SREDet: Semantic-Driven Rotational Feature Enhancement for Oriented Object Detection in Remote Sensing Images
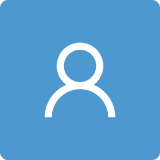
Round 1
Reviewer 1 Report
Comments and Suggestions for AuthorsThis paper proposes an oriented object detection method for remote sensing images that utilizes both semantic and spatial information. The idea is interesting and the authors evaluate its effectiveness with sufficient experiments. However, there are some issues that the authors should address.
1. Despite conventional rotational object detection loss, the loss function of this method also incorporates the segmentation loss. Have you experimented with other segmentation loss functions, and if so, what about the results? Please conduct an ablation experiment to demonstrate the performance advantages of this segmentation loss function.
2. Several methods have been proposed in recent years for rotation-invariant feature extraction of remote sensing images, such as “Rotation-invariant feature learning via convolutional neural network with cyclic polar coordinates convolutional layer, T-GRS, 2023”. The authors are recommended to introduce these related work more comprehensively in the paper.
3. The MRFPN module in the paper utilizes the deformable convolution. Please provide further explanation regarding the utility of deformable convolution. Besides, it is necessary to conduct comparative experiments with conventional convolution to demonstrate the effectiveness of deformable convolution.
4. Figure 2 lacks annotations for the four directional feature maps, which should be added to facilitate understanding. Additionally, the formatting style of Table 5 should be consistent with that of the other tables. Please carefully review all the figures and tables in the paper to ensure they are clear and well-defined.
Comments on the Quality of English LanguageN/A
Author Response
The Response Letter for Remote Sensing Reviewer
We would like to sincerely thank the editor and all reviewers for the positive comments, valuable suggestions, and inspiring criticisms. We believe that all comments raised in the review report have been carefully accommodated to the best of our knowledge. Newly added or modified text is indicated by red highlighting in the revised text, and the point-by-point responses to all comments are as follows. We incorporate all the changes that are highlighted in the new version of the manuscript. We believe this version is in much better shape and hope it is satisfactory for publication in this reputed journal.
Response to the Reviewer 1
Question 1: Despite conventional rotational object detection loss, the loss function of this method also incorporates the segmentation loss. Have you experimented with other segmentation loss functions, and if so, what about the results? Please conduct an ablation experiment to demonstrate the performance advantages of this segmentation loss function.
Response 1: In this article, we proposed a method for implicitly generating weights using semantic segmentation information to enhance feature maps. Therefore, the accuracy of semantic segmentation directly affects the network's performance. In the SFEM module, we tested three different losses. By comparison, it can be seen that without adjusting the loss weights, Focal loss performs best on the DOTA dataset for the class imbalance in remote sensing images. However, considering that Dice loss has a stronger ability to distinguish target regions, and based on our statistics, background pixels account for 96.95\% of the dataset. We introduced weights to Dice loss by setting the classification weight of background pixels to 1 and foreground pixels to 20. The experimental results showed that this approach achieved the best performance.
The discussed contents have been added in the revised version, and they can be found in section 4.3.3, Table 8.
Question 2: Several methods have been proposed in recent years for rotation-invariant feature extraction of remote sensing images, such as “Rotation-invariant feature learning via convolutional neural network with cyclic polar coordinates convolutional layer, T-GRS, 2023”. The authors are recommended to introduce these related works more comprehensively in the paper.
Response 2: Thank you for your suggestions. We have carefully reviewed the papers you recommended and incorporated them into our article to enrich the related work section and better summarize the development trends in the relevant field.
The discussed contents have been added in the revised version, and they can be found in section 2.2.
Question 3: The MRFPN module in the paper utilizes the deformable convolution. Please provide further explanation regarding the utility of deformable convolution. Besides, it is necessary to conduct comparative experiments with conventional convolution to demonstrate the effectiveness of deformable convolution?
Response 3: In the MRFPN, we tested different numbers of feature layers and compared standard convolutions with deformable convolutions. The experiments revealed that when using standard convolutions, there was no significant difference in performance between using four and five feature layers. However, after employing DCN for feature extraction, additional feature layers improved the network's performance. This improvement is primarily attributed to the DCN's enhanced capability to extract features from irregular targets.
The discussed contents have been added in the revised version, and they can be found in section 4.3.3, Table 6.
Question 4: Figure 2 lacks annotations for the four directional feature maps, which should be added to facilitate understanding. Additionally, the formatting style of Table 5 should be consistent with that of the other tables. Please carefully review all the figures and tables in the paper to ensure they are clear and well-defined.
