Journal Description
Analytics
Analytics
is an international, peer-reviewed, open access journal on methodologies, technologies, and applications of analytics, published quarterly online by MDPI.
- Open Access— free for readers, with article processing charges (APC) paid by authors or their institutions.
- High Visibility: indexed within Scopus and other databases.
- Rapid Publication: manuscripts are peer-reviewed and a first decision is provided to authors approximately 27.4 days after submission; acceptance to publication is undertaken in 7.7 days (median values for papers published in this journal in the first half of 2025).
- Recognition of Reviewers: APC discount vouchers, optional signed peer review, and reviewer names published annually in the journal.
- Analytics is a companion journal of Mathematics.
Latest Articles
Domestication of Source Text in Literary Translation Prevails over Foreignization
Analytics 2025, 4(3), 17; https://doi.org/10.3390/analytics4030017 - 20 Jun 2025
Abstract
►
Show Figures
Domestication is a translation theory in which the source text (to be translated) is matched to the foreign reader by erasing its original linguistic and cultural difference. This match aims at making the target text (translated text) more fluent. On the contrary, foreignization
[...] Read more.
Domestication is a translation theory in which the source text (to be translated) is matched to the foreign reader by erasing its original linguistic and cultural difference. This match aims at making the target text (translated text) more fluent. On the contrary, foreignization is a translation theory in which the foreign reader is matched to the source text. This paper mathematically explores the degree of domestication/foreignization in current translation practice of texts written in alphabetical languages. A geometrical representation of texts, based on linear combinations of deep–language parameters, allows us (a) to calculate a domestication index which measures how much domestication is applied to the source text and (b) to distinguish language families. An expansion index measures the relative spread around mean values. This paper reports statistics and results on translations of (a) Greek New Testament books in Latin and in 35 modern languages, belonging to diverse language families; and (b) English novels in Western languages. English and French, although attributed to different language families, mathematically almost coincide. The requirement of making the target text more fluent makes domestication, with varying degrees, universally adopted, so that a blind comparison of the same linguistic parameters of a text and its translation hardly indicates that they refer to each other.
Full article
Open AccessArticle
The Classical Model of Type-Token Systems Compared with Items from the Standardized Project Gutenberg Corpus
by
Martin Tunnicliffe and Gordon Hunter
Analytics 2025, 4(2), 16; https://doi.org/10.3390/analytics4020016 - 5 Jun 2025
Abstract
►▼
Show Figures
We compare the “classical” equations of type-token systems, namely Zipf’s laws, Heaps’ law and the relationships between their indices, with data selected from the Standardized Project Gutenberg Corpus (SPGC). Selected items all exceed 100,000 word-tokens and are trimmed to 100,000 word-tokens each. With
[...] Read more.
We compare the “classical” equations of type-token systems, namely Zipf’s laws, Heaps’ law and the relationships between their indices, with data selected from the Standardized Project Gutenberg Corpus (SPGC). Selected items all exceed 100,000 word-tokens and are trimmed to 100,000 word-tokens each. With the most egregious anomalies removed, a dataset of 8432 items is examined in terms of the relationships between the Zipf and Heaps’ indices computed using the Maximum Likelihood algorithm. Zipf’s second (size) law indices suggest that the types vs. frequency distribution is log–log convex, with the high and low frequency indices showing weak but significant negative correlation. Under certain circumstances, the classical equations work tolerably well, though the level of agreement depends heavily on the type of literature and the language (Finnish being notably anomalous). The frequency vs. rank characteristics exhibit log–log linearity in the “middle range” (ranks 100–1000), as characterised by the Kolmogorov–Smirnov significance. For most items, the Heaps’ index correlates strongly with the low frequency Zipf index in a manner consistent with classical theory, while the high frequency indices are largely uncorrelated. This is consistent with a simple simulation.
Full article
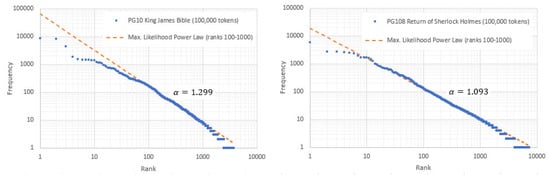
Figure 1
Open AccessArticle
Multiplicity Adjustments for Differences in Proportion Parameters in Multiple-Sample Misclassified Binary Data
by
Dewi Rahardja
Analytics 2025, 4(2), 15; https://doi.org/10.3390/analytics4020015 - 28 May 2025
Abstract
►▼
Show Figures
Generally, following an omnibus (overall equality) test, multiple pairwise comparison (MPC) tests are typically conducted as the second step in a sequential testing procedure to identify which specific pairs (e.g., proportions) exhibit significant differences. In this manuscript, we develop maximum likelihood estimation (MLE)
[...] Read more.
Generally, following an omnibus (overall equality) test, multiple pairwise comparison (MPC) tests are typically conducted as the second step in a sequential testing procedure to identify which specific pairs (e.g., proportions) exhibit significant differences. In this manuscript, we develop maximum likelihood estimation (MLE) methods to construct three different types of confidence intervals (CIs) for multiple pairwise differences in proportions, specifically in contexts where both types of misclassifications (i.e., over-reporting and under-reporting) exist in multiple-sample binomial data. Our closed-form algorithm is straightforward to implement. Consequently, when dealing with multiple sample proportions, we can readily apply MPC adjustment procedures—such as Bonferroni, Šidák, and Dunn—to address the issue of multiplicity. This manuscript advances the existing literature by extending from scenarios with only one type of misclassification to those involving both. Furthermore, we demonstrate our methods using a real-world data example.
