Privacy and Security in Machine Learning and Artificial Intelligence
A special issue of Applied Sciences (ISSN 2076-3417). This special issue belongs to the section "Computing and Artificial Intelligence".
Deadline for manuscript submissions: closed (20 October 2024) | Viewed by 4254
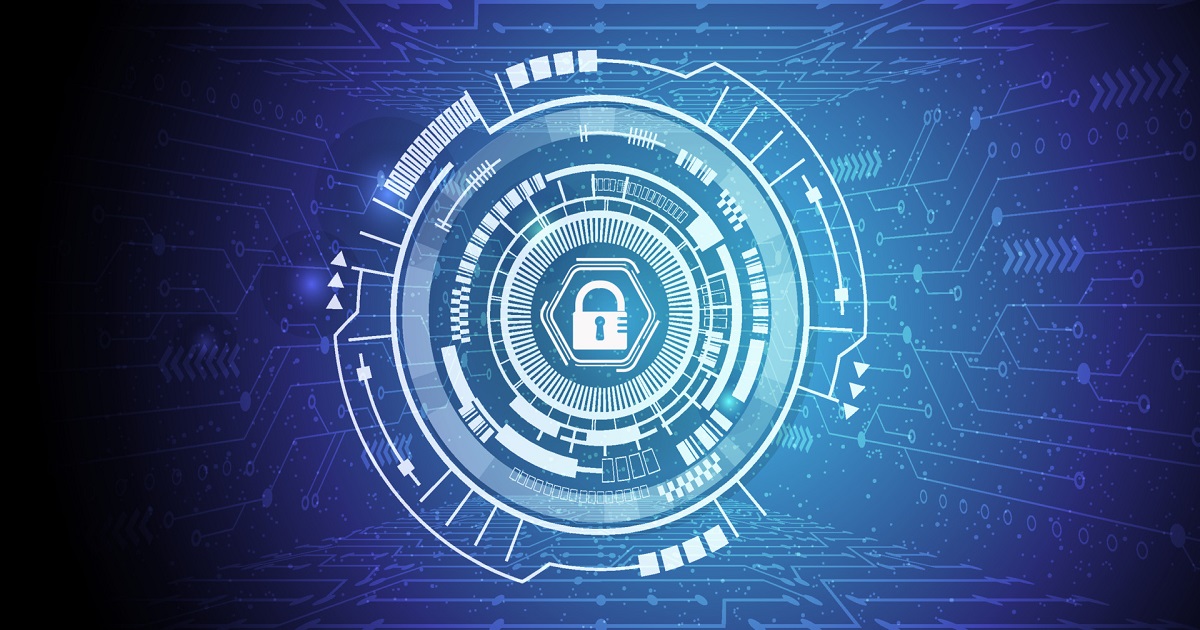
Special Issue Editor
Special Issue Information
Dear Colleagues,
The development of Artificial Intelligence (AI) and its learning techniques, such as Machine Learning (ML) and Deep Learning (DL), have revolutionized data processing and analysis. This transformation is rapidly changing human life and has allowed for many practical applications based on AI, including the Internet of Things/Vehicles (IoT/ IoV), smart grid and energy saving, fog/edge computing, face/image recognition, text/sentimental analysis, attack detection, and healthcare.
However, the potential benefits of AI are hindered by issues such as insecurity, bias, unreliability, and privacy violations in data processing and communication. This negative impact affects both AI applications and society as a consequence. To address these concerns, this Special Issue seeks novel ideas, findings, and envisions the future of private and secure machine learning and AI.
The Special Issue will focus on and welcome submissions on topics such as privacy-preserving machine learning, deep learning, federated learning, trustworthy machine learning, metrics in private, secure and trustworthy AI, adversarial attacks against AI models, cryptography and security protocols in AI, privacy by design in AI-based systems, and applications of private, secure, and trustworthy AI.
Dr. Mina Sheikhalishahi
Guest Editor
Manuscript Submission Information
Manuscripts should be submitted online at www.mdpi.com by registering and logging in to this website. Once you are registered, click here to go to the submission form. Manuscripts can be submitted until the deadline. All submissions that pass pre-check are peer-reviewed. Accepted papers will be published continuously in the journal (as soon as accepted) and will be listed together on the special issue website. Research articles, review articles as well as short communications are invited. For planned papers, a title and short abstract (about 100 words) can be sent to the Editorial Office for announcement on this website.
Submitted manuscripts should not have been published previously, nor be under consideration for publication elsewhere (except conference proceedings papers). All manuscripts are thoroughly refereed through a single-blind peer-review process. A guide for authors and other relevant information for submission of manuscripts is available on the Instructions for Authors page. Applied Sciences is an international peer-reviewed open access semimonthly journal published by MDPI.
Please visit the Instructions for Authors page before submitting a manuscript. The Article Processing Charge (APC) for publication in this open access journal is 2400 CHF (Swiss Francs). Submitted papers should be well formatted and use good English. Authors may use MDPI's English editing service prior to publication or during author revisions.
Keywords
- privacy
- trustworthy AI
- federated learning
- AI security
Benefits of Publishing in a Special Issue
- Ease of navigation: Grouping papers by topic helps scholars navigate broad scope journals more efficiently.
- Greater discoverability: Special Issues support the reach and impact of scientific research. Articles in Special Issues are more discoverable and cited more frequently.
- Expansion of research network: Special Issues facilitate connections among authors, fostering scientific collaborations.
- External promotion: Articles in Special Issues are often promoted through the journal's social media, increasing their visibility.
- e-Book format: Special Issues with more than 10 articles can be published as dedicated e-books, ensuring wide and rapid dissemination.
Further information on MDPI's Special Issue polices can be found here.