Response 4: Thank you for your careful review. In the caption of Figure 2, we have added annotations using four different colors to represent feature maps at different angles. Additionally, we have adjusted the style of Table 5 to be consistent with the styles of the other tables.
The discussed contents have been added in the revised version, and they can be found in section 3, Figure 2, and section 4.3.3, Table 5.
We express our gratitude for your insightful comments, which have proven invaluable in the revision and enhancement of our article.
Author Response File: Author Response.pdf
Reviewer 2 Report
Comments and Suggestions for AuthorsThis paper presents a novel oriented object detection algorithm, primarily designing two modules: MRFPN and SFEM. Additionally, it introduces an evaluation metric for different error types. The proposed method iseffective and scientifically sound. The experimental design and results are reasonable. Some parts in the article confuse me and need additional clarification.
1. The summary of attention mechanism-related technologies in the paper is not comprehensive enough and should be further supplemented. 2. The authors are requested to provide a more detailed explanation of the implicit and explicit enhancement strategies, as the current description is not clear enough. 3. The network currently uses five different scales of feature maps. Is it necessary to use so many layers of feature maps for detection? It is encouraged to provide relevant experimental verification. 4. The paper proposes the SFEM module to enhance feature maps using semantic segmentation information, validating on a single-stage algorithm. Can this module be introduced into a two-stage algorithm for testing? 5. It is recommended to bold the maximum values in the tables to enhance the readability of the paper. 6. Please check the specific terms in the paper and define with clear explanation. For example, the meaning of RX-101 in Table 1 is not descripted.
Author Response
The Response Letter for Remote Sensing Reviewer
We would like to sincerely thank the editor and all reviewers for the positive comments, valuable suggestions, and inspiring criticisms. We believe that all comments raised in the review report have been carefully accommodated to the best of our knowledge. Newly added or modified text is indicated by red highlighting in the revised text, and the point-by-point responses to all comments are as follows. We incorporate all the changes that are highlighted in the new version of the manuscript. We believe this version is in much better shape and hope it is satisfactory for publication in this reputed journal.
Response to the Reviewer 2
Question 1: The summary of attention mechanism-related technologies in the paper is not comprehensive enough and should be further supplemented.
Response 1: Thank you for your suggestions. We have carefully reviewed the latest literature related to the field of attention mechanisms and have added relevant content to our article.
Question 2: The authors are requested to provide a more detailed explanation of the implicit and explicit enhancement strategies, as the current description is not clear enough.
Response 2: We have supplemented and clearly defined the methods of implicit and explicit enhancement in the revised manuscript. We define the method of directly multiplying the predicted semantic information probabilities with the feature maps in the spatial domain as an explicit feature enhancement. Conversely, the approach of generating a set of weights from the semantic information feature maps and then weighting the feature maps accordingly is defined as an implicit enhancement.
The discussed contents have been added in the revised version, and they can be found in section 3.2.
Question 3: The network currently uses five different scales of feature maps. Is it necessary to use so many layers of feature maps for detection? It is encouraged to provide relevant experimental verification.
Response 3: We tested different numbers of feature layers and compared the use of standard convolutions with deformable convolutions. The experiments revealed that when using standard convolutions, there was no significant difference in performance between using four and five feature layers. However, after employing DCN for feature extraction, additional feature layers improved the network's performance. This improvement is primarily attributed to the DCN's enhanced capability to extract features from irregular targets.
The discussed contents have been added in the revised version, and they can be found in section 4.3.3, Table 6.
Question 4: The paper proposes the SFEM module to enhance feature maps using semantic segmentation information, validating on a single-stage algorithm. Can this module be introduced into a two-stage algorithm for testing?
Response 4: We conducted comparative experiments by integrating the SFEM into different baseline models, including the two-stage detection algorithm Faster R-CNN. Our proposed module improved performance by 0.88 on Faster R-CNN, although the enhancement was less pronounced compared to the single-stage model. This is primarily because the RPN operation in two-stage algorithms helps the network focus on key feature areas of the target, rather than detecting across the entire feature map.
The discussed contents have been added in the revised version, and they can be found in section 4.3.3, Table 9.
Question 5: It is recommended to bold the maximum values in the tables to enhance the readability of the paper.
Response 5: Thank you for your careful review, We have bolded the best experimental results in the experimental tables.
Question 6: Please check the specific terms in the paper and define with clear explanation. For example, the meaning of RX-101 in Table 1 is not descripted.
