CSRLoan: Cold Start Loan Recommendation with Semantic-Enhanced Neural Matrix Factorization
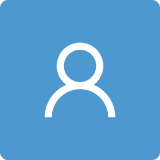
Round 1
Reviewer 1 Report
1. In eqn 3, i is used as time index (1,…K) while in eqn 9, I is used as training sample index. Please use different letters and avoid abusing notations when possible.
2. The pre-training task involves a transformer encoder-decoder architecture to predict the words at decoder output that have been masked in encoder input. Is there any reason why authors went for this specific method?
Why did the authors not use a BERT like MLM task which will have less parameters to train?
3. What is the “activity label” used in loss term L_activity (equation 8)? Is it categorical/binary variable? How is it defined and obtained? What is the output dimension of final softmax layer? Is it the same “a” denoted in section 3.1 line 155 and 156?
4. Did the authors experiment with tuning the contribution of two losses L_mask and L_activity in equation 9 and observe any effect on model performance?
5. Feature encoding: What is difference between a and a’ in section 4.2? As mentioned previously in section 3.1 and line 156, “a” contains loan display name, purchase number etc but what is a’? Is one of them used in L_activity cross entropy loss in eqn 8?
6. What are some examples of value features and category features? What part of a and a’ are value feature and category features?
7. Hyper-parameter values missing — Number of MLP layers, Number of heads, Number of transformer layers, for how many epochs pre-training was done and for how many epochs was the fine-tuning done?
8. Authors should give more information about dataset and experimental methodology. What is the total number of datapoints? How many samples were used for pre-training and fine-tuning with mixture loss? Was a validation set used? What is the size of test set? Are the figures and results showing the numbers on validation set or test set? Did the authors make sure that training and test sets don’t have overlap of users to simulate cold start setting?
9. It is not clear what R and D matrices are in section 4.2. Is Ri,j and Di,j a binary variable?
10. Authors should Show some training data samples in a table for better understanding — e.g. u (applicant user), v (Loan project), s(statement) and the ground truth values for Ri,j and default value Di,j. Activity labels, a, a’ etc.
11. Grammatical and related rectification required. Some examples shown below:
Line 2 there has —> they have
Line 22 has no enough —. has not enough
Punctuation like comma is missing in certain parts
s_n should be included in equation 1
A historical record indicates u apply v with t —> s instead of t in Table 1
Author Response
Dear Reviewer,
We would like to thank you for the valuable comments and the time you spent to help us improve our work “CSRLoan: Cold Start Loan Recommendation with Semantic-Enhanced Neural Matrix Factorization”. We have revised the paper and modified the paper according to your advice. The modifications and responses are listed in the attached file. Moreover, in the revised manuscript, we also highlighted the modified parts in red color.
We look forward to a favorable review.
Sincerely,
Kai Zhuang, Sen Wu, Shuaiqi Liu
Author Response File: Author Response.pdf
Reviewer 2 Report
Moderate english changes required.
References are good, but need to be improved by adding recent publication.
Literature review section need to be modified.
Conclusion section need to be modified.
Author Response
Dear Reviewer,
We would like to thank you for the valuable comments and the time you spent to help us improve our work “CSRLoan: Cold Start Loan Recommendation with Semantic-Enhanced Neural Matrix Factorization”. We have revised the paper and modified the paper according to your advice. The modifications and responses are listed in the attached file. Moreover, in the revised manuscript, we also highlighted the modified parts in red color.
We look forward to a favorable review.
Sincerely,
Kai Zhuang, Sen Wu, Shuaiqi Liu
Author Response File: Author Response.pdf
Reviewer 3 Report
This study addresses an important problem in credit scoring - cold start loan recommendation based on applicant's statement only. This is a challenging prediction task, needing an effective document representation.
Comments:
1) Data used and main findings should be added to the abstract section.
2) In the introduction section, the authors focus on loan recommendation only, however, the credit scoring literature utilizing text information should also be considered in the related literature.
3) The methodology is clear but some settings need justification or reference to support it (e.g., the topology and structure of the neural network, masking parameter).
4) Also, the structure of the neural network should be presented using a scheme. Fig. 1 is too generic in this respect.
5) Why a pre-trained transformer-based model was not used should be explained in more detail. Is the data size sufficient for the pre-training?
6) The data should be described in much more detail. What is the class distribution? What is the min, average and max lenght of statements? How does a typical statement looks like?
7) The results need a detailed discussion. Results in every table/figure should be explained.
8) What was the effect of the number of embedding dimensions?
9) Minor issues: the CSRLoan abbreviation should be explaine, avoid using "and etc.", and so on. Overall, a thorough proofreading is highly recommended.
Author Response
Dear Reviewer,
We would like to thank you for the valuable comments and the time you spent to help us improve our work “CSRLoan: Cold Start Loan Recommendation with Semantic-Enhanced Neural Matrix Factorization”. We have revised the paper and modified the paper according to your advice. The modifications and responses are listed in the attached file. Moreover, in the revised manuscript, we also highlighted the modified parts in red color.
We look forward to a favorable review.
Sincerely,
Kai Zhuang, Sen Wu, Shuaiqi Liu
Author Response File: Author Response.pdf
Reviewer 4 Report
- Authors may extend the Methodology section with an appropriate example (like an analysis of detailed/short statements) to adequately comprehend the three key modules of the proposed approach. In the current form of the manuscript, it is challenging to understand the flow, starting from the generation of embeddings ( focus on the explanation of the minimization of Risk as well)
- The last paragraph regarding the contribution of this paper may be rewritten by avoiding overstatements/ claims (like pioneering, etc.).
3. This manuscript requires extensive language editing.
Author Response
Dear Reviewer,
We would like to thank you for the valuable comments and the time you spent to help us improve our work “CSRLoan: Cold Start Loan Recommendation with Semantic-Enhanced Neural Matrix Factorization”. We have revised the paper and modified the paper according to your advice. The modifications and responses are listed in the attached file. Moreover, in the revised manuscript, we also highlighted the modified parts in red color.
We look forward to a favorable review.
Sincerely,
Kai Zhuang, Sen Wu, Shuaiqi Liu
Author Response File: Author Response.pdf
Round 2
Reviewer 3 Report
The authors considered my previous concerns adequately, I am satisfied with the authors' responses to my comments and that the quality of the paper was substantially improved by presenting the necessary methodological background and additional results.
Author Response
Thanks for your kindly suggestions. We have revised the manuscript and highlight the modification parts in red. Best regards, Kai, Sen and ShuaiqiReviewer 4 Report
This manuscript can be processed further.
Author Response
Thanks for your kindly suggestions. We have revised the manuscript and highlight the modification parts in red. Best regards, Kai, Sen and Shuaiqi