Approaches to Machine and Deep Learning, Big Data or Modern Analytical Methods in the Agri-Food Industry
A special issue of Applied Sciences (ISSN 2076-3417). This special issue belongs to the section "Food Science and Technology".
Deadline for manuscript submissions: closed (20 February 2024) | Viewed by 5776
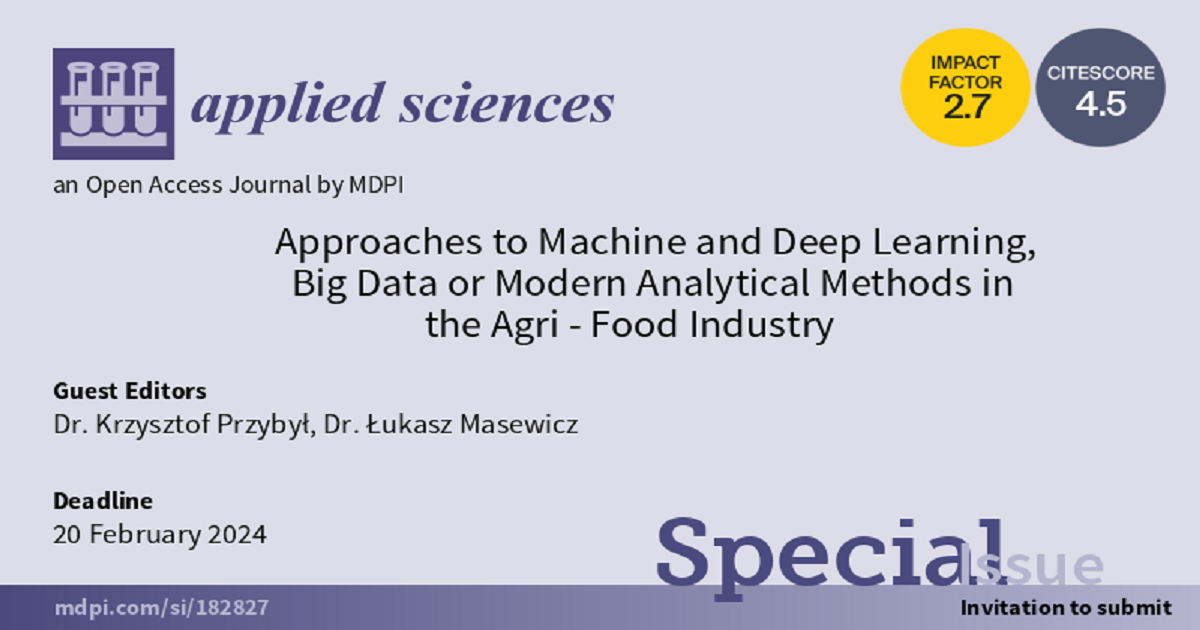
Special Issue Editors
Interests: application of artificial intelligence; deep learning and machine learning; high-dimensional data visualization; python programming; database designing; data preprocessing; statistical analysis; optimizing fruit and vegetable drying processes; patterns analyzing; analysis of the morphological structure of raw materials using electron microscopy
Special Issues, Collections and Topics in MDPI journals
Interests: food safety; water diffusion in food systems; physical properties of food; modified starch and its applications; water activity measurement; low-field nuclear magnetic resonance (LF-NMR); FTIR spectroscopy; colour measurement
Special Issue Information
Dear Colleague,
Changing consumer habits, food supply and economic aspirations require the optimization of processes and the application of modern technologies aimed at food preservation and achieving a high-quality index of finished foodstuffs. High levels of food waste are causing food producers to find ways in which to extend the shelf life of food items. Efforts are being made to reduce industrial energy intensity in the food production process via automation, optimization and the application of artificial intelligence to individual processes. Novel means of managing production, production organization, processing and waste management are also being applied using artificial intelligence. This article will take a deeper look at key aspects related to data analysis and technological applications in the agri-food industry. In this edition, topics of interest include, but are not limited to, the following:
- Classification and prediction models, machine and deep learning.
- Analytical methods, including spectroscopy, chromatography, image analysis and computer vision, electron microscopy and microbiological methods.
- Quality control in food production and distribution.
- Precision agriculture, yield forecasting, process optimization.
- New product development and innovation.
- Data collection and management.
In food production, quality control at every stage of the process, from the acquisition of raw materials to the receipt of the final product after processing, is an essential aspect. In view of this, it is reasonable to employ modern tools such as classification and prediction models, based on machine learning or deep learning. These advanced techniques make it possible to analyze vast quantities of data, identify patterns and predict quality, which translates into a quick yet effective response to potential quality problems.
In agriculture, yield forecasting, optimizing cultivation processes and monitoring environmental parameters are becoming key aspects of effective farm management. By employing technologies such as remote sensing, field sensors and geospatial data analysis, farmers can make better-informed decisions, resulting in enhanced productivity and minimized environmental impact.
In innovation and new product development, data analysis is influencing the outcome. Tracking consumer trends, analyzing preferences and identifying market niches make it possible to create products that better meet consumer needs. Image analysis and computer vision technologies enable the automatic sorting and quality assessment of products, accelerating production processes.
All these aspects require effective data collection, management and analysis, which apply to both the food and agricultural sectors. By employing advanced analytical methods and machine learning technologies, the agri-food industry can achieve higher standards of quality, efficiency and innovation, while minimizing its environmental impact.
Dr. Krzysztof Przybył
Dr. Łukasz Masewicz
Guest Editors
Manuscript Submission Information
Manuscripts should be submitted online at www.mdpi.com by registering and logging in to this website. Once you are registered, click here to go to the submission form. Manuscripts can be submitted until the deadline. All submissions that pass pre-check are peer-reviewed. Accepted papers will be published continuously in the journal (as soon as accepted) and will be listed together on the special issue website. Research articles, review articles as well as short communications are invited. For planned papers, a title and short abstract (about 100 words) can be sent to the Editorial Office for announcement on this website.
Submitted manuscripts should not have been published previously, nor be under consideration for publication elsewhere (except conference proceedings papers). All manuscripts are thoroughly refereed through a single-blind peer-review process. A guide for authors and other relevant information for submission of manuscripts is available on the Instructions for Authors page. Applied Sciences is an international peer-reviewed open access semimonthly journal published by MDPI.
Please visit the Instructions for Authors page before submitting a manuscript. The Article Processing Charge (APC) for publication in this open access journal is 2400 CHF (Swiss Francs). Submitted papers should be well formatted and use good English. Authors may use MDPI's English editing service prior to publication or during author revisions.