Topic Menu
► Topic MenuTopic Editors


Machine Learning in Communication Systems and Networks
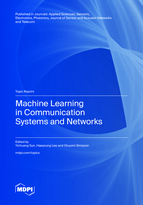
A printed edition is available here.
Topic Information
Dear Colleagues,
Recent advances in machine learning, including the availability of powerful computing platforms, have received huge attention from related academic, research and industry communities. Machine learning is considered as a promising tool to tackle the challenge in increasingly complex, heterogeneous and dynamic communication environments. Machine learning would be able to contribute to intelligent management and optimization of communication systems and networks by enabling us to predict changes, find patterns of uncertainties in the communication environment, and make data-driven decisions.
This Topic will focus on machine learning-based solutions to manage complex issues in communication systems and networks across various layers and within various ranges of communication applications. The objective of the Topic is to share and discuss recent advances and future trends of machine learning for intelligent communication. Original studies (unpublished and not currently under review by another journal) are welcome in relevant areas, including (but not limited to) the following:
- Fundamental limits of machine learning in communication.
- Design and implementation of advanced machine learning algorithms (including distributed learning) in communication.
- Machine learning for physical layer and cross-layer processing (e.g., channel modeling and estimation, interference avoidance, beamforming and antenna configuration, etc.).
- Machine learning for adaptive radio resource allocation and optimization.
- Machine learning for network slicing, virtualization and software defined networking.
- Service performance optimization and evaluation of machine learning based solutions in various vertical applications (e.g., healthcare, transport, aquaculture, farming, etc.).
- Machine learning for anomaly detection in communication systems and networks.
- Security, privacy and trust of machine learning over communication systems and networks.
Prof. Dr. Yichuang Sun
Dr. Haeyoung Lee
Dr. Oluyomi Simpson
Topic Editors
Keywords
- wireless communications
- mobile communications
- vehicular communications
- 5G/6G systems and networks
- artificial intelligence
- machine learning
- deep learning
Participating Journals
Journal Name | Impact Factor | CiteScore | Launched Year | First Decision (median) | APC |
---|---|---|---|---|---|
![]()
Applied Sciences
|
2.5 | 5.3 | 2011 | 18.4 Days | CHF 2400 |
![]()
Sensors
|
3.4 | 7.3 | 2001 | 18.6 Days | CHF 2600 |
![]()
Electronics
|
2.6 | 5.3 | 2012 | 16.4 Days | CHF 2400 |
![]()
Photonics
|
2.1 | 2.6 | 2014 | 14.9 Days | CHF 2400 |
![]()
Journal of Sensor and Actuator Networks
|
3.3 | 7.9 | 2012 | 19 Days | CHF 2000 |
![]()
Telecom
|
2.1 | 4.8 | 2020 | 20.5 Days | CHF 1200 |
MDPI Topics is cooperating with Preprints.org and has built a direct connection between MDPI journals and Preprints.org. Authors are encouraged to enjoy the benefits by posting a preprint at Preprints.org prior to publication:
- Immediately share your ideas ahead of publication and establish your research priority;
- Protect your idea from being stolen with this time-stamped preprint article;
- Enhance the exposure and impact of your research;
- Receive feedback from your peers in advance;
- Have it indexed in Web of Science (Preprint Citation Index), Google Scholar, Crossref, SHARE, PrePubMed, Scilit and Europe PMC.