Journal Description
Forecasting
Forecasting
is an international, peer-reviewed, open access journal on all aspects of forecasting published quarterly online by MDPI.
- Open Access— free for readers, with article processing charges (APC) paid by authors or their institutions.
- High Visibility: indexed within Scopus, ESCI (Web of Science), AGRIS, RePEc, and other databases.
- Journal Rank: JCR - Q2 (Multidisciplinary Sciences) / CiteScore - Q1 (Decision Sciences (miscellaneous))
- Rapid Publication: manuscripts are peer-reviewed and a first decision is provided to authors approximately 18.5 days after submission; acceptance to publication is undertaken in 2.3 days (median values for papers published in this journal in the second half of 2024).
- Recognition of Reviewers: reviewers who provide timely, thorough peer-review reports receive vouchers entitling them to a discount on the APC of their next publication in any MDPI journal, in appreciation of the work done.
Impact Factor:
2.3 (2023);
5-Year Impact Factor:
2.3 (2023)
subject
Imprint Information
Open Access
ISSN: 2571-9394
Latest Articles
Day-Ahead Energy Price Forecasting with Machine Learning: Role of Endogenous Predictors
Forecasting 2025, 7(2), 18; https://doi.org/10.3390/forecast7020018 - 9 Apr 2025
Abstract
Accurate Day-Ahead Energy Price (DAEP) forecasting is essential for optimizing energy market operations. This study introduces a machine learning framework to predict the DAEP with a 24 h lead time, leveraging historical data and forecasts available at the prediction time. Hourly DAEP data
[...] Read more.
Accurate Day-Ahead Energy Price (DAEP) forecasting is essential for optimizing energy market operations. This study introduces a machine learning framework to predict the DAEP with a 24 h lead time, leveraging historical data and forecasts available at the prediction time. Hourly DAEP data from the California Independent System Operator (January 2017 to July 2023) were integrated with exogenous and engineered endogenous features. A custom rolling window cross-validation, with 24 h validation blocks sliding daily across 2372 folds, evaluates an Extreme Gradient Boosting (XGBoost) model’s performance under diverse market conditions, achieving a median mean absolute error of 6.26 USD/MWh and root mean squared error of 8.27 USD/MWh, with variability reflecting market volatility. The feature importance analysis using Shapley additive explanations highlighted the dominance of engineered endogenous features in driving the 24 h lead time forecasts under relatively stable market conditions. Forecasting the DAEP at a runtime of 10 AM on the prior day was used to assess model uncertainty. This involved training random forest, support vector regression, XGBoost, and feed forward neural network models, followed by stacking and voting ensembles. The results indicate the need for ensemble forecasting and evaluation beyond a static train–test split to ensure the practical utility of machine learning for DAEP forecasting across varied market dynamics. Finally, operationalizing the forecast model for bidding decisions by forecasting the DAEP and real-time prices at runtime is presented and discussed.
Full article
(This article belongs to the Special Issue New Challenges in Energy and Finance Forecasting in the Era of Big Data)
►
Show Figures
Open AccessCorrection
Correction: Ferreira Lima dos Santos et al. Riding into Danger: Predictive Modeling for ATV-Related Injuries and Seasonal Patterns. Forecasting 2024, 6, 266–278
by
Fernando Ferreira Lima dos Santos, Farzaneh Khorsandi and Guilherme De Moura Araujo
Forecasting 2025, 7(2), 17; https://doi.org/10.3390/forecast7020017 - 7 Apr 2025
Abstract
Addition of an Author [...]
Full article
Open AccessArticle
Volatility Modelling of the Johannesburg Stock Exchange All Share Index Using the Family GARCH Model
by
Israel Maingo, Thakhani Ravele and Caston Sigauke
Forecasting 2025, 7(2), 16; https://doi.org/10.3390/forecast7020016 - 3 Apr 2025
Abstract
In numerous domains of finance and economics, modelling and predicting stock market volatility is essential. Predicting stock market volatility is widely used in the management of portfolios, analysis of risk, and determination of option prices. This study is about volatility modelling of the
[...] Read more.
In numerous domains of finance and economics, modelling and predicting stock market volatility is essential. Predicting stock market volatility is widely used in the management of portfolios, analysis of risk, and determination of option prices. This study is about volatility modelling of the daily Johannesburg Stock Exchange All Share Index (JSE ALSI) stock price data between 1 January 2014 and 29 December 2023. The modelling process incorporated daily log returns derived from the JSE ALSI. The following volatility models were presented for the period: sGARCH(1, 1) and fGARCH(1, 1). The models for volatility were fitted using five unique error distribution assumptions, including Student’s t, its skewed version, the generalized error and skewed generalized error distributions, and the generalized hyperbolic distribution. Based on information criteria such as Akaike, Bayesian, and Hannan–Quinn, the ARMA(0, 0)-fGARCH(1, 1) model with a skewed generalized error distribution emerged as the best fit. The chosen model revealed that the JSE ALSI prices are highly persistent with the leverage effect. JSE ALSI price volatility was notably influenced during the COVID-19 pandemic. The forecast over the next 10 days shows a rise in volatility. A comparative study was then carried out with the JSE Top 40 and the S&P500 indices. Comparison of the FTSE/JSE Top 40, S&P 500, and JSE ALLSI return indices over the COVID-19 pandemic indicated higher initial volatility in the FTSE/JSE Top 40 and S&P 500, with the JSE ALLSI following a similar trend later. The S&P 500 showed long-term reliability and high rolling returns in spite of short-run volatility, the FTSE/JSE Top 40 showed more pre-pandemic risk and volatility but reduced levels of rolling volatility after the pandemic, similar in magnitude for each index with low correlations among them. These results provide important insights for risk managers and investors navigating the South African equity market.
