Human-Computer Interaction and Advanced Driver-Assistance Systems
A special issue of Applied Sciences (ISSN 2076-3417). This special issue belongs to the section "Transportation and Future Mobility".
Deadline for manuscript submissions: closed (20 September 2022) | Viewed by 7603
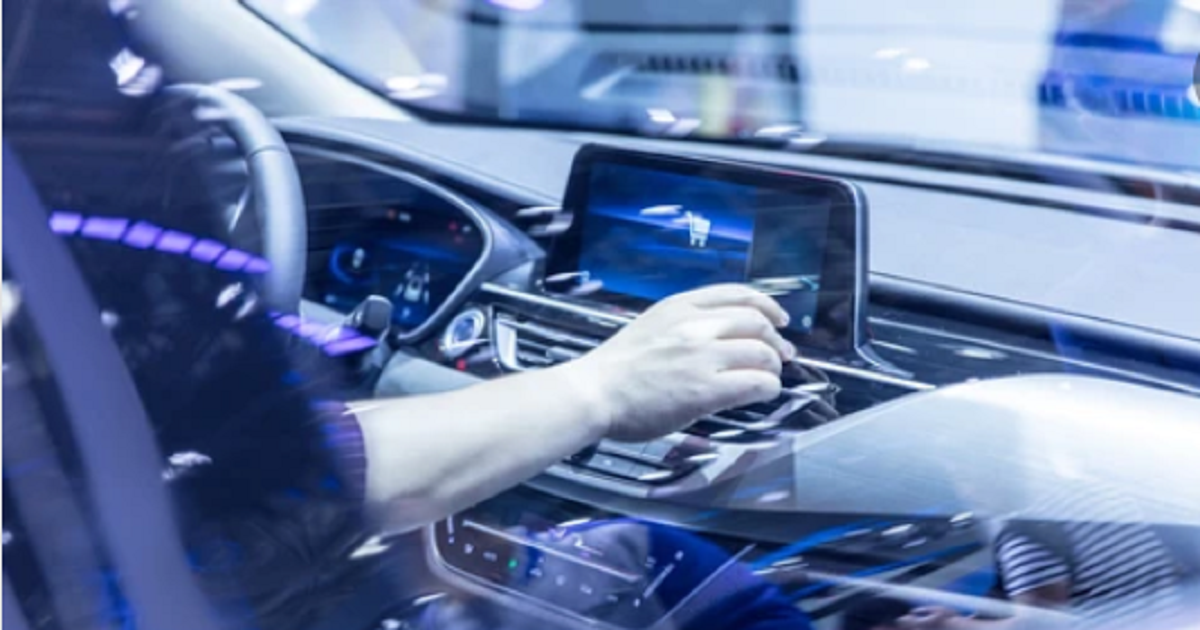
Special Issue Editor
Interests: intelligent vehicle; human machine interaction; advanced driver assistance systems
Special Issues, Collections and Topics in MDPI journals
Special Issue Information
Dear Colleagues,
The Sixth Asian Conference on Artificial Intelligence Technology will be held in Changzhou, China. The ACAIT 2022 conference invites the submission of substantial, original, and unpublished research papers regarding artificial intelligence (AI) applications in environment perception, intelligent vehicles, advanced driver-assistance systems, driver behavior analysis, and other recent advancements in and future trends of AI applications.
This Special Issue is dedicated to the application of human–machine interaction and advanced driver assistance systems in intelligent vehicles. In recent years, to reduce traffic accidents caused by human problems, advanced driving assistance technologies have been widely used to enhance the intelligence of vehicles. By reminding the driver or manipulating the car through actuators, advanced driving assistance technology greatly improves driving safety and comfort as well as frees the driver from heavy driving tasks.
However, during assisted driving, the driver may interfere with the assisted driving system and cause human–machine conflict. In some cases, due to system limitations, the driver may even need to regain control of the vehicle. Therefore, it is necessary to study human–machine interaction and co-driving technology.
For better design human–machine co-driving technology and advanced driving assistance systems, we need to comprehensively consider issues such as perception, control algorithms, human–machine interaction, human factors, and driving authority distribution, so as to reduce human–machine conflicts and improve vehicle safety and comfort.
This Special Issue includes but is not limited to the following topics:
- Vehicle states perception technologies;
- Intelligent decision technologies;
- Environment identification technologies;
- Chassis cooperative control technologies;
- Multisource information fusion technologies;
- Human factor;
- Human-machine interaction;
- Human-machine co-driving;
- Driving state detection and intention identification;
- Driver modeling.
Dr. Lie Guo
Guest Editor
Manuscript Submission Information
Manuscripts should be submitted online at www.mdpi.com by registering and logging in to this website. Once you are registered, click here to go to the submission form. Manuscripts can be submitted until the deadline. All submissions that pass pre-check are peer-reviewed. Accepted papers will be published continuously in the journal (as soon as accepted) and will be listed together on the special issue website. Research articles, review articles as well as short communications are invited. For planned papers, a title and short abstract (about 100 words) can be sent to the Editorial Office for announcement on this website.
Submitted manuscripts should not have been published previously, nor be under consideration for publication elsewhere (except conference proceedings papers). All manuscripts are thoroughly refereed through a single-blind peer-review process. A guide for authors and other relevant information for submission of manuscripts is available on the Instructions for Authors page. Applied Sciences is an international peer-reviewed open access semimonthly journal published by MDPI.
Please visit the Instructions for Authors page before submitting a manuscript. The Article Processing Charge (APC) for publication in this open access journal is 2400 CHF (Swiss Francs). Submitted papers should be well formatted and use good English. Authors may use MDPI's English editing service prior to publication or during author revisions.
Keywords
- vehicle states perception technologies
- intelligent decision technologies
- environment identification technologies
- chassis cooperative control technologies
- multi-source information fusion technologies
- human factor
- human-machine interaction
- human-machine co-driving
- driving state detection and intention identification
- driver modeling
Benefits of Publishing in a Special Issue
- Ease of navigation: Grouping papers by topic helps scholars navigate broad scope journals more efficiently.
- Greater discoverability: Special Issues support the reach and impact of scientific research. Articles in Special Issues are more discoverable and cited more frequently.
- Expansion of research network: Special Issues facilitate connections among authors, fostering scientific collaborations.
- External promotion: Articles in Special Issues are often promoted through the journal's social media, increasing their visibility.
- e-Book format: Special Issues with more than 10 articles can be published as dedicated e-books, ensuring wide and rapid dissemination.
Further information on MDPI's Special Issue polices can be found here.