Data-Driven Agricultural Innovation Technology for Digital Agriculture
A special issue of Applied Sciences (ISSN 2076-3417). This special issue belongs to the section "Agricultural Science and Technology".
Deadline for manuscript submissions: closed (20 July 2023) | Viewed by 13779
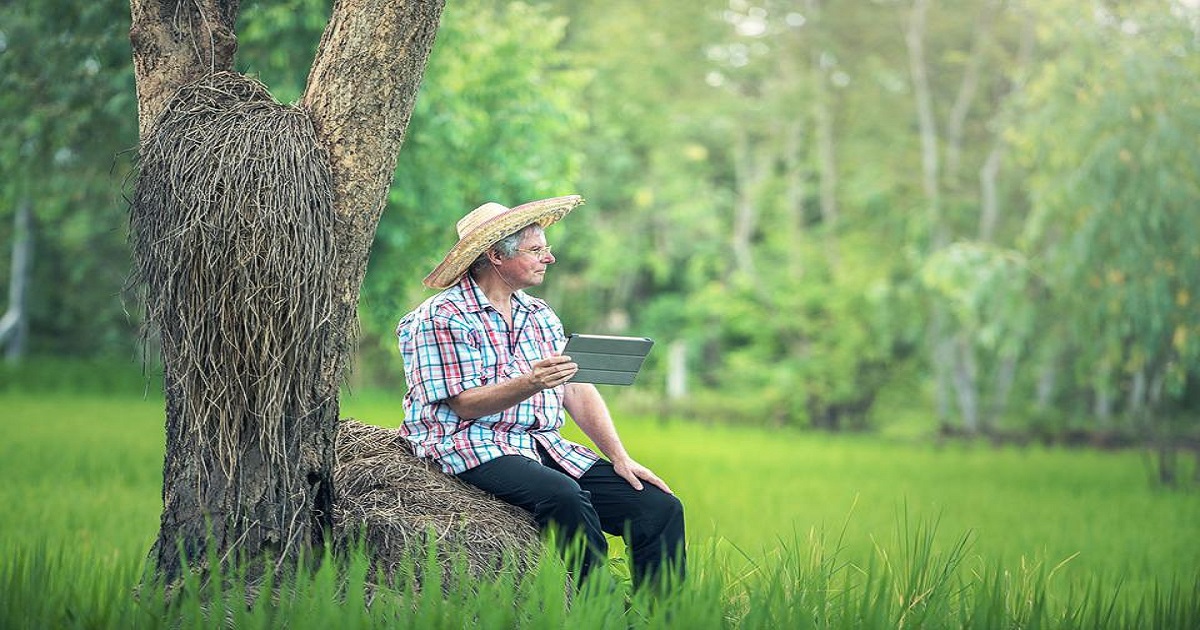
Special Issue Editor
Interests: digital agriculture; precision agriculture; soil characteristics; agricultural machinery load characteristics
Special Issue Information
Dear Colleagues,
Food security issues are emerging around the world and the need for improved agricultural productivity and high-convenience agriculture is increasing. Digital agriculture can be an effective solution to the above issues. In digital agriculture, data are the most valuable thing, and the effect of digital agriculture will be different depending on how well it is processed and reproduced. This is why we should pay attention to data-driven agricultural innovation technology. Even at this moment, various attempts are being made to implement digital agriculture by many researchers around the world. However, there is still an opening for new agricultural innovation technologies to be developed.
This Special Issue will focus on data-driven agricultural innovation technology for digital agriculture for the digitization of the data-driven entire agricultural cycle. We welcome research and reviews covering all relevant topics, including various agricultural innovation technologies such as sensing, control, data measurement, mapping, computational modelling, digital twins, artificial intelligence, intelligent systems, and solutions for digital agriculture.
Dr. Wan-Soo Kim
Guest Editor
Manuscript Submission Information
Manuscripts should be submitted online at www.mdpi.com by registering and logging in to this website. Once you are registered, click here to go to the submission form. Manuscripts can be submitted until the deadline. All submissions that pass pre-check are peer-reviewed. Accepted papers will be published continuously in the journal (as soon as accepted) and will be listed together on the special issue website. Research articles, review articles as well as short communications are invited. For planned papers, a title and short abstract (about 100 words) can be sent to the Editorial Office for announcement on this website.
Submitted manuscripts should not have been published previously, nor be under consideration for publication elsewhere (except conference proceedings papers). All manuscripts are thoroughly refereed through a single-blind peer-review process. A guide for authors and other relevant information for submission of manuscripts is available on the Instructions for Authors page. Applied Sciences is an international peer-reviewed open access semimonthly journal published by MDPI.
Please visit the Instructions for Authors page before submitting a manuscript. The Article Processing Charge (APC) for publication in this open access journal is 2400 CHF (Swiss Francs). Submitted papers should be well formatted and use good English. Authors may use MDPI's English editing service prior to publication or during author revisions.
Keywords
- digital agriculture
- data agriculture
- precision agriculture
- agricultural informatics
- digitalization
- computational modelling
- digital twin
- artificial intelligence
- intelligent systems