Full article
(This article belongs to the Section Forecasting in Economics and Management)
►▼
Show Figures
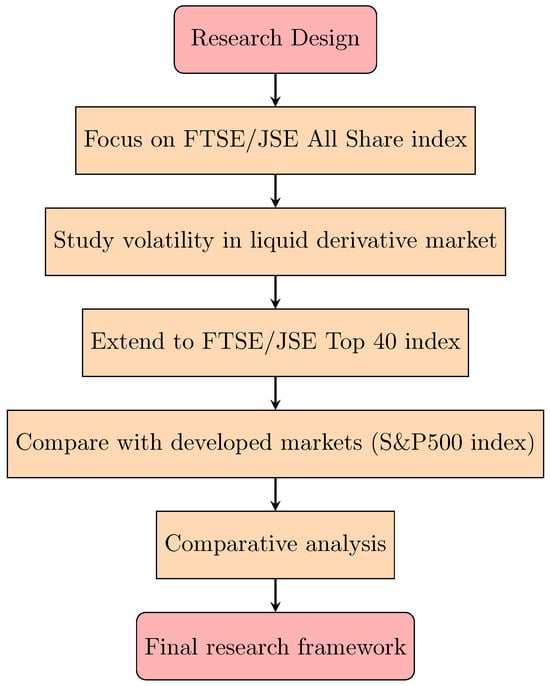
Figure 1
Open AccessArticle
Mode Decomposition Bi-Directional Long Short-Term Memory (BiLSTM) Attention Mechanism and Transformer (AMT) Model for Ozone (O3) Prediction in Johannesburg, South Africa
by
Israel Edem Agbehadji and Ibidun Christiana Obagbuwa
Forecasting 2025, 7(2), 15; https://doi.org/10.3390/forecast7020015 - 2 Apr 2025
Abstract
This paper presents a model that combines mode decomposition approaches with a bi-directional long short-term memory (BiLSTM) attention mechanism and a transformer (AMT) to predict the concentration level of ozone (O3) in Johannesburg, South Africa. Johannesburg is a densely populated city
[...] Read more.
This paper presents a model that combines mode decomposition approaches with a bi-directional long short-term memory (BiLSTM) attention mechanism and a transformer (AMT) to predict the concentration level of ozone (O3) in Johannesburg, South Africa. Johannesburg is a densely populated city and the industrial and economic hub of South Africa. Being the industrial hub, air pollution is a major concern as it affects human health. Using air pollutants and meteorological datasets, a model was proposed that uses a mode decomposition approach to address the nonlinear nature of O3 concentration. This nonlinearity is one of the most challenging issues in air quality prediction, and this study proposed a model to decompose input data and identify the most relevant features and leverage attention mechanisms to produce weighted parameters that can enhance the model’s performance. The model’s performance enhancement approach was aimed at ensuring an effective model that easily adapts to frequently changing pollutant data in air quality prediction. The performance was evaluated statistically with root mean squared error (RMSE), mean absolute error (MAE), and mean square error (MSE). The proposed EEMD-CEEMDAN-BiLSTM-AMT model produced the most optimal result with MSE (4.80 × 10−6), RMSE (0.002), and MAE (0.001). When compared with the other similar models, the proposed model was best in terms of MSE value. Future work seeks to enhance the proposed model to fine-tune its performance on different air pollutant concentrations in South Africa.
Full article
(This article belongs to the Section Environmental Forecasting)
►▼
Show Figures
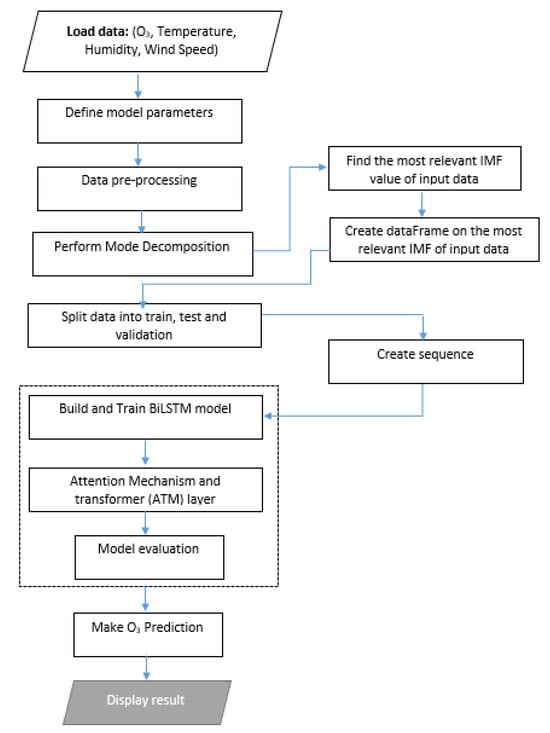
Figure 1
Open AccessArticle
Synthetic Demand Flow Generation Using the Proximity Factor
by
Ekin Yalvac and Michael G. Kay
Forecasting 2025, 7(1), 14; https://doi.org/10.3390/forecast7010014 - 19 Mar 2025
Abstract
One of the biggest challenges in designing a logistics network is predicting the demand flows between all pairs of points in the network. Currently, the gravity model is mainly used for estimating the demand flow between points. However, the gravity model uses historical
[...] Read more.
One of the biggest challenges in designing a logistics network is predicting the demand flows between all pairs of points in the network. Currently, the gravity model is mainly used for estimating the demand flow between points. However, the gravity model uses historical data to estimate values for its multiple parameters and distance between pairs to forecast the demand flow. Distance values close to zero and unprecedented changes in demand flow data create numerical instability for the gravity model’s output. Hence, the proximity factor, a single parameter model that uses the relative ordering of pairs instead of distance, was developed. In this paper, we systematically compare the proximity factor and the gravity model. It is shown that the proximity factor is a robust in terms of reliability and competitive alternative to the gravity model. According to our analysis, the proximity factor model can replace the gravity model in some applications when no historical data are available to adjust the parameters of the latter.
