Employing a Multilingual Transformer Model for Segmenting Unpunctuated Arabic Text
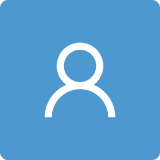
Round 1
Reviewer 1 Report
1. The abstract needs to be improved by indicating the main contribution.
2. In the Abstract, define terms before abbreviations (for example "punctuation detector for text splitting (PDTS) ").
3. Please check Equation (3).
4. The implementation of the proposed model should be explained in more detail and with clarity.
5. While the study was created based on PDTS, there are not enough criteria related to measuring the performance of the proposed method..
6. Tabel2 needs further clarification.
7. Please indicate the references of the equations (1-4)
8. To demonstrate the effectiveness of the proposed algorithm a real experimental validation is mandatory for a rigorous and accurate comparison and validation.
9. The conclusion must be rewritten.
10. English writing (e.g., grammar, spelling, wording, and sentencing) should be carefully checked when revising the manuscript.
Author Response
We are grateful for your thoughtful comments and greatly appreciate your time and effort invested in reviewing our manuscript. Below are point-by-point responses to your comments.
Author Response File: Author Response.pdf
Reviewer 2 Report
There are quite a few acronyms, like BERT and NLP that need to be defined for the reader. Also, the parenthetical, like (PDTS), needs to go after the idea it describes, like after "punctuation detection for text segmentation (PDTS)." In the lit review, provide a few examples of Arabic sentences and the segmentation that are described. For non-Arabic speakers, the issue of segmentation may see trivial, so describe the importance for Arabic orthography.
As for the drawbacks of approaches, like "The high computational effort consumed in generating linguistic annotation features," they are more about limitations with Arabic orthography, not a limitation of the approach to inquiry. On page 5, BERT is defined as "Bidirectional Encoder Representations from Transformers," but this is needed far earlier at the first mention of BERT.
What is the methodology of this study? Is this just a summary of research related the segmentation of Arabic in computer writing? Again, I think examples of punctuating Arabic sentences would illustrate this study better. On page 8, you write "The ultimate overarching purpose..." But, this is needed much earlier to structure this study. Although you utilized quantitative analysis, how does this address your RQ? In quantitative studies, there is a null hypothesis which you are disproving from a low p-value, but I do not see any test. The F-value or F-score in the chart are quite low. You typically would like to see a F-score of 3.95 or above. Also, the alpha level is way too high to be deemed solid research.
I would like to know how the "gold standard" was determined, because it seems quite subjective.
Author Response
We greatly appreciate your time and effort invested in reviewing our manuscript. Below are point-by-point responses to your comments.
Author Response File: Author Response.pdf
Round 2
Reviewer 1 Report
I accepted the paper
Author Response
Thank you so much
Reviewer 2 Report
My only issue is with the methods of this study. In an experimental procedure, the details of the participants, control group, experimental group, experimental procedure. Also, there seems to be too many different tests conducted, where one streamlined test would be better.
More space is needed for the methods section, especially in an experimental design. You need a control group, baseline scores, and other factors in such a design.
Author Response
We really appreciate the effort invested in reviewing our manuscript again. We understand your concern regarding the validity of our method, which is absolutely valid in some research methodologies, including social science studies.
Yes, your point of view is correct if our research method focuses, in the first place, on a social investigation problem (where authors should define the hypothesis, framework, procedure, and objective evaluation methods, including pre/post-test scores to prove the effectiveness of the proposed method). Nevertheless, we've applied a different (technical) method, well-known within NLP scientific communities, which is NOT primarily focusing on social investigation. Let's explain.
As briefly replied in Round1#Concern#5, our proposal overview is illustrated in Fig1 and evaluated using both qualitative and qualitative experiments. The suggested technical proposal involves:
• A state-of-the-art language understanding model (mBERT).
• Some computational linguistics rules.
• A greedy algorithm for the best subset selection problem.
And for evaluation, we explained our experimental setup, including data, protocol, metrics, and baseline models to compare with. The results of the automatic evaluations are reported in section 4.3.
Also, to clarify further, the problem of segmenting unpunctuated text is one of the most complex NLP applications to date, and its quality/precision is highly subjective and often affected by contrasting human perceptions. In our humble view, we believe that human judgments (as we asked three experts about their self-evaluation, see section 4.3) of automatically generated segmentations are vital to establish the method's credibility and guarantee its quality. What we follow here is a common practice in many similar published methods. Below we name a few:
- Fan, X., Liu, Y., Liu, G. and Su, B., 2020, November. A Memory-Based Sentence Split and Rephrase Model with Multi-task Training. In International Conference on Neural Information Processing (pp. 643-654). Springer, Cham.
- Guo, Y., Ge, T. and Wei, F., 2020, April. Fact-aware sentence split and rephrase with permutation invariant training. In Proceedings of the AAAI Conference on Artificial Intelligence (Vol. 34, No. 05, pp. 7855-7862).
- Maddela, M., Alva-Manchego, F. and Xu, W., 2021, June. Controllable text simplification with explicit paraphrasing. Association for Computational Linguistics.
- Wang, Q., Liu, P., Zhu, Z., Yin, H., Zhang, Q. and Zhang, L., 2019. A text abstraction summary model based on BERT word embedding and reinforcement learning. Applied Sciences, 9(21), p.4701. (similar method but without conducting a human evaluation)
Thank you so much again for your understanding.