Visualization Technologies in Deep Learning
A special issue of Applied Sciences (ISSN 2076-3417). This special issue belongs to the section "Computing and Artificial Intelligence".
Deadline for manuscript submissions: closed (10 April 2023) | Viewed by 2348
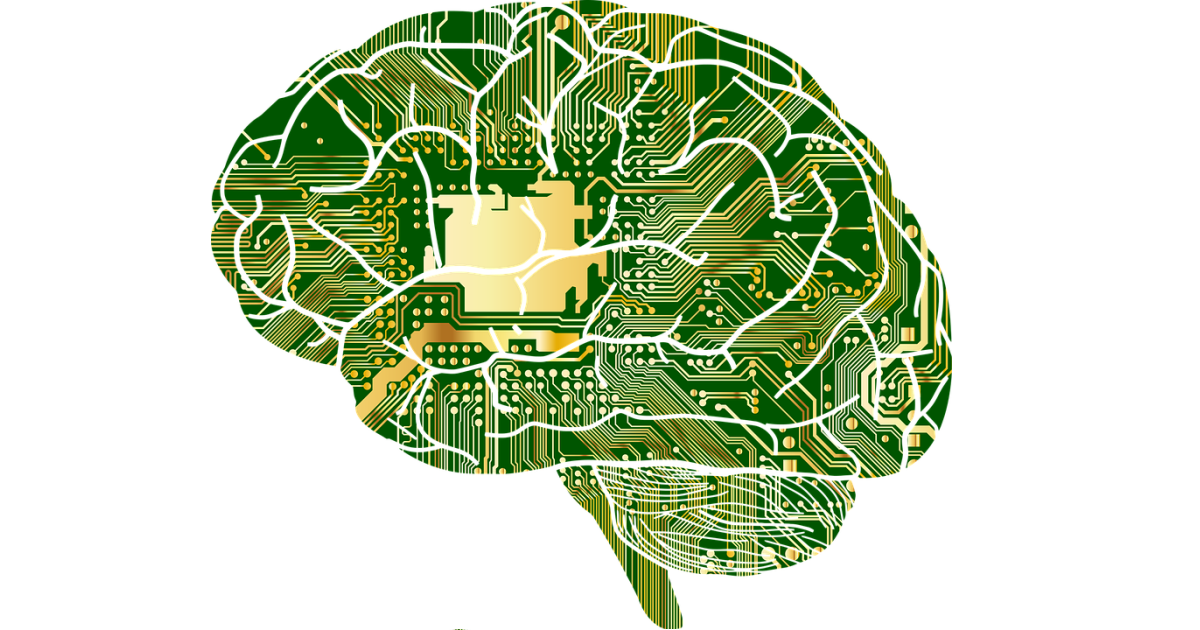
Special Issue Editors
Interests: artificial intelligence; big data; machine learning; data mining; virtual reality; human–computer interaction
Interests: visualization; visual analytics
Special Issue Information
Dear Colleagues,
This Special Issue is devoted to visualization technologies in deep learning. Over recent years, deep learning has been widely applied in a variety of fields ranging from facial recognition and strategy games to shopping recommendations and answering questions. However, the effectiveness and efficiency of the training models are still difficult to manage because they serve as black boxes, providing little insight into how, why, and when they are successful. Visualization is an effective way to present the internal features in the objects and events, which will be quite suitable for enabling users to obtain insights into the training courses available and the usefulness of models. Thus, we are pleased to announce this Special Issue, “Visualization Technologies in Deep Learning”, in which works focusing on DL4Vis (deep-learning-based visualization) and VIS4DL (visualization methods for the interpretation of deep learning models) are welcome. Hope that this Special Issue will assist the promotion and utilization of visualization and deep learning applications.
Prof. Dr. Zhiguang Zhou
Prof. Dr. Fangfang Zhou
Dr. Chenhui Li
Guest Editors
Manuscript Submission Information
Manuscripts should be submitted online at www.mdpi.com by registering and logging in to this website. Once you are registered, click here to go to the submission form. Manuscripts can be submitted until the deadline. All submissions that pass pre-check are peer-reviewed. Accepted papers will be published continuously in the journal (as soon as accepted) and will be listed together on the special issue website. Research articles, review articles as well as short communications are invited. For planned papers, a title and short abstract (about 100 words) can be sent to the Editorial Office for announcement on this website.
Submitted manuscripts should not have been published previously, nor be under consideration for publication elsewhere (except conference proceedings papers). All manuscripts are thoroughly refereed through a single-blind peer-review process. A guide for authors and other relevant information for submission of manuscripts is available on the Instructions for Authors page. Applied Sciences is an international peer-reviewed open access semimonthly journal published by MDPI.
Please visit the Instructions for Authors page before submitting a manuscript. The Article Processing Charge (APC) for publication in this open access journal is 2400 CHF (Swiss Francs). Submitted papers should be well formatted and use good English. Authors may use MDPI's English editing service prior to publication or during author revisions.
Keywords
- deep learning
- visualization
- visual analytics
- human–computer interaction
- data mining
- machine learning