Full article
(This article belongs to the Section Forecasting in Economics and Management)
►▼
Show Figures
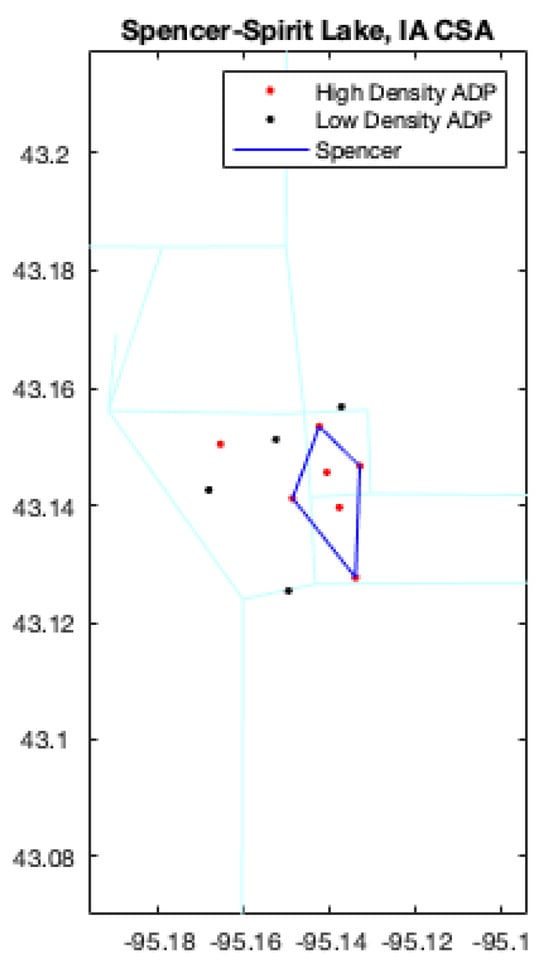
Figure 1
Open AccessArticle
Forecasting Wind Speed Using Climate Variables
by
Rafael Araujo Couto, Paula Medina Maçaira Louro and Fernando Luiz Cyrino Oliveira
Forecasting 2025, 7(1), 13; https://doi.org/10.3390/forecast7010013 - 11 Mar 2025
Abstract
Wind energy in Brazil has been steadily growing, influenced significantly by climate change. To enhance wind energy generation, it is essential to incorporate external climatic variables into wind speed modeling to reduce uncertainties. Periodic Autoregressive Models with Exogenous Variables (PARX), which include the
[...] Read more.
Wind energy in Brazil has been steadily growing, influenced significantly by climate change. To enhance wind energy generation, it is essential to incorporate external climatic variables into wind speed modeling to reduce uncertainties. Periodic Autoregressive Models with Exogenous Variables (PARX), which include the exogenous variable ENSO, are effective for this purpose. This study modeled wind speed series in Rio Grande do Norte, Paraíba, Pernambuco, Alagoas, Sergipe, Rio Grande do Sul, and Santa Catarina, considering the spatial correlation between these states through PARX-Cov modeling. Additionally, the correlation with ENSO indicators was used for out-of-sample prediction of climatic variables, aiding in wind speed scenario simulation. The proposed PARX and PARX-Cov models outperformed the current model used in the Brazilian electric sector for simulating future wind speed series. Specifically, the PARX-Cov model with the Cumulative ONI index is most suitable for Pernambuco, Rio Grande do Sul, and Santa Catarina, while the PARX-Cov with the SOI index is more appropriate for Rio Grande do Norte. For Alagoas and Sergipe, the PARX with the Cumulative ONI index is the best fit, and the PARX with the Cumulative Niño 4 index is most suitable for Paraíba.
Full article
(This article belongs to the Special Issue Advance Techniques for Solar Radiation, Wind Speed and Photovoltaic Forecasting)
►▼
Show Figures
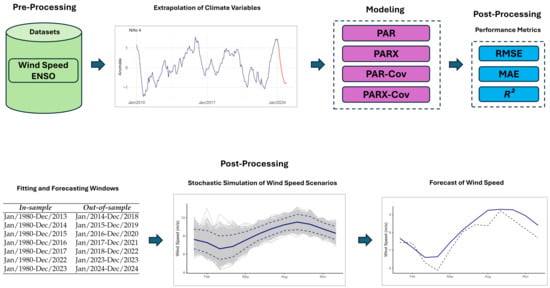
Figure 1
Open AccessArticle
Multifeature-Driven Multistep Wind Speed Forecasting Using NARXR and Modified VMD Approaches
by
Rose Ellen Macabiog and Jennifer Dela Cruz
Forecasting 2025, 7(1), 12; https://doi.org/10.3390/forecast7010012 - 5 Mar 2025
Cited by 1
Abstract
The global demand for clean and sustainable energy has driven the rapid growth of wind power. However, wind farm managers face the challenge of forecasting wind power for efficient power generation and management. Accurate wind speed forecasting (WSF) is vital for predicting wind
[...] Read more.
The global demand for clean and sustainable energy has driven the rapid growth of wind power. However, wind farm managers face the challenge of forecasting wind power for efficient power generation and management. Accurate wind speed forecasting (WSF) is vital for predicting wind power; yet, the variability and intermittency of the wind make forecasting wind speeds difficult. Consequently, WSF remains a challenging area of wind research, driving continuous improvement in the field. This study aimed to enhance the optimization of multifeature-driven short multistep WSF. The primary contributions of this research include the integration of ReliefF feature selection (RFFS), a novel approach to variational mode decomposition for multifeature decomposition (NAMD), and a recursive non-linear autoregressive with exogenous inputs (NARXR) neural network. In particular, RFFS aids in identifying meteorological features that significantly influence wind speed variations, thus ensuring the selection of the most impactful features; NAMD improves the accuracy of neural network training on historical data; and NARXR enhances the overall robustness and stability of the wind speed forecasting results. The experimental results demonstrate that the predictive accuracy of the proposed NAMD–NARXR hybrid model surpasses that of the models used for comparison, as evidenced by the forecasting error and statistical metrics. Integrating the strengths of RFFS, NAMD, and NARXR enhanced the forecasting performance of the proposed NAMD–NARXR model, highlighting its potential suitability for applications requiring multifeature-driven short-term multistep WSF.
