MRI in Prostate Cancer
A special issue of Cancers (ISSN 2072-6694). This special issue belongs to the section "Cancer Causes, Screening and Diagnosis".
Deadline for manuscript submissions: 20 July 2024 | Viewed by 5432
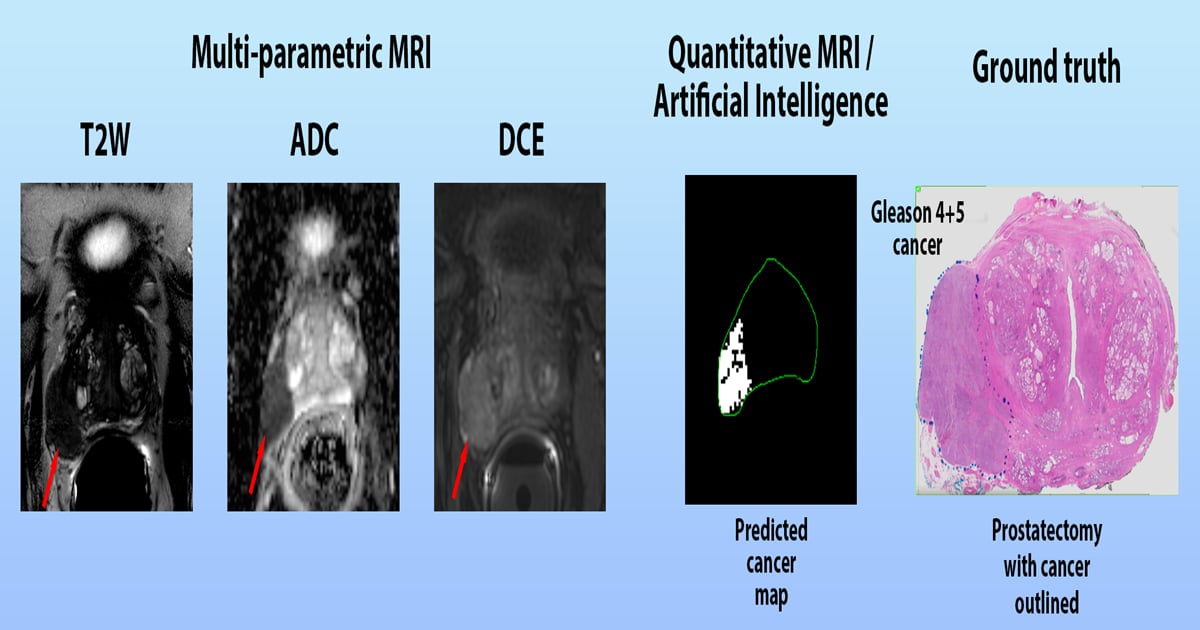
Special Issue Editors
Interests: quantitative MRI methods; diffusion weighted imaging (DWI); dynamic contrast-enhanced MRI (DCE-MRI); breast; prostate; urogenital; cancer imaging; body MRI; MRI physics
Special Issue Information
Dear Colleagues,
Prostate cancer is one of the most common types of cancer in men, representing a major public health concern. It is estimated that one in nine men will be diagnosed with prostate cancer in their lifetime. Magnetic resonance imaging (MRI) is a powerful imaging technique that has been used to diagnose and monitor prostate cancer. It uses a combination of a strong magnetic field and radio waves to create detailed images of the prostate. MRI can detect the size, shape, and location of a tumor, as well as its relationship to other organs and structures. It can also be used to monitor the progression of the disease and assess the effectiveness of treatment.
This Special Issue focuses on the use of MRI in the diagnosis and management of prostate cancer. It covers topics such as the use of MRI for prostate cancer screening, the role of MRI in the staging and treatment of prostate cancer, and the use of MRI to monitor the response to treatment. This Special Issue also includes reviews of the latest research and clinical applications of MRI in prostate cancer. It provides an invaluable resource for researchers, clinicians, and patients interested in the use of MRI in the diagnosis and management of prostate cancer.
Dr. Milica Medved
Dr. Aritrick Chatterjee
Guest Editors
Manuscript Submission Information
Manuscripts should be submitted online at www.mdpi.com by registering and logging in to this website. Once you are registered, click here to go to the submission form. Manuscripts can be submitted until the deadline. All submissions that pass pre-check are peer-reviewed. Accepted papers will be published continuously in the journal (as soon as accepted) and will be listed together on the special issue website. Research articles, review articles as well as short communications are invited. For planned papers, a title and short abstract (about 100 words) can be sent to the Editorial Office for announcement on this website.
Submitted manuscripts should not have been published previously, nor be under consideration for publication elsewhere (except conference proceedings papers). All manuscripts are thoroughly refereed through a single-blind peer-review process. A guide for authors and other relevant information for submission of manuscripts is available on the Instructions for Authors page. Cancers is an international peer-reviewed open access semimonthly journal published by MDPI.
Please visit the Instructions for Authors page before submitting a manuscript. The Article Processing Charge (APC) for publication in this open access journal is 2900 CHF (Swiss Francs). Submitted papers should be well formatted and use good English. Authors may use MDPI's English editing service prior to publication or during author revisions.
Keywords
- MRI
- prostate cancer
- screening
- diagnosis
- treatment