Full article
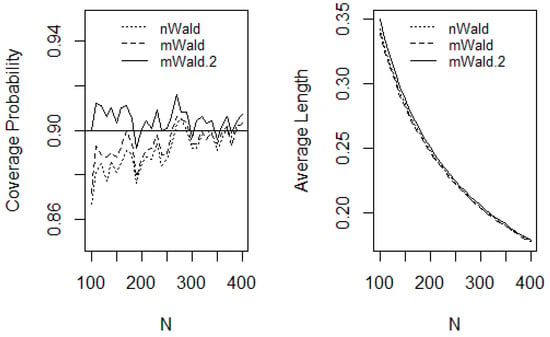
Figure 1
Open AccessReview
Analytical Modeling of Ancillary Items
by
John Wilson
Analytics 2025, 4(2), 14; https://doi.org/10.3390/analytics4020014 - 19 May 2025
Abstract
►▼
Show Figures
Airlines profitability increasingly depends on the sale of ancillary items such as seat selection, baggage fees, etc. The modeling of ancillary items is becoming more important in the analytics literature. Much of the modeling is stylized and not immediately applicable. This paper contains
[...] Read more.
Airlines profitability increasingly depends on the sale of ancillary items such as seat selection, baggage fees, etc. The modeling of ancillary items is becoming more important in the analytics literature. Much of the modeling is stylized and not immediately applicable. This paper contains a review of the approaches and modeling assumptions made in the literature. The focus is on the assumptions made so that models may be evaluated for how effective they are for applications and to highlight gaps in the literature.
Full article
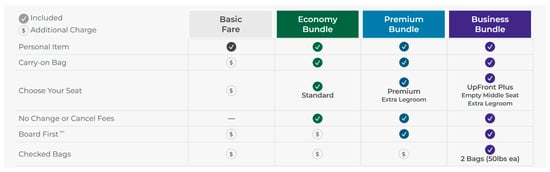
Figure 1
Open AccessSystematic Review
Artificial Intelligence Applied to the Analysis of Biblical Scriptures: A Systematic Review
by
Bruno Cesar Lima, Nizam Omar, Israel Avansi and Leandro Nunes de Castro
Analytics 2025, 4(2), 13; https://doi.org/10.3390/analytics4020013 - 11 Apr 2025
Abstract
►▼
Show Figures
The Holy Bible is the most read book in the world, originally written in Aramaic, Hebrew, and Greek over a time span in the order of centuries by many people, and formed by a combination of various literary styles, such as stories, prophecies,
[...] Read more.
The Holy Bible is the most read book in the world, originally written in Aramaic, Hebrew, and Greek over a time span in the order of centuries by many people, and formed by a combination of various literary styles, such as stories, prophecies, poetry, instructions, and others. As such, the Bible is a complex text to be analyzed by humans and machines. This paper provides a systematic survey of the application of Artificial Intelligence (AI) and some of its subareas to the analysis of the Biblical scriptures. Emphasis is given to what types of tasks are being solved, what are the main AI algorithms used, and their limitations. The findings deliver a general perspective on how this field is being developed, along with its limitations and gaps. This research follows a procedure based on three steps: planning (defining the review protocol), conducting (performing the survey), and reporting (formatting the report). The results obtained show there are seven main tasks solved by AI in the Bible analysis: machine translation, authorship identification, part of speech tagging (PoS tagging), semantic annotation, clustering, categorization, and Biblical interpretation. Also, the classes of AI techniques with better performance when applied to Biblical text research are machine learning, neural networks, and deep learning. The main challenges in the field involve the nature and style of the language used in the Bible, among others.
Full article
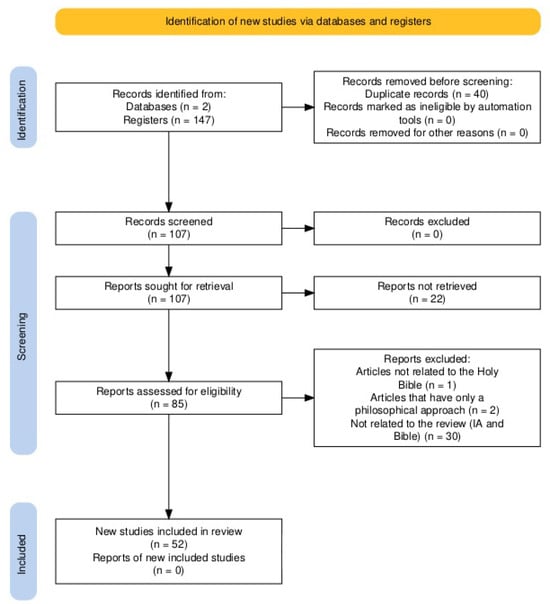
Figure 1
Open AccessArticle
Traffic Prediction with Data Fusion and Machine Learning
by
Juntao Qiu and Yaping Zhao
Analytics 2025, 4(2), 12; https://doi.org/10.3390/analytics4020012 - 9 Apr 2025
Cited by 2
Abstract
►▼
Show Figures
Traffic prediction, as a core task to alleviate urban congestion and optimize the transport system, has limitations in the integration of multimodal data, making it difficult to comprehensively capture the complex spatio-temporal characteristics of the transport system. Although some studies have attempted to
[...] Read more.