Full article
(This article belongs to the Special Issue Advance Techniques for Solar Radiation, Wind Speed and Photovoltaic Forecasting)
►▼
Show Figures
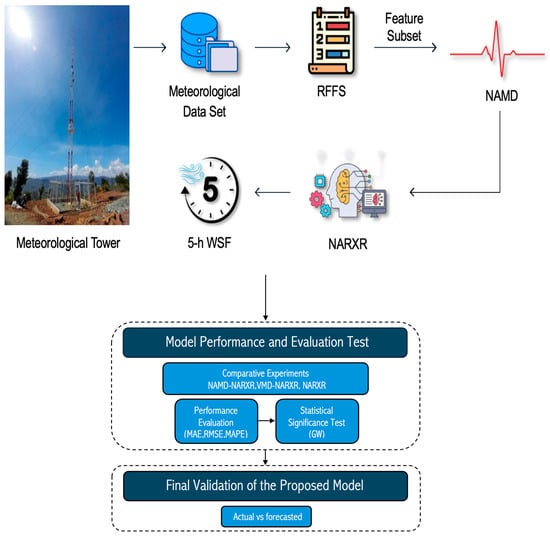
Figure 1
Open AccessArticle
Dynamic Bayesian Network Model for Overhead Power Lines Affected by Hurricanes
by
Kehkashan Fatima and Hussain Shareef
Forecasting 2025, 7(1), 11; https://doi.org/10.3390/forecast7010011 - 5 Mar 2025
Abstract
This paper investigates the dynamics of Hurricane-Induced Failure (HIF) by developing a probabilistic framework using a Dynamic Bayesian Network (DBN) model. The model captures the complex interplay of factors influencing Hurricane Wind Speed Intensity (HWSI) and its impact on asset failures. In the
[...] Read more.
This paper investigates the dynamics of Hurricane-Induced Failure (HIF) by developing a probabilistic framework using a Dynamic Bayesian Network (DBN) model. The model captures the complex interplay of factors influencing Hurricane Wind Speed Intensity (HWSI) and its impact on asset failures. In the proposed DBN model, the pole failure mechanism is represented using Bayesian probabilistic principles, encompassing bending elasticity endurance and the foundational strength of the system poles. To characterize the stochastic properties of HIF, Monte Carlo simulation (MCS) is employed in conjunction with fragility curves (FC) and the scenario reduction (SCENRED) algorithm. The proposed DBN model evaluates the probability of asset failure and compares the results using stochastic Monte Carlo simulation based on the fragility curve scenario reduction algorithm (FC-MCS-SCENRED) model. The results are validated on a standard IEEE 15 bus and IEEE 33 bus radial distribution system as a case study. The DBN results show that they are consistent with the data obtained using the FC-MCS-SCENRED model. The results also reveal that the HWSI plays a critical role in determining HIF rates and the likelihood of asset failures. These findings hold significant implications for the inspection and maintenance scheduling of distribution overhead power lines susceptible to hurricane-induced impacts.
Full article
(This article belongs to the Section Power and Energy Forecasting)
►▼
Show Figures
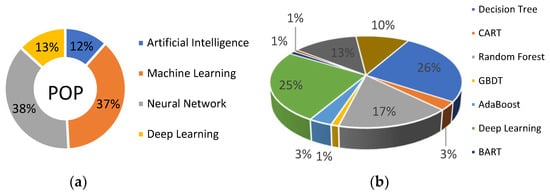
Figure 1
Open AccessArticle
Exchange Rates, Supply Chain Activity/Disruption Effects, and Exports
by
Simiso Msomi and Paul-Francios Muzindutsi
Forecasting 2025, 7(1), 10; https://doi.org/10.3390/forecast7010010 - 28 Feb 2025
Abstract
In the past, South African monetary policy aimed to protect the external value of the domestic currency (Rand); however, these efforts failed. Later, its monetary policy approach changed to allow the foreign exchange rate market to determine the exchange rates. In such a
[...] Read more.
In the past, South African monetary policy aimed to protect the external value of the domestic currency (Rand); however, these efforts failed. Later, its monetary policy approach changed to allow the foreign exchange rate market to determine the exchange rates. In such a change, the South African Reserve Bank (SARB) aimed to stabilize the demand for the Rand in the foreign exchange market by providing information to stabilize market expectations and create favorable market conditions. However, South African policymakers have struggled with currency depreciation since the early 60s, increasing the uncertainty of South African exports. This study aims to examine the effect of currency depreciation on exports using the Threshold Autoregressive (TAR) model. Additionally, this study created and validated the supply chain activity/disruption index to capture the sea trade activity. The sample period for the analysis is 2009 to 2023. The study finds that currency depreciation does not improve trade between South Africa and its trading partners over time. Furthermore, the currency depreciation was found to be asymmetric to the effect of international trade across the different regimes. The supply chain activity index shows that the effect of supply chain activity/disruption on exports is regime-dependent. This implies that the effect on exports is dependent on the economic environment.
Full article
(This article belongs to the Special Issue Forecasting and Foresight in Business and Economics in the Turbulent and Uncertain New Normal)
►▼
Show Figures
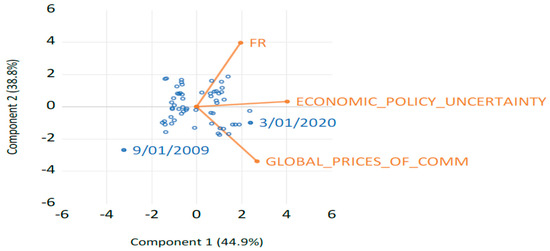
Figure 1
Open AccessArticle
Comparative Analysis of Physics-Guided Bayesian Neural Networks for Uncertainty Quantification in Dynamic Systems
by
Xinyue Xu and Julian Wang
Forecasting 2025, 7(1), 9; https://doi.org/10.3390/forecast7010009 - 21 Feb 2025
Abstract
Uncertainty quantification (UQ) is critical for modeling complex dynamic systems, ensuring robustness and interpretability. This study extends Physics-Guided Bayesian Neural Networks (PG-BNNs) to enhance model robustness by integrating physical laws into Bayesian frameworks. Unlike Artificial Neural Networks (ANNs), which provide deterministic predictions, and
[...] Read more.
Uncertainty quantification (UQ) is critical for modeling complex dynamic systems, ensuring robustness and interpretability. This study extends Physics-Guided Bayesian Neural Networks (PG-BNNs) to enhance model robustness by integrating physical laws into Bayesian frameworks. Unlike Artificial Neural Networks (ANNs), which provide deterministic predictions, and Bayesian Neural Networks (BNNs), which handle uncertainty probabilistically but struggle with generalization under sparse and noisy data, PG-BNNs incorporate the laws of physics, such as governing equations and boundary conditions, to enforce physical consistency. This physics-guided approach improves generalization across different noise levels while reducing data dependency. The effectiveness of PG-BNNs is validated through a one-degree-of-freedom vibration system with multiple noise levels, serving as a representative case study to compare the performance of Monte Carlo (MC) dropout ANNs, BNNs, and PG-BNNs across interpolation and extrapolation domains. Model accuracy is assessed using Mean Squared Error (MSE), Mean Absolute Percentage Error (MAE), and Coefficient of Variation of Root Mean Square Error (CVRMSE), while UQ is evaluated through 95% Credible Intervals (CIs), Mean Prediction Interval Width (MPIW), the Quality of Confidence Intervals (QCI), and Coverage Width-based Criterion (CWC). Results demonstrate that PG-BNNs can achieve high accuracy and good adherence to physical laws simultaneously, compared to MC dropout ANNs and BNNs, which confirms the potential of PG-BNNs in engineering applications related to dynamic systems.
