Analysis, Control and Modeling of Big Data for COVID-19 and Other Pandemics
A special issue of Processes (ISSN 2227-9717). This special issue belongs to the section "Biological Processes and Systems".
Deadline for manuscript submissions: closed (15 December 2023) | Viewed by 4874
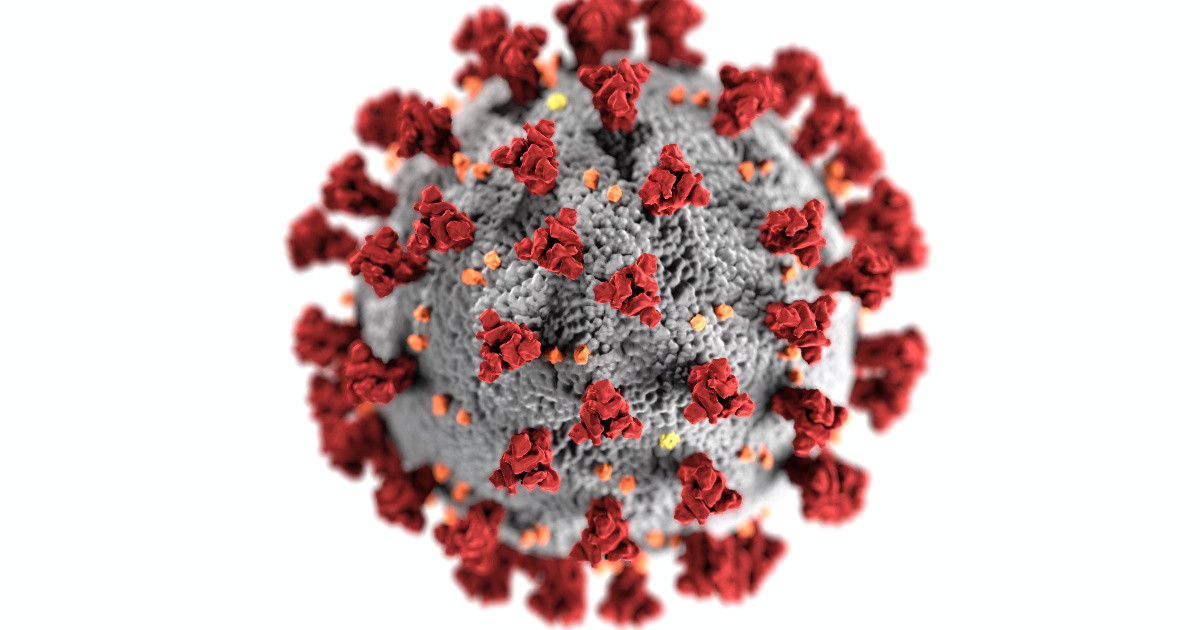
Special Issue Editor
Special Issue Information
Dear Colleagues,
Epidemic diseases have negatively impacted human life and health. For example, the COVID-19 pandemic has causes millions of deaths in the last two years. It is important to learn from the past or ongoing epidemics to develop preventive strategies or treatment approaches for future potential epidemics. Fortunately, historical data for certain epidemics have been recorded via surveillance systems. For example, the experimental Household Pulse Survey was designed by the Census Bureau in the United States to quickly and efficiently deploy data collected on how people’s lives have been impacted by the coronavirus pandemic. In this Special Issue “Analysis, Control and Modeling of Big Data for COVID-19 and Other Pandemics”, we solicit contributions that present the analysis or modeling of historical data for most recent epidemics which advance our understanding of epidemics in the following areas, including, but not limited to, epidemic modeling and prediction, analysis of surveillance data, epidemic evolution, spatial-temporal patterns of epidemic, and visualization of epidemic data. Applications of advanced machine learning techniques to big data for epidemics are especially encouraged. Example topics include:
- Develop models from historical outbreak data to predict the trend of an epidemic;
- Analyze surveillance data for an epidemic to identify effective approaches in responding to an epidemic;
- Identify the evolution trend of an epidemic from the mutation in virus DNA sequences;
- Study the spatial-temporal pattern of epidemic cases;
- Visualize the large amount of spatial-temporal epidemic data.
Dr. Zuyi (Jacky) Huang
Guest Editor
Manuscript Submission Information
Manuscripts should be submitted online at www.mdpi.com by registering and logging in to this website. Once you are registered, click here to go to the submission form. Manuscripts can be submitted until the deadline. All submissions that pass pre-check are peer-reviewed. Accepted papers will be published continuously in the journal (as soon as accepted) and will be listed together on the special issue website. Research articles, review articles as well as short communications are invited. For planned papers, a title and short abstract (about 100 words) can be sent to the Editorial Office for announcement on this website.
Submitted manuscripts should not have been published previously, nor be under consideration for publication elsewhere (except conference proceedings papers). All manuscripts are thoroughly refereed through a single-blind peer-review process. A guide for authors and other relevant information for submission of manuscripts is available on the Instructions for Authors page. Processes is an international peer-reviewed open access monthly journal published by MDPI.
Please visit the Instructions for Authors page before submitting a manuscript. The Article Processing Charge (APC) for publication in this open access journal is 2400 CHF (Swiss Francs). Submitted papers should be well formatted and use good English. Authors may use MDPI's English editing service prior to publication or during author revisions.
Keywords
- machine learning
- mathematical modeling
- data analysis
- epidemic
- surveillance data
Benefits of Publishing in a Special Issue
- Ease of navigation: Grouping papers by topic helps scholars navigate broad scope journals more efficiently.
- Greater discoverability: Special Issues support the reach and impact of scientific research. Articles in Special Issues are more discoverable and cited more frequently.
- Expansion of research network: Special Issues facilitate connections among authors, fostering scientific collaborations.
- External promotion: Articles in Special Issues are often promoted through the journal's social media, increasing their visibility.
- e-Book format: Special Issues with more than 10 articles can be published as dedicated e-books, ensuring wide and rapid dissemination.
Further information on MDPI's Special Issue polices can be found here.