Traffic prediction, as a core task to alleviate urban congestion and optimize the transport system, has limitations in the integration of multimodal data, making it difficult to comprehensively capture the complex spatio-temporal characteristics of the transport system. Although some studies have attempted to introduce multimodal data, they mostly rely on resource-intensive deep neural network architectures, which have difficultly meeting the demands of practical applications. To this end, we propose a traffic prediction framework based on simple machine learning techniques that effectively integrates property features, amenity features, and emotion features (PAE features). Validated with large-scale real datasets, the method demonstrates excellent prediction performance while significantly reducing computational complexity and deployment costs. This study demonstrates the great potential of simple machine learning techniques in multimodal data fusion, provides an efficient and practical solution for traffic prediction, and offers an effective alternative to resource-intensive deep learning methods, opening up new paths for building scalable traffic prediction systems.
Full article
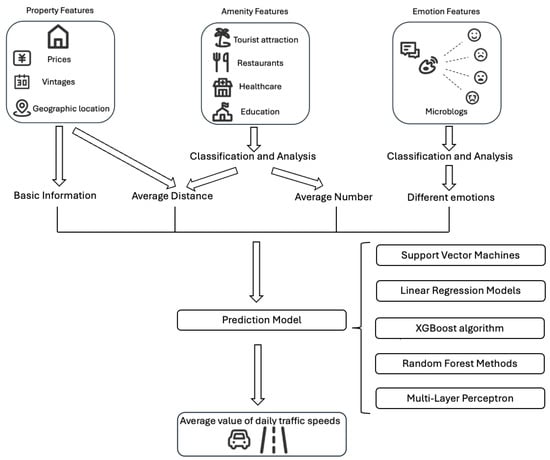
Figure 1
Open AccessArticle
Copula-Based Bayesian Model for Detecting Differential Gene Expression
by
Prasansha Liyanaarachchi and N. Rao Chaganty
Analytics 2025, 4(2), 11; https://doi.org/10.3390/analytics4020011 - 3 Apr 2025
Abstract
►▼
Show Figures
Deoxyribonucleic acid, more commonly known as DNA, is a fundamental genetic material in all living organisms, containing thousands of genes, but only a subset exhibit differential expression and play a crucial role in diseases. Microarray technology has revolutionized the study of gene expression,
[...] Read more.
Deoxyribonucleic acid, more commonly known as DNA, is a fundamental genetic material in all living organisms, containing thousands of genes, but only a subset exhibit differential expression and play a crucial role in diseases. Microarray technology has revolutionized the study of gene expression, with two primary types available for expression analysis: spotted cDNA arrays and oligonucleotide arrays. This research focuses on the statistical analysis of data from spotted cDNA microarrays. Numerous models have been developed to identify differentially expressed genes based on the red and green fluorescence intensities measured using these arrays. We propose a novel approach using a Gaussian copula model to characterize the joint distribution of red and green intensities, effectively capturing their dependence structure. Given the right-skewed nature of the intensity distributions, we model the marginal distributions using gamma distributions. Differentially expressed genes are identified using the Bayes estimate under our proposed copula framework. To evaluate the performance of our model, we conduct simulation studies to assess parameter estimation accuracy. Our results demonstrate that the proposed approach outperforms existing methods reported in the literature. Finally, we apply our model to Escherichia coli microarray data, illustrating its practical utility in gene expression analysis.
Full article
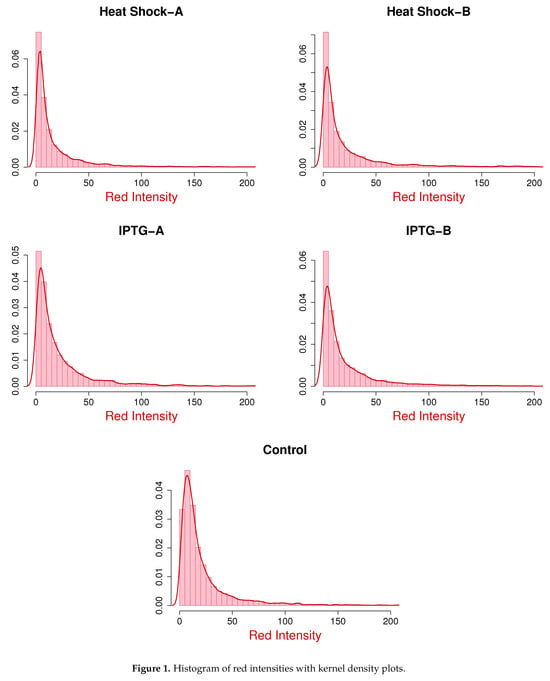
Figure 1
Open AccessArticle
Unveiling the Impact of Socioeconomic and Demographic Factors on Graduate Salaries: A Machine Learning Explanatory Analytical Approach Using Higher Education Statistical Agency Data
by
Bassey Henshaw, Bhupesh Kumar Mishra, William Sayers and Zeeshan Pervez
Analytics 2025, 4(1), 10; https://doi.org/10.3390/analytics4010010 - 11 Mar 2025
Abstract
►▼
Show Figures
Graduate salaries are a significant concern for graduates, employers, and policymakers, as various factors influence them. This study investigates determinants of graduate salaries in the UK, utilising survey data from HESA (Higher Education Statistical Agency) and integrating advanced machine learning (ML) explanatory techniques
[...] Read more.