Full article
(This article belongs to the Special Issue Feature Papers of Forecasting 2024)
►▼
Show Figures
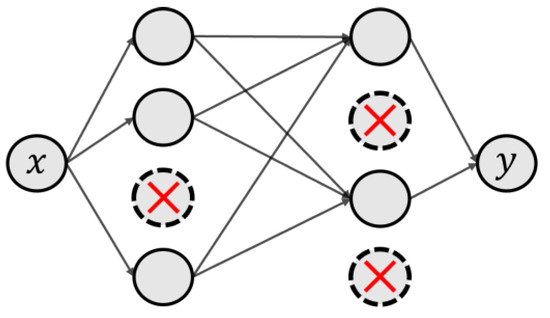
Figure 1
Open AccessArticle
White Noise and Its Misapplications: Impacts on Time Series Model Adequacy and Forecasting
by
Hossein Hassani, Leila Marvian Mashhad, Manuela Royer-Carenzi, Mohammad Reza Yeganegi and Nadejda Komendantova
Forecasting 2025, 7(1), 8; https://doi.org/10.3390/forecast7010008 - 5 Feb 2025
Cited by 1
Abstract
This paper contributes significantly to time series analysis by discussing the empirical properties of white noise and their implications for model selection. This paper illustrates the ways in which the standard assumptions about white noise typically fail in practice, with a special emphasis
[...] Read more.
This paper contributes significantly to time series analysis by discussing the empirical properties of white noise and their implications for model selection. This paper illustrates the ways in which the standard assumptions about white noise typically fail in practice, with a special emphasis on striking differences in sample ACF and PACF. Such findings prove particularly important when assessing model adequacy and discerning between residuals of different models, especially ARMA processes. This study addresses issues involving testing procedures, for instance, the Ljung–Box test, to select the correct time series model determined in the review. With the improvement in understanding the features of white noise, this work enhances the accuracy of modeling diagnostics toward real forecasting practice, which gives it applied value in time series analysis and signal processing.
Full article
(This article belongs to the Special Issue Forecasting and Foresight in Business and Economics in the Turbulent and Uncertain New Normal)
►▼
Show Figures
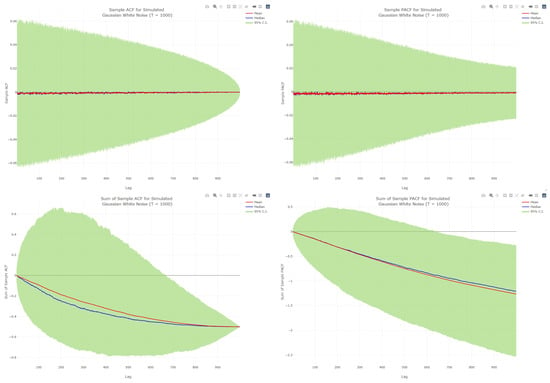
Figure 1
Open AccessArticle
Evaluating the Potential of Copulas for Modeling Correlated Scenarios for Hydro, Wind, and Solar Energy
by
Anderson M. Iung, Fernando L. Cyrino Oliveira, Andre L. M. Marcato and Guilherme A. A. Pereira
Forecasting 2025, 7(1), 7; https://doi.org/10.3390/forecast7010007 - 30 Jan 2025
Abstract
The increasing global adoption of variable renewable energy (VRE) sources has transformed the use of forecasting, scenario planning, and other techniques for managing their inherent generation uncertainty and interdependencies. What were once desirable enhancements are now fundamental requirements. This is more prominent in
[...] Read more.
The increasing global adoption of variable renewable energy (VRE) sources has transformed the use of forecasting, scenario planning, and other techniques for managing their inherent generation uncertainty and interdependencies. What were once desirable enhancements are now fundamental requirements. This is more prominent in Brazil, given the large hydro capacity that has been installed. Given the need to understand the interdependencies within variable renewable energy systems, copula-based techniques are receiving increasing consideration. The objective is to explore and model the correlation and complementarity, based on the copula approach, evaluating the potential of this methodology considering a case test composed of hydro, wind, and solar assets. The proposed framework simulated joint scenarios for monthly natural energy (streamflows transformed into energy), wind speed and solar radiation, applied to a small case test, considering historical data from the Brazilian energy system. The results demonstrate that simulated scenarios are validated by their ability to replicate key statistical attributes of the historical record, as well as the interplay and complementarity among hydrology, wind speed, and solar radiation.
Full article
(This article belongs to the Special Issue Feature Papers of Forecasting 2024)
►▼
Show Figures
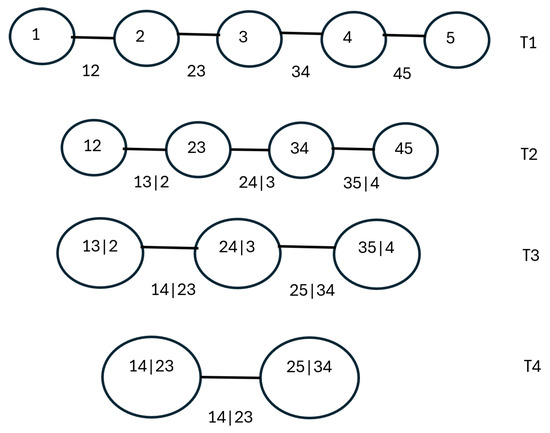
Figure 1
Open AccessArticle
Assessment of Deep Neural Network Models for Direct and Recursive Multi-Step Prediction of PM10 in Southern Spain
by
Javier Gómez-Gómez, Eduardo Gutiérrez de Ravé and Francisco J. Jiménez-Hornero
Forecasting 2025, 7(1), 6; https://doi.org/10.3390/forecast7010006 - 26 Jan 2025
Abstract
Western Europe has been strongly affected in the last decades by Saharan dust incursions, causing a high PM10 concentration and red rain. In this study, dust events and the performance of seven neural network prediction models, including convolutional neural networks (CNN) and recurrent
[...] Read more.
