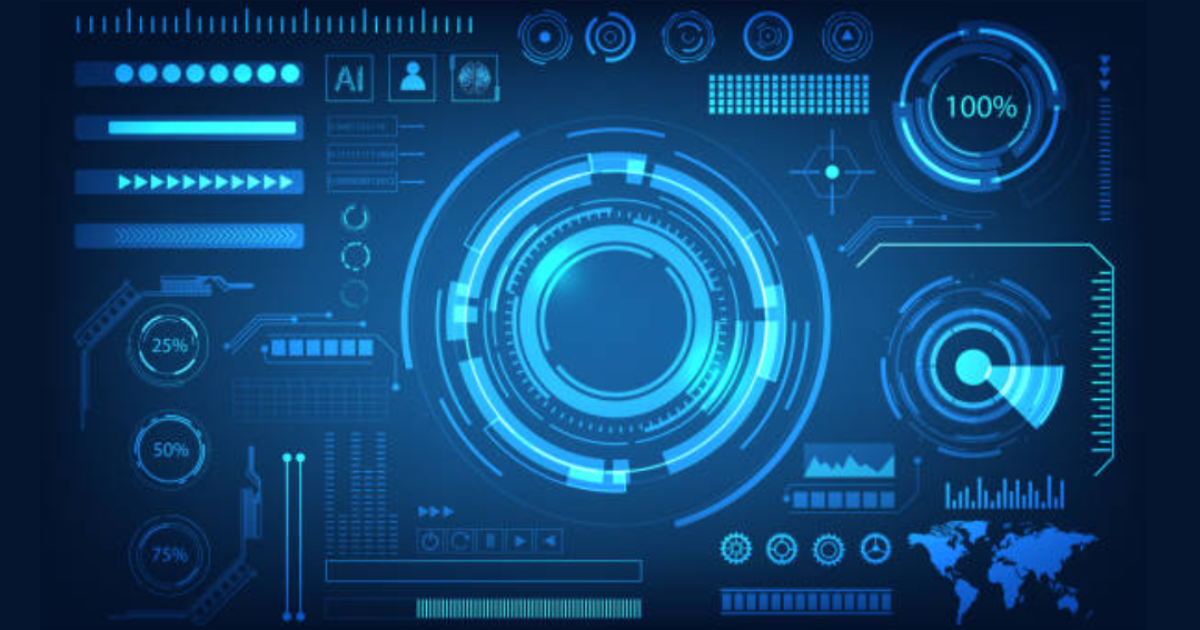
Special Issue Editors
Interests: perception and decision-making
Special Issues, Collections and Topics in MDPI journals
Interests: engineering information fusion theory and methods; collaborative optimization of unmanned systems; intelligent evaluation of man-machine hybrid systems; big data analysis of smart grids
Interests: intelligent driving vehicles; machine learning; deep learning; autonomous decision
Special Issues, Collections and Topics in MDPI journals
Special Issue Information
Dear Colleagues,
As the application of robots, unmanned aerial vehicles, unmanned vehicles, and other unmanned systems expands, the requirements for their level of intelligence progressively increase. At the same time, intelligent unmanned systems are at the forefront of a new generation of artificial intelligence. This Special Issue, a collaboration between Applied Science and the IEEE International Conference on Unmanned Systems (ICUS), will discuss recent findings and best practices in the fields of unmanned systems, robotics, automation, and intelligent systems. Topics of interest include algorithms, devices, and systems of intelligent unmanned systems; the intelligent control, motion planning, mechanical design, and communications engineering of unmanned systems; and intelligent unmanned system technology applications in robotics and automation, aerospace science and engineering, marine science and engineering, and transportation and future mobility.
Dr. Chengchao Bai
Prof. Dr. Quanbo Ge
Prof. Dr. Hongbo Gao
Prof. Dr. Ming He
Guest Editors
Manuscript Submission Information
Manuscripts should be submitted online at www.mdpi.com by registering and logging in to this website. Once you are registered, click here to go to the submission form. Manuscripts can be submitted until the deadline. All submissions that pass pre-check are peer-reviewed. Accepted papers will be published continuously in the journal (as soon as accepted) and will be listed together on the special issue website. Research articles, review articles as well as short communications are invited. For planned papers, a title and short abstract (about 100 words) can be sent to the Editorial Office for announcement on this website.
Submitted manuscripts should not have been published previously, nor be under consideration for publication elsewhere (except conference proceedings papers). All manuscripts are thoroughly refereed through a single-blind peer-review process. A guide for authors and other relevant information for submission of manuscripts is available on the Instructions for Authors page. Applied Sciences is an international peer-reviewed open access semimonthly journal published by MDPI.
Please visit the Instructions for Authors page before submitting a manuscript. The Article Processing Charge (APC) for publication in this open access journal is 2400 CHF (Swiss Francs). Submitted papers should be well formatted and use good English. Authors may use MDPI's English editing service prior to publication or during author revisions.
Keywords
- unmanned system
- artificial intelligence
- machine learning
- motion planning
- mechanical design
- communications engineering
- robotics and automation
- aerospace science and engineering
- marine science and engineering
- transportation and future mobility