Graduate salaries are a significant concern for graduates, employers, and policymakers, as various factors influence them. This study investigates determinants of graduate salaries in the UK, utilising survey data from HESA (Higher Education Statistical Agency) and integrating advanced machine learning (ML) explanatory techniques with statistical analytical methodologies. By employing multi-stage analyses alongside machine learning models such as decision trees, random forests and the explainability with SHAP stands for (Shapley Additive exPanations), this study investigates the influence of 21 socioeconomic and demographic variables on graduate salary outcomes. Key variables, including institutional reputation, age at graduation, socioeconomic classification, job qualification requirements, and domicile, emerged as critical determinants, with institutional reputation proving the most significant. Among ML methods, the decision tree achieved a standout with the highest accuracy through rigorous optimisation techniques, including oversampling and undersampling. SHAP highlighted the top 12 influential variables, providing actionable insights into the interplay between individual and systemic factors. Furthermore, the statistical analysis using ANOVA (Analysis of Variance) validated the significance of these variables, revealing intricate interactions that shape graduate salary dynamics. Additionally, domain experts’ opinions are also analysed to authenticate the findings. This research makes a unique contribution by combining qualitative contextual analysis with quantitative methodologies, machine learning explainability and domain experts’ views on addressing gaps in the existing identification of graduate salary predicting components. Additionally, the findings inform policy and educational interventions to reduce wage inequalities and promote equitable career opportunities. Despite limitations, such as the UK-specific dataset and the focus on socioeconomic and demographic variables, this study lays a robust foundation for future research in predictive modelling and graduate outcomes.
Full article
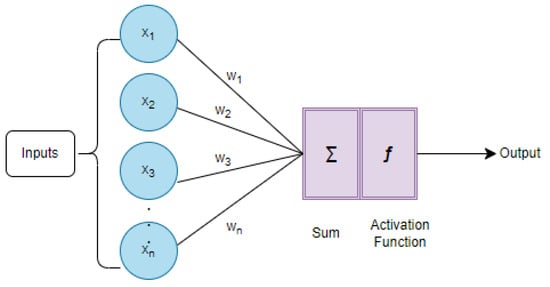
Figure 1
Open AccessEditorial
Updated Aims and Scope of Analytics
by
Carson K. Leung
Analytics 2025, 4(1), 9; https://doi.org/10.3390/analytics4010009 - 6 Mar 2025
Abstract
Analytics [...]
Full article
Open AccessArticle
The Role of Cognitive Performance in Older Europeans’ General Health: Insights from Relative Importance Analysis
by
Eleni Serafetinidou and Christina Parpoula
Analytics 2025, 4(1), 8; https://doi.org/10.3390/analytics4010008 - 4 Mar 2025
Abstract
This study explores the role of cognitive performance in the general health of older Europeans aged 50 and over, focusing on gender differences, using data from 336,500 respondents in the sixth wave of the Survey of Health, Aging, and Retirement in Europe (SHARE).
[...] Read more.
This study explores the role of cognitive performance in the general health of older Europeans aged 50 and over, focusing on gender differences, using data from 336,500 respondents in the sixth wave of the Survey of Health, Aging, and Retirement in Europe (SHARE). Cognitive functioning was assessed through self-rated reading and writing skills, orientation in time, numeracy, memory, verbal fluency, and word-list learning. General health status was estimated by constructing a composite index of physical and mental health-related measures, including chronic diseases, mobility limitations, depressive symptoms, self-perceived health, and the Global Activity Limitation Indicator. Participants were classified into good or poor health status, and logistic regression models assessed the predictive significance of cognitive variables on general health, supplemented by a relative importance analysis to estimate relative effect sizes. The results indicated that males had a 51.1% lower risk of reporting poor health than females, and older age was associated with a 4.0% increase in the odds of reporting worse health for both genders. Memory was the strongest predictor of health status (26% of the model ), with a greater relative contribution than the other cognitive variables. No significant gender differences were found. While this study estimates the odds of reporting poorer health in relation to gender and various cognitive characteristics, adopting a lifespan approach could provide valuable insights into the longitudinal associations between cognitive functioning and health outcomes.
Full article
Open AccessArticle
Towards Visual Analytics for Explainable AI in Industrial Applications
by
Kostiantyn Kucher, Elmira Zohrevandi and Carl A. L. Westin
Analytics 2025, 4(1), 7; https://doi.org/10.3390/analytics4010007 - 12 Feb 2025
Abstract
As the levels of automation and reliance on modern artificial intelligence (AI) approaches increase across multiple industries, the importance of the human-centered perspective becomes more evident. Various actors in such industrial applications, including equipment operators and decision makers, have their needs and preferences
[...] Read more.
