New Insights into Digital Image Processing and Denoising
A special issue of Applied Sciences (ISSN 2076-3417). This special issue belongs to the section "Computing and Artificial Intelligence".
Deadline for manuscript submissions: closed (20 April 2025) | Viewed by 3987
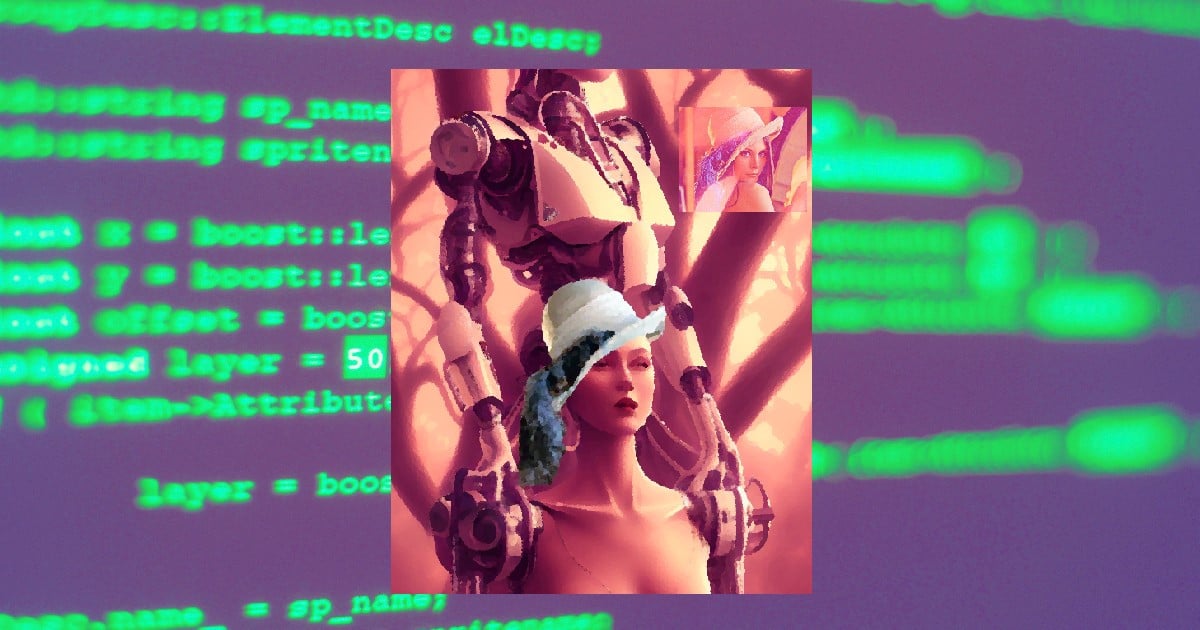
Special Issue Editors
Interests: visual content analysis; image features detection; image classification and recognition; image segmentation; image compression; contour extraction and approximation
Interests: digital communication; image and data processing; intelligent monitoring; security systems; information and coding theory; random signals; computer communications networks and signal processing; watermarking technology
Special Issue Information
Dear Colleagues,
Nowadays, in the era of cutting-edge technologies, the implementation of digital image processing techniques has become a key element of many solutions. This is because there are several potential innovative digital imaging applications that include different areas such as geographic information systems (GIS), environmental and traffic monitoring, quality control in production automation, medical diagnosis and treatment, autonomous vehicles, defense vision, robotics, remote sensing, art and cultural heritage preservation and exploitation, detecting crime and threats, 3D modeling and multimedia, etc.
The growing trends and requirements of the above-mentioned image applications are an increasing challenge for researchers and engineers dealing with digital image processing (DIP). Therefore, DIP is a multidisciplinary science that employs knowledge from various fields such as computer science, mathematics, and also visual psychophysics.
The aim of this Special Issue is to bring together high-quality scientific papers and reports referring to the latest and the most innovative research from a wide range of specialists that could provide comprehensive insights into modern approaches of digital image processing and denoising.
The topics of this Special Issue include (but are not limited to) the following:
- Advanced image (and video) enhancement, denoising, and restoration;
- Image segmentation and object extraction;
- Image classification and recognition;
- Image transforms and manipulations;
- Machine learning for image processing;
- Neural computing for image processing;
- Evolutionary algorithms for image processing;
- Object recognition and image tagging;
- Remote sensing image classification;
- Medical image classification;
- Face and person recognition;
- Handwriting recognition (HWR);
- Content-based image retrieval;
- Building (and other GIS objects) footprint extraction from LIDAR and photogrammetric data;
- 3D imaging, modeling, and visualization;
- Image augmentation and virtual reality;
- Watermarking techniques for data hiding and privacy protection;
- Video surveillance, object tracking, and threat detection;
- Video indexing and abstraction;
- Image quality assessment (IQA);
- Prediction of plant‒leaf disease.
Dr. Remigiusz Baran
Prof. Dr. Andrzej Dziech
Guest Editors
Manuscript Submission Information
Manuscripts should be submitted online at www.mdpi.com by registering and logging in to this website. Once you are registered, click here to go to the submission form. Manuscripts can be submitted until the deadline. All submissions that pass pre-check are peer-reviewed. Accepted papers will be published continuously in the journal (as soon as accepted) and will be listed together on the special issue website. Research articles, review articles as well as short communications are invited. For planned papers, a title and short abstract (about 100 words) can be sent to the Editorial Office for announcement on this website.
Submitted manuscripts should not have been published previously, nor be under consideration for publication elsewhere (except conference proceedings papers). All manuscripts are thoroughly refereed through a single-blind peer-review process. A guide for authors and other relevant information for submission of manuscripts is available on the Instructions for Authors page. Applied Sciences is an international peer-reviewed open access semimonthly journal published by MDPI.
Please visit the Instructions for Authors page before submitting a manuscript. The Article Processing Charge (APC) for publication in this open access journal is 2400 CHF (Swiss Francs). Submitted papers should be well formatted and use good English. Authors may use MDPI's English editing service prior to publication or during author revisions.
Keywords
- image processing
- image enhancement
- image recognition
- image augmentation
- image retrieval
- object instance segmentation
- shallow and deep learning
- CNN
- filter banks
- 3D imaging
Benefits of Publishing in a Special Issue
- Ease of navigation: Grouping papers by topic helps scholars navigate broad scope journals more efficiently.
- Greater discoverability: Special Issues support the reach and impact of scientific research. Articles in Special Issues are more discoverable and cited more frequently.
- Expansion of research network: Special Issues facilitate connections among authors, fostering scientific collaborations.
- External promotion: Articles in Special Issues are often promoted through the journal's social media, increasing their visibility.
- e-Book format: Special Issues with more than 10 articles can be published as dedicated e-books, ensuring wide and rapid dissemination.
Further information on MDPI's Special Issue policies can be found here.