Response 6: Thank you for your careful review. We have supplemented the definitions of relevant abbreviations in Table 1. RX-101 denotes ResNeXt-101
The discussed contents have been added in the revised version, and they can be found in section 4.3.1, Table 1.
Special thanks to you for all your good comments that are very helpful for revising and improving our article.
Author Response File: Author Response.pdf
Reviewer 3 Report
Comments and Suggestions for AuthorsThe article is well prepared and well argued. I suggest it be published.
Author Response
The Response Letter for Remote Sensing Reviewer
We would like to sincerely thank the editor and all reviewers for the positive comments, valuable suggestions, and inspiring criticisms. We believe that all comments raised in the review report have been carefully accommodated to the best of our knowledge. Newly added or modified text is indicated by red highlighting in the revised text, and the point-by-point responses to all comments are as follows. We incorporate all the changes that are highlighted in the new version of the manuscript. We believe this version is in much better shape and hope it is satisfactory for publication in this reputed journal.
Response to the Reviewer 3
Thank you very much for your positive evaluation of our manuscript. We are delighted to hear that it has met your standards and has been accepted without further revisions. Your support and the smooth review process are greatly appreciated. We are looking forward to seeing our work contribute to the field and are grateful for the opportunity to share our findings with the community. Thank you once again for your time and consideration.
Author Response File: Author Response.pdf
Reviewer 4 Report
Comments and Suggestions for AuthorsThe paper “SREDet: Semantic-Driven Rotational Feature Enhancement for Oriented Object Detection in Remote Sensing Images” presents a novel deep neural network architecture designed to detect objects wrapped in an oriented rectangle. As a proof of the advantages offered by the new architecture, results obtained with two well-known datasets (DOTA and HRSC2016) and documented in the form of a workbench are presented in a comparative and ablaction study.
Methodologically the authors propose SREDet, based on a Multi-Rotation Feature Pyramid Network (MRFPN) architecture, introducing a Semantic-driven Feature Enhancement Module (SFEM) and propose the use of a new metric to evaluate the semantic segmentation task loss to supervise the SFEM module.
The main shortcoming that I see in the study and the proposed new architecture for the detection of oriented objects is not taking into account either in the state of the art or related works or in the comparisons to YOLOv8 OBB. In the same way that the authors have used to implement their proposal MMRotate, they could perform with the same datasets built from DOA and HRSC2016 with YOLOv8 OBB and present and discuss or comment the results.
Therefore, my main recommendation is to extend the scarce state of the art articles and implementations related to the problem of detecting objects in images using oriented rectangles and to include in the comparison the results of YOLOv8 OBB with the same dataset used. This will allow a better understanding of the limitations and strengths of the analyzed models.
I would also recommend the authors to review some sections of the paper where there is a high degree of similarity with other published work and to rewrite some paragraphs that appear to have been generated with the help of AI Generative.
Finally, to reinforce the last section of conclusions. It is perceived as scarce.
Author Response
The Response Letter for Remote Sensing Reviewer
We would like to sincerely thank the editor and all reviewers for the positive comments, valuable suggestions, and inspiring criticisms. We believe that all comments raised in the review report have been carefully accommodated to the best of our knowledge. Newly added or modified text is indicated by red highlighting in the revised text, and the point-by-point responses to all comments are as follows. We incorporate all the changes that are highlighted in the new version of the manuscript. We believe this version is in much better shape and hope it is satisfactory for publication in this reputed journal.
Response to the Reviewer 4
Question 1: my main recommendation is to extend the scarce state of the art articles and implementations related to the problem of detecting objects in images using oriented rectangles and to include in the comparison the results of YOLOv8 OBB with the same dataset used. This will allow a better understanding of the limitations and strengths of the analyzed models.
Response 1: Thank you for your suggestion. We conducted additional experiments on YOLOv8-m and YOLOv8-l. To ensure the fairness and accuracy of the experiments, we did not load pre-trained models during training and used the default data augmentation method of YOLOv8. All networks were trained on the training set and tested on the validation set. The experimental results show that after adding the SFEM module, the mAP50 increased by 0.61 and 0.76 respectively.
The discussed contents have been added in the revised version, and they can be found in section 4.3.3, Table 9.
Question 2: I would also recommend the authors to review some sections of the paper where there is a high degree of similarity with other published work and to rewrite some paragraphs that appear to have been generated with the help of AI Generative.
Response 2:
Thank you for your suggestion. We have conducted a plagiarism check and polished the language of the paper. The specific modifications are highlighted in red font in the manuscript.