As the levels of automation and reliance on modern artificial intelligence (AI) approaches increase across multiple industries, the importance of the human-centered perspective becomes more evident. Various actors in such industrial applications, including equipment operators and decision makers, have their needs and preferences that often do not align with the decisions produced by black-box models, potentially leading to mistrust and wasted productivity gain opportunities. In this paper, we examine these issues through the lenses of visual analytics and, more broadly, interactive visualization, and we argue that the methods and techniques from these fields can lead to advances in both academic research and industrial innovations concerning the explainability of AI models. To address the existing gap within and across the research and application fields, we propose a conceptual framework for visual analytics design and evaluation for such scenarios, followed by a preliminary roadmap and call to action for the respective communities.
Full article
(This article belongs to the Special Issue Visual Analytics: Techniques and Applications)
►▼
Show Figures
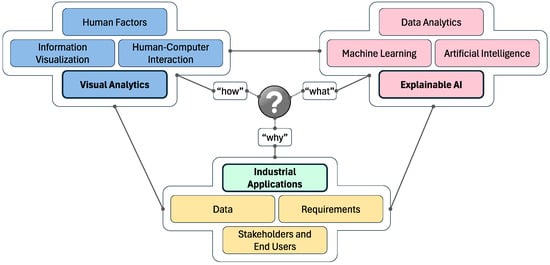
Figure 1
Open AccessArticle
Monetary Policy Sentiment and Its Influence on Healthcare and Technology Markets: A Transformer Model Approach
by
Dongnan Liu and Jong-Min Kim
Analytics 2025, 4(1), 6; https://doi.org/10.3390/analytics4010006 - 11 Feb 2025
Abstract
This study investigates how the Federal Open Market Committee’s (FOMC) statements impact healthcare spending, mental health trends, and stock performance in healthcare and tech sectors By analyzing FOMC’s sentiment from 2018 to 2024, we found that higher sentiment correlates with increased depressive disorders
[...] Read more.
This study investigates how the Federal Open Market Committee’s (FOMC) statements impact healthcare spending, mental health trends, and stock performance in healthcare and tech sectors By analyzing FOMC’s sentiment from 2018 to 2024, we found that higher sentiment correlates with increased depressive disorders (2019–2021) and tech stock returns, especially for the “Magnificent Seven” (like Apple and Amazon). Although healthcare stocks showed weaker ties to sentiment, Granger causality tests suggest some influence, hinting at ways to adjust stock strategies based on FOMC trends. These results highlight how central bank communication can shape both mental health dynamics and investment decisions in healthcare and technology.
Full article
(This article belongs to the Special Issue Business Analytics and Applications)
►▼
Show Figures
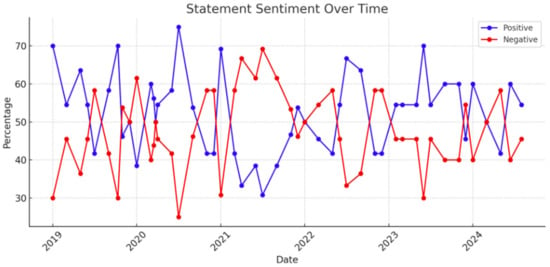
Figure 1
Open AccessArticle
A Comparative Analysis of Machine Learning and Deep Learning Techniques for Accurate Market Price Forecasting
by
Olamilekan Shobayo, Sidikat Adeyemi-Longe, Olusogo Popoola and Obinna Okoyeigbo
Analytics 2025, 4(1), 5; https://doi.org/10.3390/analytics4010005 - 11 Feb 2025
Cited by 2
Abstract
►▼
Show Figures
This study compares three machine learning and deep learning models—Support Vector Regression (SVR), Recurrent Neural Networks (RNN), and Long Short-Term Memory (LSTM)—for predicting market prices using the NGX All-Share Index dataset. The models were evaluated using multiple error metrics, including Mean Absolute Error
[...] Read more.
This study compares three machine learning and deep learning models—Support Vector Regression (SVR), Recurrent Neural Networks (RNN), and Long Short-Term Memory (LSTM)—for predicting market prices using the NGX All-Share Index dataset. The models were evaluated using multiple error metrics, including Mean Absolute Error (MAE), Mean Squared Error (MSE), Root Mean Square Error (RMSE), Mean Percentage Error (MPE), and R-squared. RNN and LSTM were tested with both 30 and 60-day windows, with performance compared to SVR. LSTM delivered better R-squared values, with a 60-day LSTM achieving the best accuracy (R-squared = 0.993) when using a combination of endogenous market data and technical indicators. SVR showed reliable results in certain scenarios but struggled in fold 2 with a sudden spike that shows a high probability of not capturing the entire underlying NGX pattern in the dataset correctly, as witnessed by the high validation loss during the period. Additionally, RNN faced the vanishing gradient problem that limits its long-term performance. Despite challenges, LSTM’s ability to handle temporal dependencies, especially with the inclusion of On-Balance Volume, led to significant improvements in prediction accuracy. The use of the Optuna optimisation framework further enhanced model training and hyperparameter tuning, contributing to the performance of the LSTM model.
