Topic Menu
► Topic MenuTopic Editors

Machine Learning in Internet of Things
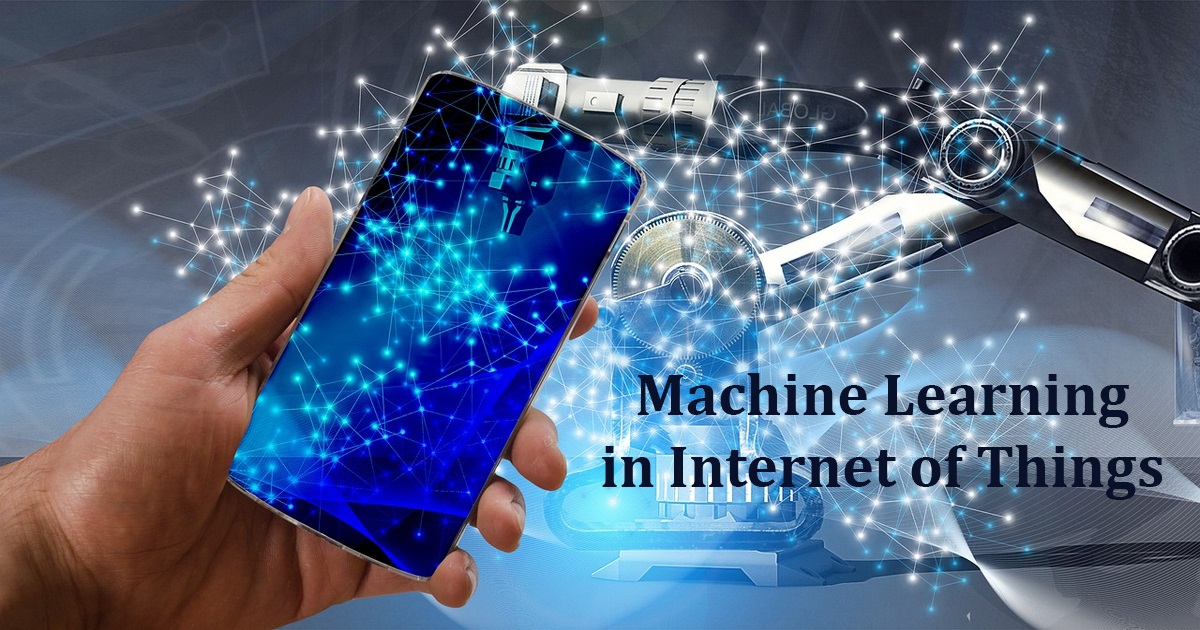
Image courtesy of no need
Topic Information
Dear Colleagues,
Technological development has contributed to the enormous amount of data generated by intelligent robots, sensors, cameras, etc. Additionally, this has brought new challenges related to their analysis and understanding, which are especially important due to their practical uses. To make their use possible, there is a need to process, classify, and understand them quickly and effectively.
At the same time, attention should be paid to the diversity of data and their potential for various problems. Data are not always perfect or in large amounts. Hence, with various methods of augmentation, GAN networks find their applications. However, it is not always possible to obtain high efficiencies. For this purpose, new solutions in machine learning and data analysis are emerging. Moreover, the training process is often demanding due to the selection of parameters, the amount of training data, or even the training time. Recent years have brought the idea of federated learning, which enables the training of one model on many clients while maintaining data privacy. However, implementing the solution in practice is associated with transmission security, data poisoning attacks, or even the selection of the model aggregation method.
Improving existing methods and proposing new solutions automate various processes and analyses. Such methods are one of the basic assumptions of intelligent solutions in various disciplines and the Internet of things. Hence, the topics of machine learning, optimization techniques, data processing/analysis, and, above all, the use of artificial intelligence methods in practical IoT solutions are the basic topics of this multidisciplinary topic. The theoretical and practical aspects of the application of intelligent solutions are needed to improve the current state of knowledge; hence, topics related to machine learning, security, and data mining in such systems are welcome.
Dr. Dawid Połap
Dr. Robertas Damasevicius
Dr. Hafiz Tayyab Rauf
Topic Editors
Keywords
- 6G
- artificial intelligence
- augmented reality or virtual reality
- bioinformatics, biosensors, biomarkers
- computational intelligence
- data augmentation, data fusion and data mining
- decision support systems and theory
- dron application
- edge computing
- explainable AI Federated learning
- transfer learning
- fuzzy logic
- swarm intelligence
- hybrid systems
- mobile applications
Participating Journals
Journal Name | Impact Factor | CiteScore | Launched Year | First Decision (median) | APC |
---|---|---|---|---|---|
![]()
AI
|
- | - | 2020 | 20.8 Days | CHF 1600 |
![]()
Electronics
|
2.9 | 4.7 | 2012 | 15.6 Days | CHF 2400 |
![]()
IoT
|
- | 5.2 | 2020 | 23.3 Days | CHF 1200 |
![]()
Journal of Sensor and Actuator Networks
|
3.5 | 7.6 | 2012 | 20.4 Days | CHF 2000 |
![]()
Remote Sensing
|
5.0 | 7.9 | 2009 | 23 Days | CHF 2700 |
![]()
Sensors
|
3.9 | 6.8 | 2001 | 17 Days | CHF 2600 |
MDPI Topics is cooperating with Preprints.org and has built a direct connection between MDPI journals and Preprints.org. Authors are encouraged to enjoy the benefits by posting a preprint at Preprints.org prior to publication:
- Immediately share your ideas ahead of publication and establish your research priority;
- Protect your idea from being stolen with this time-stamped preprint article;
- Enhance the exposure and impact of your research;
- Receive feedback from your peers in advance;
- Have it indexed in Web of Science (Preprint Citation Index), Google Scholar, Crossref, SHARE, PrePubMed, Scilit and Europe PMC.