Traffic Prediction and Route Guidance
A special issue of Applied Sciences (ISSN 2076-3417). This special issue belongs to the section "Transportation and Future Mobility".
Deadline for manuscript submissions: closed (20 July 2022) | Viewed by 17345
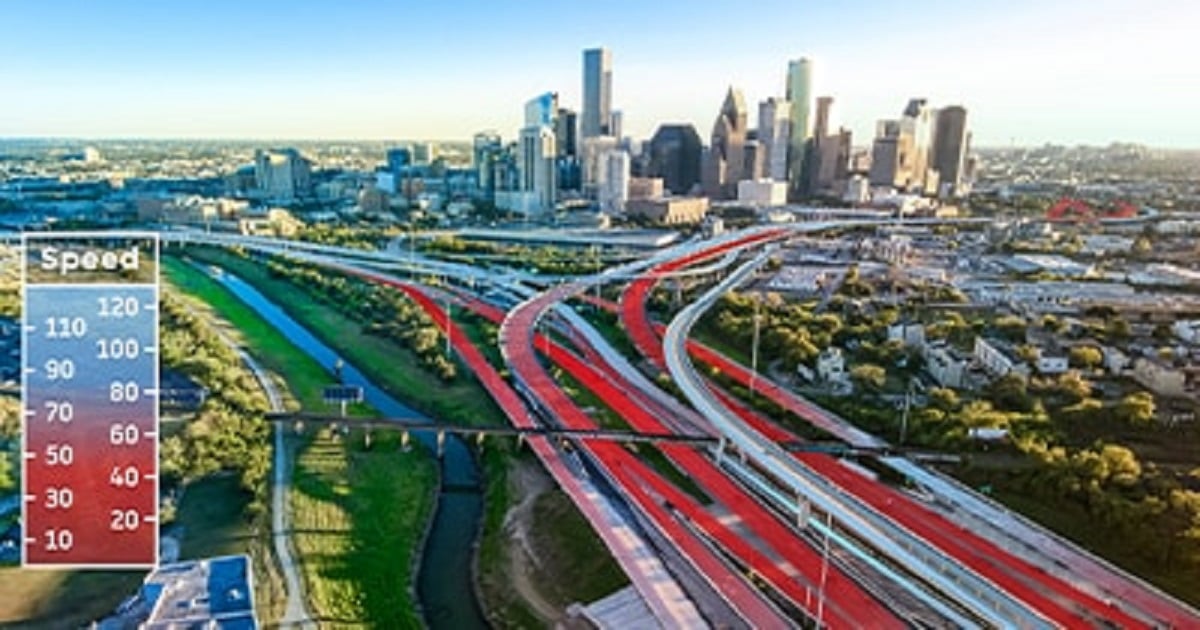
Special Issue Editor
Interests: automated vehicles; intelligent transportation system; traffic control
Special Issues, Collections and Topics in MDPI journals
Special Issue Information
Dear Colleagues,
Traffic congestion costs people valuable time, fuel, and frustration every single day. At the same time, large amounts of congestion impact governments who need to keep traffic flowing for the movement of goods, reducing pollution in certain areas, and for the safety of those on the road. Congestion is a global problem that impacts all levels of society. Intelligent transportation systems (ITS) including infrastructure and services, as well as the planning, operation, and control methods is to optimize transportation and to increase the safety of transportation. More and more drivers are relying on navigation applications and devices to guide them through the fastest routes and avoid hitting congestion. The best navigation devices use advanced traffic prediction services for accurate estimated times of arrivals (ETAs) and optimized routes during a driver’s journey.
Traffic prediction is the task of forecasting real-time traffic information based on floating car data and historical traffic data, such as traffic flow, average traffic speed and traffic incidents. Recent technological advances applying the various methodologies of AI, machine learning in navigation systems for vehicles have the capability to provide drivers with route information. These technological advances, together with two-way radio communication of digital information, automatic measurement of traffic flows, and supercomputer technology, could be combined to provide useful information to drivers concerning expected travel times, best routes, and best departure times.
The aim of this Special Issue is to collect papers describing technological tools currently applied in traffic prediction and route guidance.
Dr. Sehyun Tak
Guest Editor
Manuscript Submission Information
Manuscripts should be submitted online at www.mdpi.com by registering and logging in to this website. Once you are registered, click here to go to the submission form. Manuscripts can be submitted until the deadline. All submissions that pass pre-check are peer-reviewed. Accepted papers will be published continuously in the journal (as soon as accepted) and will be listed together on the special issue website. Research articles, review articles as well as short communications are invited. For planned papers, a title and short abstract (about 100 words) can be sent to the Editorial Office for announcement on this website.
Submitted manuscripts should not have been published previously, nor be under consideration for publication elsewhere (except conference proceedings papers). All manuscripts are thoroughly refereed through a single-blind peer-review process. A guide for authors and other relevant information for submission of manuscripts is available on the Instructions for Authors page. Applied Sciences is an international peer-reviewed open access semimonthly journal published by MDPI.
Please visit the Instructions for Authors page before submitting a manuscript. The Article Processing Charge (APC) for publication in this open access journal is 2400 CHF (Swiss Francs). Submitted papers should be well formatted and use good English. Authors may use MDPI's English editing service prior to publication or during author revisions.
Keywords
- traffic prediction
- route guidance
- traffic control
- traffic simulation
- pedestrian simulation
- autonomous vehicle
- traffic congestion
- intelligent transportation
- traffic flow
- traffic data
- machine learning
- Big Data transportation