AI in Clean Energy Systems
A special issue of Clean Technologies (ISSN 2571-8797).
Deadline for manuscript submissions: closed (28 February 2022) | Viewed by 17187
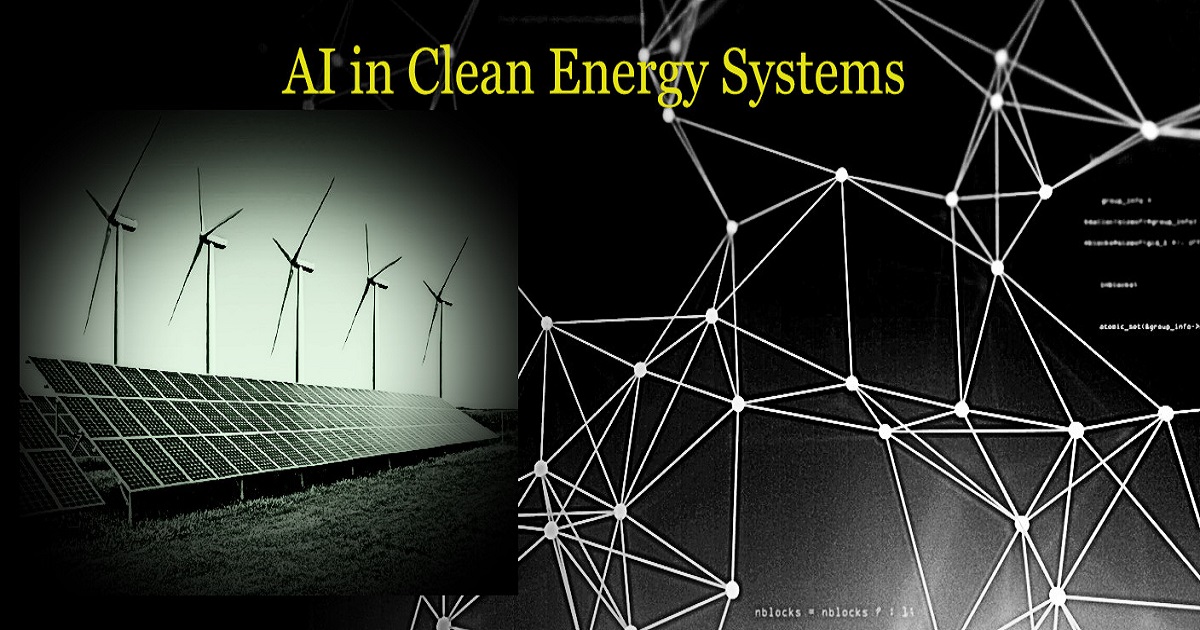
Special Issue Editors
Interests: fluid mechanics; computational fluid dynamics; numerical simulation; CFD simulation; numerical analysis; engineering thermodynamics; thermal engineering; numerical modeling
Special Issues, Collections and Topics in MDPI journals
Interests: green supply chain; sustainability management; clean waste management; clean energy supply chain; renewable energy supply chain management
Special Issues, Collections and Topics in MDPI journals
Special Issue Information
Dear Colleagues,
Artificial intelligence (AI) is applied in many fields, including clean energies. AI enables clean energy systems to collect, handle, and process a vast quantity of data. This leads to new technologies and policies that improve the efficiency, distribution, and conversion of clean energy systems. Machine learning as the most common approach in AI and new technologies are developed to study and analyze different aspects of clean energy systems, such as system control, optimization, system design, supply chain design, cost minimization, distribution management, policy design, and socio-economic planning. For example, new forecasting methods provide a better prediction of wind farm renewable energy production, making energy grid design easier. AI has improved automation in clean energy systems. This increases efficiency, particularly in solar and wind energy systems. Cost-saving and power generation increasing are advantages of using AI-based automation systems.
This Special Issue "AI in Clean Energy Systems" will publish new studies using AI approaches to explore, produce, distribute, and consume clean energies, including wind, solar, wave, geothermal, and hydropower.
We invite all researchers active in the broad and captivating AI and Clean Energy Systems domain to submit articles for this Special Issue of Clean Technologies journal.
Dr. Marjan Goodarzi
Dr. Reza Maihami
Guest Editors
Manuscript Submission Information
Manuscripts should be submitted online at www.mdpi.com by registering and logging in to this website. Once you are registered, click here to go to the submission form. Manuscripts can be submitted until the deadline. All submissions that pass pre-check are peer-reviewed. Accepted papers will be published continuously in the journal (as soon as accepted) and will be listed together on the special issue website. Research articles, review articles as well as short communications are invited. For planned papers, a title and short abstract (about 100 words) can be sent to the Editorial Office for announcement on this website.
Submitted manuscripts should not have been published previously, nor be under consideration for publication elsewhere (except conference proceedings papers). All manuscripts are thoroughly refereed through a single-blind peer-review process. A guide for authors and other relevant information for submission of manuscripts is available on the Instructions for Authors page. Clean Technologies is an international peer-reviewed open access quarterly journal published by MDPI.
Please visit the Instructions for Authors page before submitting a manuscript. The Article Processing Charge (APC) for publication in this open access journal is 1600 CHF (Swiss Francs). Submitted papers should be well formatted and use good English. Authors may use MDPI's English editing service prior to publication or during author revisions.
Keywords
- clean energy
- AI
- machine learning
- system design
- generating and integration clean energy
- plasma process
- clean fuel production
- clean energy life cycle assessment (LCA)
- sustainable supply chain planning