Figure 1 The plagiarism check results after modifying
Question 3: Finally, to reinforce the last section of conclusions. It is perceived as scarce.
Response 3: Thank you for your suggestions. We have provided a more detailed summary in the conclusion section. The revised content is as follows:
We innovatively introduced semantic segmentation into the domain of oriented remote sensing object detection, proposing an effective Semantic-Driven Feature Enhancement Detector (SREDet). The MRFPN module extracts rotation-invariant features of remote sensing objects at various scales, accommodating remote sensing objects of different orientations and shapes. A feature enhancement module informed by semantic segmentation information is proposed; the SFEM module decouples the features of different objects into separate channels, thereby enhancing the features of each category of instances while simultaneously suppressing the background. We added the SFEM module to various network architectures and conducted experiments. The results showed improvements across all baseline models, demonstrating the effectiveness and adaptability of the designed module. Additionally, we have introduced error-type analysis methods from general object detection, providing more refined evaluation metrics for rotated object detection. This metric can demonstrate the network's ability to handle different types of errors, providing a guiding direction for further network improvements. Detailed ablation and comparative experiments were conducted on two public aviation datasets, DOTA and HRSC2016, where our approach achieved competitive performance for the oriented object detection task.
The discussed contents have been added in the revised version, and they can be found in section 5. Conclusions.
Special thanks to you for all your good comments that are very helpful for revising and improving our article.
Author Response File: Author Response.pdf
Round 2
Reviewer 2 Report
Comments and Suggestions for AuthorsThe authors properly address all my concerns.
Author Response
Thank you very much for your positive evaluation of our manuscript. We are delighted to hear that it has met your standards and has been accepted without further revisions. Your support and the smooth review process are greatly appreciated. We are looking forward to seeing our work contribute to the field and are grateful for the opportunity to share our findings with the community. Thank you once again for your time and consideration.
Author Response File: Author Response.pdf
Reviewer 4 Report
Comments and Suggestions for AuthorsThanks to the authors for the incorporation of the tests and comparative results of using one-step and two-step models such as YOLO v8 OBB. It is interesting to note that the use of SFEM module improves the results in that framework as well.
Regarding the first comment regarding expanding the state of the art or related work they have not followed the recommendation. Nor do we see that they have expanded the conclusions section significantly.
Given that the authors have been able to apply the SFEM module on YOLOv8, it would be nice if they could share in a public repository the code that allows implementing this modification on this framework.
Regarding rewrite some sections, sorry for not having made explicit which section looks very similar to others already published, it is the description of the datasets used DOTA and HRSC2016 as well as the subsequent implementation. In the current version there is no change in those parts that could, with little effort be rewritten and avoid that bad impression of similarity.
Author Response
Question 1: Regarding the first comment regarding expanding the state of the art or related work they have not followed the recommendation. Nor do we see that they have expanded the conclusions section significantly.
Response 1: Thank you for your suggestions. We have reorganized each subsection of the related work section and incorporated some classic articles and recent literature. Specifically, in Section 2.1, we have reorganized the development of rotation object detection and added relevant rotation object detection algorithms based on the transformer architecture. In Sections 2.2 and 2.3, we have reorganized the expression and narrative logic, while also incorporating the latest literature in the field.
In the conclusion section, we have also reorganized the content, focusing on summarizing the main contributions of the paper and discussing its shortcomings and future research directions.
The discussed contents have been added in the revised version, and they can be found in section 2 and section 5.
Question 2: Given that the authors have been able to apply the SFEM module on YOLOv8, it would be nice if they could share in a public repository the code that allows implementing this modification on this framework.
Response 2: We have uploaded the code to GitHub. The code link is https://github.com/ZehaoZhang-Uestc/seg_yolov8.git.
The discussed contents have been added in the revised version, and they can be found in the abstract.
Question 3: Regarding rewrite some sections, sorry for not having made explicit which section looks very similar to others already published, it is the description of the datasets used DOTA and HRSC2016 as well as the subsequent implementation. In the current version there is no change in those parts that could, with little effort be rewritten and avoid that bad impression of similarity.
Response 3: Thank you for your suggestions. We have rewritten the introductions of the DOTA and HRSC2016 datasets and provided further details on the experimental setup in our paper.
The discussed contents have been added in the revised version, and they can be found in section 4.1 and section 4.2.
Special thanks to you for all your good comments that are very helpful for revising and improving our article.
Author Response File: Author Response.pdf