Full article
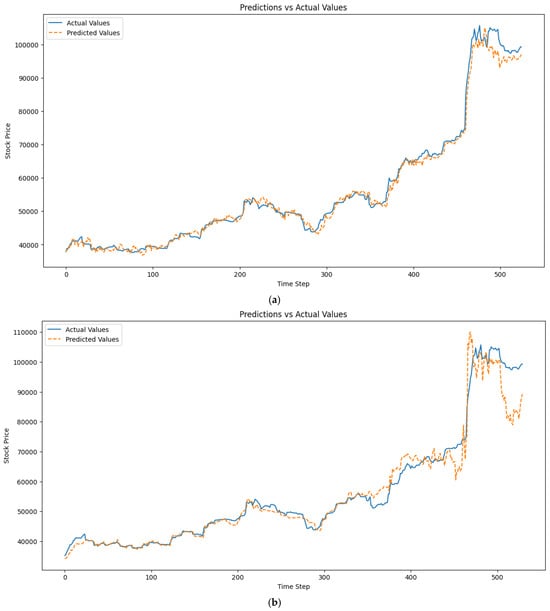
Figure 1
Open AccessArticle
Personalizing Multimedia Content Recommendations for Intelligent Vehicles Through Text–Image Embedding Approaches
by
Jin-A Choi, Taekeun Hong and Kiho Lim
Analytics 2025, 4(1), 4; https://doi.org/10.3390/analytics4010004 - 5 Feb 2025
Abstract
►▼
Show Figures
The ability to automate and personalize the recommendation of multimedia contents to consumers has been gaining significant attention recently. The burgeoning demand for digitization and automation of formerly analog communication processes has caught the attention of researchers and professionals alike. In light of
[...] Read more.
The ability to automate and personalize the recommendation of multimedia contents to consumers has been gaining significant attention recently. The burgeoning demand for digitization and automation of formerly analog communication processes has caught the attention of researchers and professionals alike. In light of the recent interest and anticipated transition to fully autonomous vehicles, this study proposes a text–image embedding method recommender system for the optimization of personalized multimedia content for in-vehicle infotainment. This study leverages existing pre-trained text embedding models and pre-trained image feature extraction methods. Previous research to date has focused mainly on textual-only or image-only analyses. By employing similarity measurements, this study demonstrates how recommendation of the most relevant multimedia content to consumers is enhanced through text–image embedding.
Full article
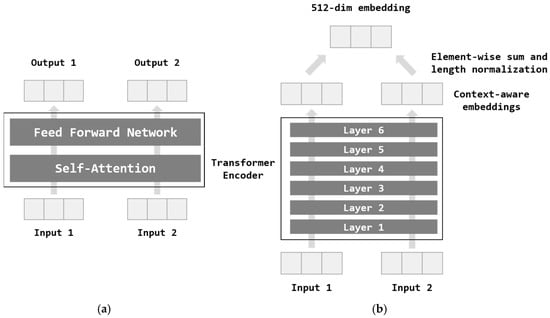
Figure 1
Open AccessArticle
A Fuzzy Analytical Network Process Framework for Prioritizing Competitive Intelligence in Startups
by
Arman Golshan, Soheila Sardar, Seyed Faraz Mahdavi Ardestani and Paria Sadeghian
Analytics 2025, 4(1), 3; https://doi.org/10.3390/analytics4010003 - 14 Jan 2025
Abstract
Competitive intelligence (CI) is a critical tool for startups, enabling informed decision making through the systematic gathering and analysis of relevant information. This study aims to identify and prioritize the key factors influencing CI in startups, providing actionable insights for entrepreneurs, educators, and
[...] Read more.
Competitive intelligence (CI) is a critical tool for startups, enabling informed decision making through the systematic gathering and analysis of relevant information. This study aims to identify and prioritize the key factors influencing CI in startups, providing actionable insights for entrepreneurs, educators, and support organizations. Through a systematic literature review, key variables and components impacting competitive intelligence were identified. Two surveys were conducted to refine these components. The first employed a five-point Likert scale to evaluate the significance of each component, while the second used a pairwise comparison approach involving ten experts in CI and startup mentorship. Utilizing the fuzzy Analytical Network Process (ANP), this study ranked Technology Intelligence as the most critical factor, followed by market and Strategic Intelligence. Competitor Intelligence and Internet intelligence were deemed moderately important, while Organizational Intelligence ranked lowest. These findings emphasize the importance of technology-driven insights and market awareness in fostering startups’ competitive advantage and informed decision making. This study provides a structured framework to guide startups in prioritizing CI efforts, offering practical strategies for navigating dynamic market conditions and achieving long-term success.
Full article
(This article belongs to the Special Issue Business Analytics and Applications)
Open AccessArticle
Use of Hazard Functions for Determining Power-Law Behaviour in Data
by
Joseph D. Bailey
Analytics 2025, 4(1), 2; https://doi.org/10.3390/analytics4010002 - 9 Jan 2025
Abstract
►▼
Show Figures
Determining the ‘best-fitting’ distribution for data is an important problem in data analysis. Specifically, observing how the distribution of data changes as values below (or above) a threshold are omitted from analyses can be of use in various applications, from animal movement to
[...] Read more.