Western Europe has been strongly affected in the last decades by Saharan dust incursions, causing a high PM10 concentration and red rain. In this study, dust events and the performance of seven neural network prediction models, including convolutional neural networks (CNN) and recurrent neural networks (RNN), have been analyzed in a PM10 concentration series from a monitoring station in Córdoba, southern Spain. The models were also assessed here for recursive multi-step prediction over different forecast periods in three different situations: background concentration, a strong dust event, and an extreme dust event. A very important increase in the number of dust events has been identified in the last few years. Results show that CNN models outperform the other models in terms of accuracy for direct 24 h prediction (RMSE values between 10.00 and 10.20 μg/m3), whereas the recursive prediction is only suitable for background concentration in the short term (for 2–5-day forecasts). The assessment and improvement of prediction models might help the development of early-warning systems for these events. From the authors’ perspective, the evaluation of trained models beyond the direct multi-step predictions allowed to fill a gap in this research field, which few articles have explored in depth.
Full article
(This article belongs to the Section Environmental Forecasting)
►▼
Show Figures
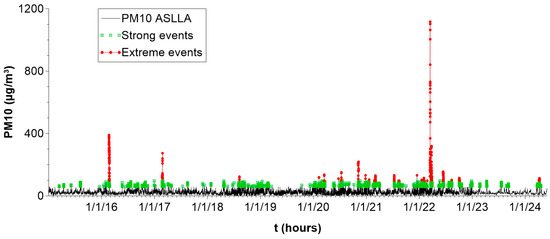
Figure 1
Open AccessArticle
Methodology Based on BERT (Bidirectional Encoder Representations from Transformers) to Improve Solar Irradiance Prediction of Deep Learning Models Trained with Time Series of Spatiotemporal Meteorological Information
by
Llinet Benavides-Cesar, Miguel-Ángel Manso-Callejo and Calimanut-Ionut Cira
Forecasting 2025, 7(1), 5; https://doi.org/10.3390/forecast7010005 - 17 Jan 2025
Abstract
►▼
Show Figures
Accurate solar resource forecasting is important because of the inherent variability associated with solar energy and its significant impact on the cost for energy producers. The traditional method applied in solar irradiance forecasting involves two main phases, related to (1) data selection and
[...] Read more.
Accurate solar resource forecasting is important because of the inherent variability associated with solar energy and its significant impact on the cost for energy producers. The traditional method applied in solar irradiance forecasting involves two main phases, related to (1) data selection and (2) model selection, training, and evaluation. In this study, we propose a novel end-to-end methodology for solar irradiance forecasting that starts with the search for the data and all of the preprocessing operations involved in obtaining a quality dataset, continuing by imputing missing data with the BERT (Bidirectional Encoder Representations from Transformers) model, and ending with obtaining and evaluating the predicted values. This novel methodology is based on three phases; namely, Phase_1, related to the acquisition and preparation of the data, Phase_2, related to the proposed imputation with a BERT model, and Phase_3, related to the training and prediction with new models based on deep learning. These phases of the proposed methodology can be applied in a disjointed manner, and were used on two public datasets accessible to the scientific community. Each of the proposed phases proved to be valuable for the workflow, and the application of the novel method delivered increases in performance of up to 3 percentage points (3%) when compared to the traditional approach.
Full article
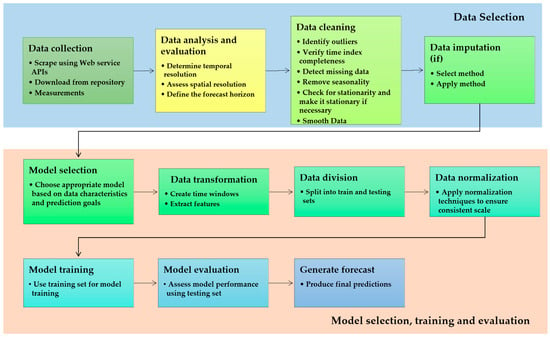
Figure 1
Open AccessArticle
Testing for Bias in Forecasts for Independent Multinomial Outcomes
by
Philip Hans Franses and Richard Paap
Forecasting 2025, 7(1), 4; https://doi.org/10.3390/forecast7010004 - 13 Jan 2025
Abstract
This paper deals with a test on forecast bias in predicting independent multinomial outcomes where the predictions are probabilities. The new Likelihood Ratio (and Wald) test extends the familiar Mincer Zarnowitz regression to a multinomial logit model instead of a linear regression. The
[...] Read more.
This paper deals with a test on forecast bias in predicting independent multinomial outcomes where the predictions are probabilities. The new Likelihood Ratio (and Wald) test extends the familiar Mincer Zarnowitz regression to a multinomial logit model instead of a linear regression. The test is evaluated using various simulation experiments, which indicate that the size and power properties are good, even for small sample sizes, in the sense that the size is close to the used 5% level, and the power quickly reaches 1. We implement the test in an empirical setting on brand choice by individual households.
Full article
(This article belongs to the Special Issue Feature Papers of Forecasting 2024)
►▼
Show Figures
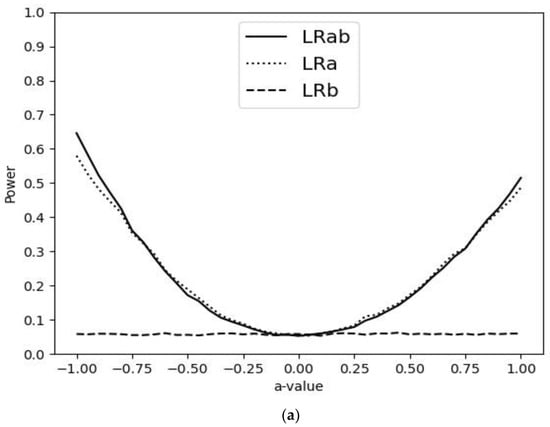
Figure 1
Open AccessArticle
The MECOVMA Framework: Implementing Machine Learning Under Macroeconomic Volatility for Marketing Predictions
by
Manuel Muth
Forecasting 2025, 7(1), 3; https://doi.org/10.3390/forecast7010003 - 7 Jan 2025
Abstract
The methodological framework introduced in this paper, MECOVMA, is a novel framework that guides the application of Machine Learning specifically for marketing predictions within volatile macroeconomic environments. MECOVMA has been developed in response to the identified gaps displayed by existing frameworks—when it comes
[...] Read more.
