Development and Applications of AI on Legal Tech
A special issue of Applied Sciences (ISSN 2076-3417). This special issue belongs to the section "Computing and Artificial Intelligence".
Deadline for manuscript submissions: closed (22 April 2022) | Viewed by 9515
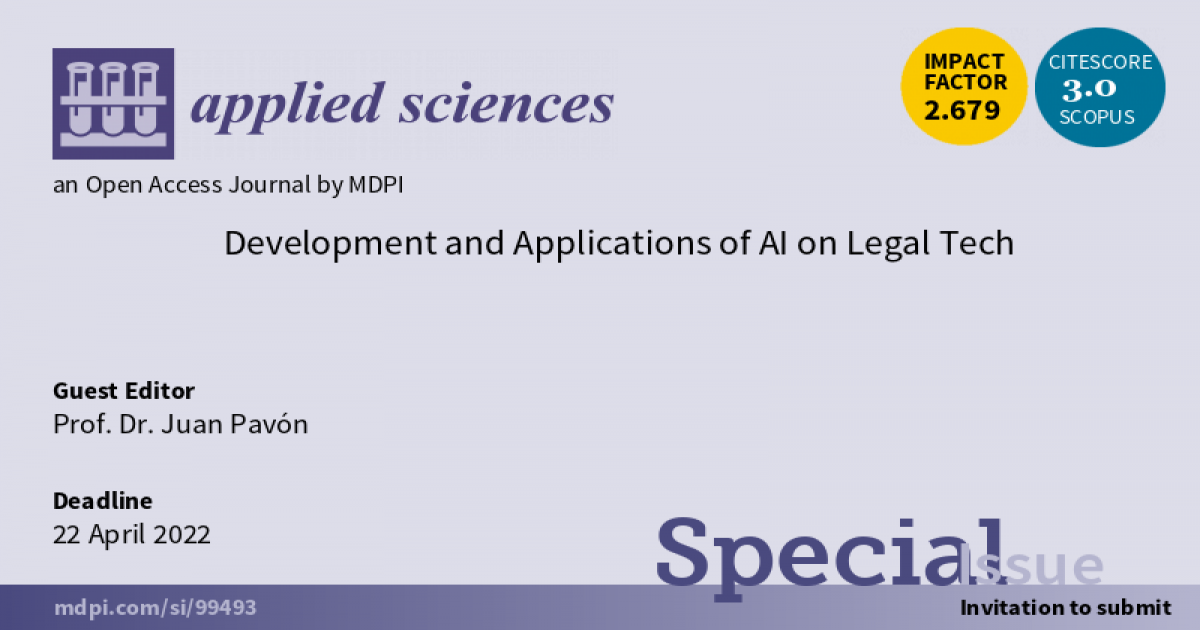
Special Issue Editor
Special Issue Information
Dear Colleagues,
Artificially intelligent (AI) techniques are applied more and more in different fields of our society. In the legal sector, AI is automating traditional legal work and has also offered opportunities to implement new services to support the legal professionals, paving the way to a new generation of LegalTech systems. Further, people can take advantage of the application of AI, with new services offered by public administrations, for instance, making legal decisions more transparent and facilitating easier access to justice. However, some risks have been identified, for instance, as a result of the bias that some data may induce in the learning process of intelligent systems, or the need for auditing AI tools to guarantee their value.
This Special Issue collects original articles addressing the emergence of new technologies and services, paradigms, and concepts of Legal Tech, by applying AI. Both theoretical and experimental contributions are welcome, as well as research papers or reviews.
Prof. Dr. Juan Pavón
Guest Editor
Manuscript Submission Information
Manuscripts should be submitted online at www.mdpi.com by registering and logging in to this website. Once you are registered, click here to go to the submission form. Manuscripts can be submitted until the deadline. All submissions that pass pre-check are peer-reviewed. Accepted papers will be published continuously in the journal (as soon as accepted) and will be listed together on the special issue website. Research articles, review articles as well as short communications are invited. For planned papers, a title and short abstract (about 100 words) can be sent to the Editorial Office for announcement on this website.
Submitted manuscripts should not have been published previously, nor be under consideration for publication elsewhere (except conference proceedings papers). All manuscripts are thoroughly refereed through a single-blind peer-review process. A guide for authors and other relevant information for submission of manuscripts is available on the Instructions for Authors page. Applied Sciences is an international peer-reviewed open access semimonthly journal published by MDPI.
Please visit the Instructions for Authors page before submitting a manuscript. The Article Processing Charge (APC) for publication in this open access journal is 2400 CHF (Swiss Francs). Submitted papers should be well formatted and use good English. Authors may use MDPI's English editing service prior to publication or during author revisions.