Determining the ‘best-fitting’ distribution for data is an important problem in data analysis. Specifically, observing how the distribution of data changes as values below (or above) a threshold are omitted from analyses can be of use in various applications, from animal movement to the modelling of natural phenomena. Such truncated distributions, known as hazard functions, are widely studied and well understood in survival analysis, although rarely widely used in data analysis. Here, by considering the hazard and reverse-hazard functions, we demonstrate a qualitative assessment of the ‘best-fit’ distribution of data. Specifically, we highlight the potential advantages of this method when determining whether power-law behaviour may or may not be present in data. Finally, we demonstrate this approach using some real-world datasets.
Full article
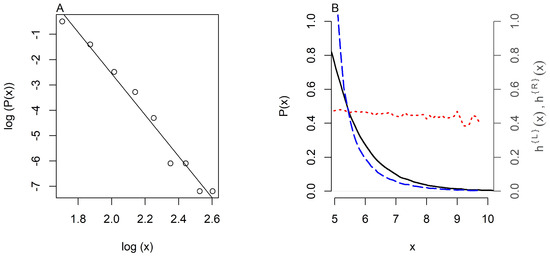
Figure 1
Open AccessArticle
Uncovering Patterns and Trends in Big Data-Driven Research Through Text Mining of NSF Award Synopses
by
Arielle King and Sayed A. Mostafa
Analytics 2025, 4(1), 1; https://doi.org/10.3390/analytics4010001 - 6 Jan 2025
Abstract
►▼
Show Figures
The rapid expansion of big data has transformed research practices across disciplines, yet disparities exist in its adoption among U.S. institutions of higher education. This study examines trends in NSF-funded big data-driven research across research domains, institutional classifications, and directorates. Using a quantitative
[...] Read more.
The rapid expansion of big data has transformed research practices across disciplines, yet disparities exist in its adoption among U.S. institutions of higher education. This study examines trends in NSF-funded big data-driven research across research domains, institutional classifications, and directorates. Using a quantitative approach and natural language processing (NLP) techniques, we analyzed NSF awards from 2006 to 2022, focusing on seven NSF research areas: Biological Sciences, Computer and Information Science and Engineering, Engineering, Geosciences, Mathematical and Physical Sciences, Social, Behavioral and Economic Sciences, and STEM Education (formally known as Education and Human Resources). Findings indicate a significant increase in big data-related awards over time, with CISE (Computer and Information Science and Engineering) leading in funding. Machine learning and artificial intelligence are dominant themes across all institutions’ classifications. Results show that R1 and non-minority-serving institutions receive the majority of big data-driven research funding, though HBCUs have seen recent growth due to national diversity initiatives. Topic modeling reveals key subdomains such as cybersecurity and bioinformatics benefiting from big data, while areas like Biological Sciences and Social Sciences engage less with these methods. These findings suggest the need for broader support and funding to foster equitable adoption of big data methods across institutions and disciplines.
Full article
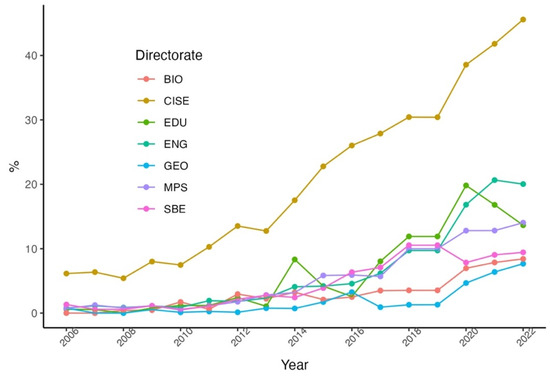
Figure 1
Open AccessReview
Advancements in Predictive Maintenance: A Bibliometric Review of Diagnostic Models Using Machine Learning Techniques
by
Nontuthuzelo Lindokuhle Vithi and Colin Chibaya
Analytics 2024, 3(4), 493-507; https://doi.org/10.3390/analytics3040028 - 10 Dec 2024
Cited by 3
Abstract
►▼
Show Figures
This bibliometric review investigates the advancements in machine learning techniques for predictive maintenance, focusing on the use of Artificial Neural Networks (ANNs) and Support Vector Machines (SVMs) for fault detection in wheelset axle bearings. Using data from Scopus and Web of Science, the
[...] Read more.
This bibliometric review investigates the advancements in machine learning techniques for predictive maintenance, focusing on the use of Artificial Neural Networks (ANNs) and Support Vector Machines (SVMs) for fault detection in wheelset axle bearings. Using data from Scopus and Web of Science, the review analyses key trends, influential publications, and significant contributions to the field from 2000 to 2024. The findings highlight the performance of ANNs in handling large datasets and modelling complex, non-linear relationships, as well as the high accuracy of SVMs in fault classification tasks, particularly with small-to-medium-sized datasets. However, the study also identifies several limitations, including the dependency on high-quality data, significant computational resource requirements, limited model adaptability, interpretability challenges, and practical implementation complexities. This review provides valuable insights for researchers and engineers, guiding the selection of appropriate diagnostic models and highlighting opportunities for future research. Addressing the identified limitations is crucial for the broader adoption and effectiveness of machine learning-based predictive maintenance strategies across various industrial contexts.