The methodological framework introduced in this paper, MECOVMA, is a novel framework that guides the application of Machine Learning specifically for marketing predictions within volatile macroeconomic environments. MECOVMA has been developed in response to the identified gaps displayed by existing frameworks—when it comes to consolidation, relevance, interdisciplinarity, and individuality—and in light of the polycrises occurring in the current decade. The methodology to develop MECOVMA comprises three phases: firstly, synthesizing existing frameworks based on their thematic relevance to select MECOVMA’s process steps; secondly, integrating the evidence provided by a systematic literature review to design the content of these process steps; and thirdly, using an expert evaluation, structured through a qualitative content analysis, to validate MECOVMA’s applicability. This leads to the final framework with four overarching PMECOVMA process steps, guiding the Machine Learning application process in this context with specific tasks. These include, for example, the processing of multidimensional data inputs, complexity reduction in a dynamic environment, and training methods adapted to particular macro-conditions. In addition, features are provided on how Machine Learning can be put into marketing practice, incorporating both narrower statistical- and broader business-oriented evaluations, and iterative feedback loops to mitigate limitations.
Full article
(This article belongs to the Special Issue Forecasting and Foresight in Business and Economics in the Turbulent and Uncertain New Normal)
►▼
Show Figures
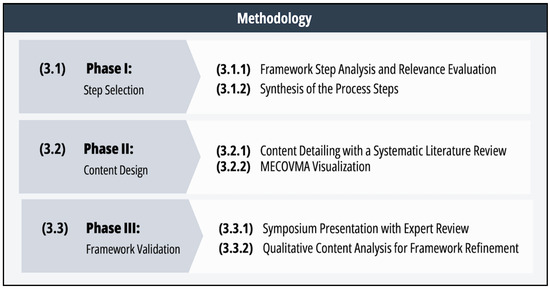
Figure 1
Open AccessArticle
Temporal Attention-Enhanced Stacking Networks: Revolutionizing Multi-Step Bitcoin Forecasting
by
Phumudzo Lloyd Seabe, Edson Pindza, Claude Rodrigue Bambe Moutsinga and Maggie Aphane
Forecasting 2025, 7(1), 2; https://doi.org/10.3390/forecast7010002 - 30 Dec 2024
Abstract
►▼
Show Figures
This study presents a novel methodology for multi-step Bitcoin (BTC) price prediction by combining advanced stacking-based architectures with temporal attention mechanisms. The proposed Temporal Attention-Enhanced Stacking Network (TAESN) integrates the complementary strengths of diverse machine learning algorithms while emphasizing critical temporal features, leading
[...] Read more.
This study presents a novel methodology for multi-step Bitcoin (BTC) price prediction by combining advanced stacking-based architectures with temporal attention mechanisms. The proposed Temporal Attention-Enhanced Stacking Network (TAESN) integrates the complementary strengths of diverse machine learning algorithms while emphasizing critical temporal features, leading to substantial improvements in forecasting accuracy over traditional methods. Comprehensive experimentation and robust evaluation validate the superior performance of TAESN across various BTC prediction horizons. Additionally, the model not only demonstrates enhanced predictive accuracy but also offers interpretable insights into the temporal dynamics underlying cryptocurrency markets, contributing to both practical forecasting applications and theoretical understanding of market behavior.
Full article
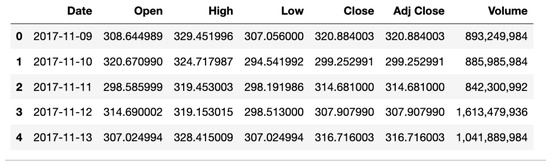
Figure 1
Open AccessArticle
Comparative Analysis of Supervised Learning Techniques for Forecasting PV Current in South Africa
by
Ely Ondo Ekogha and Pius A. Owolawi
Forecasting 2025, 7(1), 1; https://doi.org/10.3390/forecast7010001 - 26 Dec 2024
Abstract
The fluctuations in solar irradiance and temperature throughout the year require an accurate methodology for forecasting the generated current of a PV system based on its specifications. The optimal technique must effectively manage rapid weather fluctuations while maintaining high accuracy in forecasting the
[...] Read more.
The fluctuations in solar irradiance and temperature throughout the year require an accurate methodology for forecasting the generated current of a PV system based on its specifications. The optimal technique must effectively manage rapid weather fluctuations while maintaining high accuracy in forecasting the performance of a PV panel. This work presents a comparative examination of supervised learning algorithms optimized with particle swarm optimization for estimating photovoltaic output current. The empirical formula’s measured currents are compared with outputs from various neural networks techniques, including feedforward neural networks (FFNNs), the general regression network known as GRNN, cascade forward neural networks also known as CFNNs, and adaptive fuzzy inference systems known as ANFISs, all optimized for enhanced accuracy using the particle swarm optimization (PSO) method. The ground data utilized for these models comprises hourly irradiations and temperatures from 2023, sourced from several places in South Africa. The accuracy levels indicated by statistical error margins from the root mean square error (RMSE), mean bias error (MBE), and mean absolute percentage error (MAPE) imply a universal enhancement in the algorithms’ precision upon optimization.