Full article
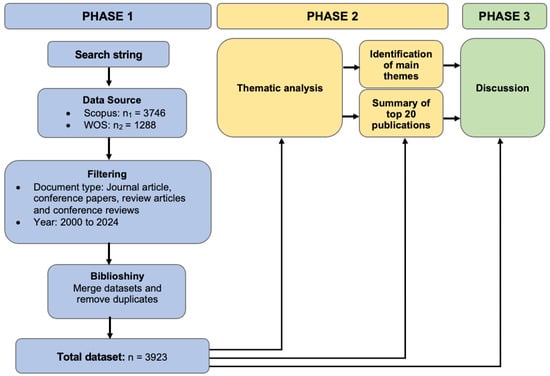
Figure 1
Open AccessArticle
NPI-WGNN: A Weighted Graph Neural Network Leveraging Centrality Measures and High-Order Common Neighbor Similarity for Accurate ncRNA–Protein Interaction Prediction
by
Fatemeh Khoushehgir, Zahra Noshad, Morteza Noshad and Sadegh Sulaimany
Analytics 2024, 3(4), 476-492; https://doi.org/10.3390/analytics3040027 - 2 Dec 2024
Cited by 1
Abstract
►▼
Show Figures
Predicting ncRNA–protein interactions (NPIs) is essential for understanding regulatory roles in cellular processes and disease mechanisms, yet experimental methods are costly and time-consuming. In this study, we propose NPI-WGNN, a novel weighted graph neural network model designed to enhance NPI prediction by incorporating
[...] Read more.
Predicting ncRNA–protein interactions (NPIs) is essential for understanding regulatory roles in cellular processes and disease mechanisms, yet experimental methods are costly and time-consuming. In this study, we propose NPI-WGNN, a novel weighted graph neural network model designed to enhance NPI prediction by incorporating topological insights from graph structures. Our approach introduces a bipartite version of the high-order common neighbor (HOCN) similarity metric to assign edge weights in an ncRNA–protein network, refining node embeddings via weighted node2vec. We further enrich these embeddings with centrality measures, such as degree and Katz centralities, to capture network hierarchy and connectivity. To optimize prediction accuracy, we employ a hybrid GNN architecture that combines graph convolutional network (GCN), graph attention network (GAT), and GraphSAGE layers, each contributing unique advantages: GraphSAGE offers scalability, GCN provides a global structural perspective, and GAT applies dynamic neighbor weighting. An ablation study confirms the complementary strengths of these layers, showing that their integration improves predictive accuracy and robustness across varied graph complexities. Experimental results on three benchmark datasets demonstrate that NPI-WGNN outperforms state-of-the-art methods, achieving up to 96.1% accuracy, 97.5% sensitivity, and an F1-score of 0.96, positioning it as a robust and accurate framework for ncRNA–protein interaction prediction.
Full article

Figure 1
Open AccessArticle
Breast Cancer Classification Using Fine-Tuned SWIN Transformer Model on Mammographic Images
by
Oluwatosin Tanimola, Olamilekan Shobayo, Olusogo Popoola and Obinna Okoyeigbo
Analytics 2024, 3(4), 461-475; https://doi.org/10.3390/analytics3040026 - 11 Nov 2024
Cited by 3
Abstract
►▼
Show Figures
Breast cancer is the most prevalent type of disease among women. It has become one of the foremost causes of death among women globally. Early detection plays a significant role in administering personalized treatment and improving patient outcomes. Mammography procedures are often used
[...] Read more.
Breast cancer is the most prevalent type of disease among women. It has become one of the foremost causes of death among women globally. Early detection plays a significant role in administering personalized treatment and improving patient outcomes. Mammography procedures are often used to detect early-stage cancer cells. This traditional method of mammography while valuable has limitations in its potential for false positives and negatives, patient discomfort, and radiation exposure. Therefore, there is a probe for more accurate techniques required in detecting breast cancer, leading to exploring the potential of machine learning in the classification of diagnostic images due to its efficiency and accuracy. This study conducted a comparative analysis of pre-trained CNNs (ResNet50 and VGG16) and vision transformers (ViT-base and SWIN transformer) with the inclusion of ViT-base trained from scratch model architectures to effectively classify mammographic breast cancer images into benign and malignant cases. The SWIN transformer exhibits superior performance with 99.9% accuracy and a precision of 99.8%. These findings demonstrate the efficiency of deep learning to accurately classify mammographic breast cancer images for the diagnosis of breast cancer, leading to improvements in patient outcomes.
Full article
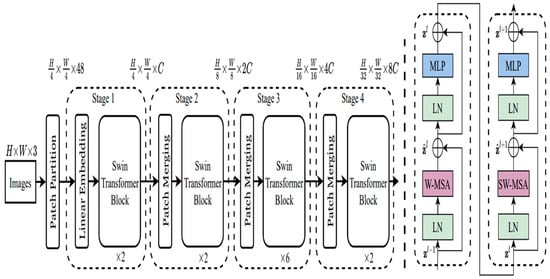
Figure 1
Highly Accessed Articles
Latest Books
E-Mail Alert
News
Topics

Conferences
Special Issues
Special Issue in
Analytics
Business Analytics and Applications
Guest Editors: Tatiana Ermakova, Benjamin FabianDeadline: 31 August 2025
Special Issue in
Analytics
Reviews on Data Analytics and Its Applications
Guest Editor: Carson K. LeungDeadline: 31 March 2026