Full article
(This article belongs to the Section Power and Energy Forecasting)
►▼
Show Figures
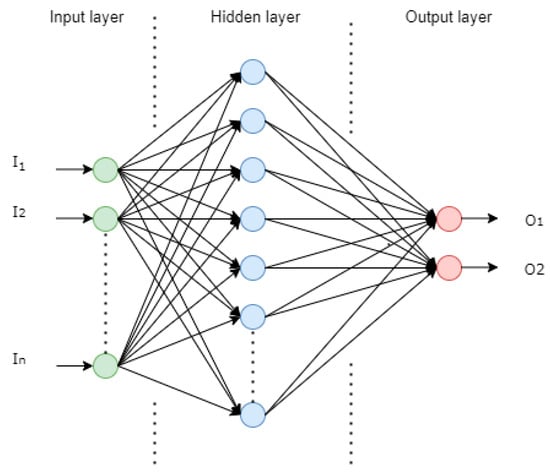
Figure 1
Open AccessArticle
Is Football Unpredictable? Predicting Matches Using Neural Networks
by
Luiz E. Luiz, Gabriel Fialho and João P. Teixeira
Forecasting 2024, 6(4), 1152-1168; https://doi.org/10.3390/forecast6040057 - 12 Dec 2024
Abstract
The growing sports betting market works on the premise that sports are unpredictable, making it more likely to be wrong than right, as the user has to choose between win, draw, or lose. So could football, the world’s most popular sport, be predictable?
[...] Read more.
The growing sports betting market works on the premise that sports are unpredictable, making it more likely to be wrong than right, as the user has to choose between win, draw, or lose. So could football, the world’s most popular sport, be predictable? This article studies this question using deep neural networks to predict the outcome of football matches using publicly available data. Data from 24,760 matches from 13 leagues over 2 to 10 years were used as input for the neural network and to generate a state-of-the-art validated feature, the pi-rating, and the parameters proposed in this work, such as relative attack, defence, and mid power. The data were pre-processed to improve the network’s interpretation and deal with missing or inconsistent data. With the validated pi-rating, data organisation methods were evaluated to find the most fitting option for this prediction system. The final network has four layers with 100, 80, 5, and 3 neurons, respectively, applying the dropout technique to reduce overfitting errors. The results showed that the most influential features are the proposed relative defending, playmaking, and midfield power, and the home team goal expectancy features, surpassing the pi-rating. Finally, the proposed model obtained an accuracy of 52.8% in 2589 matches, reaching 80.3% in specific situations. These results prove that football can be predictable and that some leagues are more predictable than others.
Full article
(This article belongs to the Special Issue Feature Papers of Forecasting 2024)
►▼
Show Figures

Figure 1
Open AccessArticle
Granger Causality-Based Forecasting Model for Rainfall at Ratnapura Area, Sri Lanka: A Deep Learning Approach
by
Shanthi Saubhagya, Chandima Tilakaratne, Pemantha Lakraj and Musa Mammadov
Forecasting 2024, 6(4), 1124-1151; https://doi.org/10.3390/forecast6040056 - 29 Nov 2024
Abstract
Rainfall forecasting, especially extreme rainfall forecasting, is one of crucial tasks in weather forecasting since it has direct impact on accompanying devastating events such as flash floods and fast-moving landslides. However, obtaining rainfall forecasts with high accuracy, especially for extreme rainfall occurrences, is
[...] Read more.
Rainfall forecasting, especially extreme rainfall forecasting, is one of crucial tasks in weather forecasting since it has direct impact on accompanying devastating events such as flash floods and fast-moving landslides. However, obtaining rainfall forecasts with high accuracy, especially for extreme rainfall occurrences, is a challenging task. This study focuses on developing a forecasting model which is capable of forecasting rainfall, including extreme rainfall values. The rainfall forecasting was achieved through sequence learning capability of the Long Short-Term Memory (LSTM) method. The identification of the optimal set of features for the LSTM model was conducted using Random Forest and Granger Causality tests. Then, that best set of features was fed into Stacked LSTM, Bidirectional LSTM, and Encoder-Decoder LSTM models to obtain three days-ahead forecasts of rainfall with the input of the past fourteen days-values of selected features. Out of the three models, the best model was taken through post hoc residual analysis and extra validation approaches. This entire approach was illustrated utilizing rainfall and weather-related measurements obtained from the gauging station located in the city of Ratnapura, Sri Lanka. Originally, twenty-three features were collected including relative humidity, ssunshine hours, and mean sea level pressure. The performances of the three models were compared using . The Bidirectional LSTM model outperformed the other methods (RMSE < 5 mm and MAE < 3 mm) and this model has the capability to forecast extreme rainfall values with high accuracy.
Full article
(This article belongs to the Section Weather and Forecasting)
►▼
Show Figures
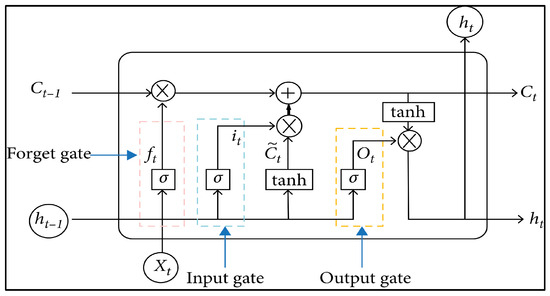
Figure 1
Highly Accessed Articles
Latest Books
E-Mail Alert
News
Topics
Topic in
Electricity, Energies, Forecasting, Processes, Smart Cities, Sustainability
Intelligent, Flexible, and Effective Operation of Smart Grids with Novel Energy Technologies and Equipment
Topic Editors: Pengfei Zhao, Sheng Chen, Yunqi Wang, Liwei Ju, Zhengmao Li, Minglei BaoDeadline: 31 July 2025
Topic in
Economies, Energies, Environments, Forecasting, Laws
Energy Policy, Regulation and Sustainable Development—2nd Edition
Topic Editors: Grzegorz Mentel, Mirela Panait, Xin ZhaoDeadline: 31 December 2025
Topic in
Applied Sciences, Energies, Forecasting, Solar, Wind
Solar and Wind Power and Energy Forecasting, 2nd Edition
Topic Editors: Emanuele Ogliari, Alessandro Niccolai, Sonia LevaDeadline: 31 July 2026

Conferences
Special Issues
Special Issue in
Forecasting
Renewable Energy Forecasting: Innovations and Breakthroughs
Guest Editors: Grzegorz Mentel, Xin ZhaoDeadline: 30 December 2025
Special Issue in
Forecasting
Feature Papers of Forecasting 2025
Guest Editor: Sonia LevaDeadline: 31 December 2025
Topical Collections
Topical Collection in
Forecasting
Supply Chain Management Forecasting
Collection Editors: Gokhan Egilmez, Juan Ramón Trapero Arenas
Topical Collection in
Forecasting
Near-Term Ecological Forecasting
Collection Editor: Michael Dietze